Multi-source sintering transfer learning in small dataset sintering prediction scenario
INTERNATIONAL JOURNAL OF MATERIAL FORMING(2021)
摘要
The purpose of this paper is to achieve fast densification prediction of target materials and particularly of difficult-to-sintering materials in small dataset sintering scenarios. A multi-source sintering transfer learning framework based on the domain adversarial network (DANN) was proposed to achieve multi-source sintering transfer learning. Further, a calibration method was presented to enhance the reliability of the integrated multi-source DANN (IMDANN), where DANN was applied as a test benchmark. The results indicate that IMDANN is significantly better than the test benchmark under all the test conditions. Error analysis illustrates that the root mean square error (RMSE) of IMDANN’s prediction converges to approximately 5% in the target domain, and the average prediction error is reduced by 56.5%. With an increasing number of source domains following the correlation criterion, the minimum number of source domains required for convergence is only 3–4. Compared to DANN, the calibrated IMDANN has high reliability and realises the cross-domain transfer of sintering knowledge with a small dataset.
更多查看译文
关键词
Spark plasma sintering,Master sintering curve,Multi-source transfer learning,Deep learning,Small dataset
AI 理解论文
溯源树
样例
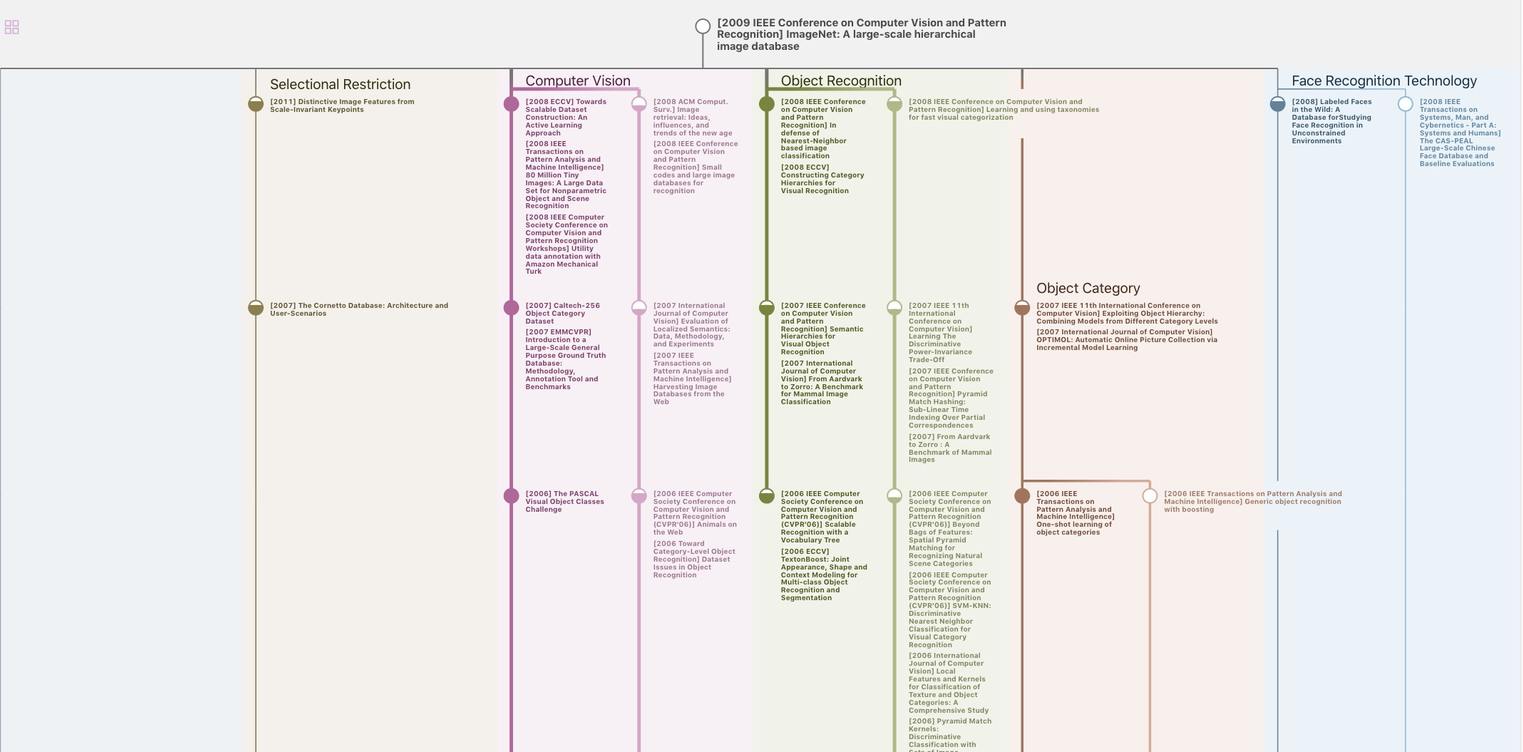
生成溯源树,研究论文发展脉络
Chat Paper
正在生成论文摘要