A comparative assessment of modeling groundwater vulnerability using DRASTIC method from GIS and a novel classification method using machine learning classifiers
GEOCARTO INTERNATIONAL(2022)
Abstract
Groundwater is more prone to contamination due to its extensive usage. Different methods are applied to study vulnerability of groundwater including widely used DRASTIC method, SI and GOD. This study proposes a novel method of mapping groundwater vulnerability using machine learning algorithms. In this study, point extraction method was used to extract point values from a grid of 646 points of seven raster layer in the Al Khatim study area of United Arab Emirates. These extracted values were classified based on nitrate concentration threshold of 50 mg/L into two classes. Machine learning models were developed, using depth to water (D), recharge (R), aquifer media (A), soil media (S), topography (T), vadose zone (I) and hydraulic conductivity (C), on the basis of nitrate class. Classified 'groundwater vulnerability class values' were trained using 10-fold cross-validation, using four machine learning models which were Random Forest, Support Vector Machine, Naive Bayes and C4. 5. Accuracy showed the model developed by Random Forest gained highest accuracy of 93%. Four groundwater vulnerability maps were developed from machine learning classifiers and was compared with base method of DRASTIC index. The efficiency, accuracy and validity of machine learning based models were evaluated based on Receiver Operating Characteristics (ROC) curve and Precision-Recall curve (PRC). The results proved that machine learning is an efficient tool to access, analyze and map groundwater vulnerability.
MoreTranslated text
Key words
Machine learning, nitrate, DRASTIC index, groundwater, ArcGIS, Random Forest, Support Vector Machine, Naive Bayes, C4. 5
AI Read Science
Must-Reading Tree
Example
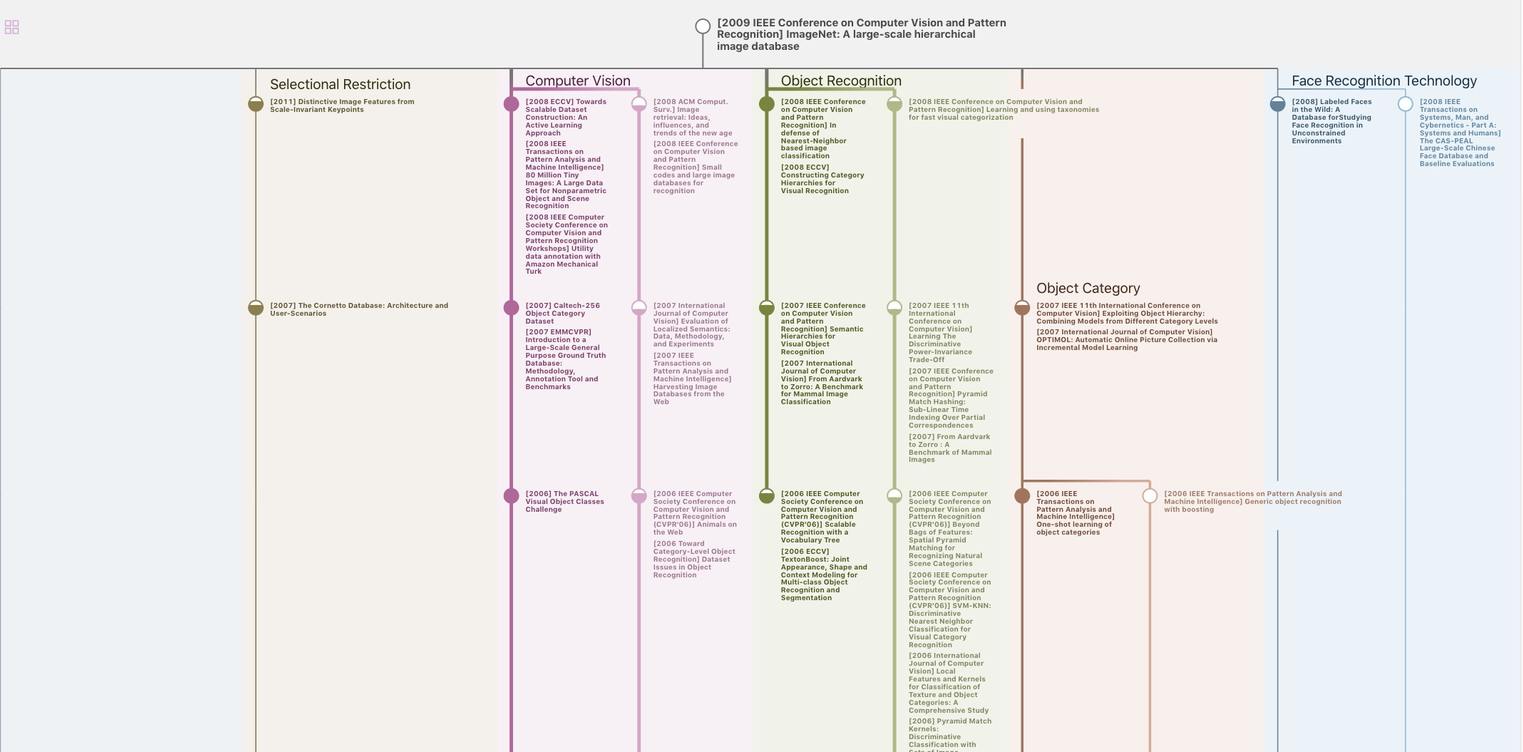
Generate MRT to find the research sequence of this paper
Chat Paper
Summary is being generated by the instructions you defined