Lithological Mapping Using Eo-1 Hyperion Hyperspectral Data And Semisupervised Self-Learning Method
JOURNAL OF APPLIED REMOTE SENSING(2021)
Abstract
Hyperspectral remote sensing data have been widely used in lithological identification and mapping. In existing studies, sufficient training samples are required. However, collecting sufficient labeled training samples for lithological classification in remote and inaccessible areas is generally time consuming, expensive, and even hard to implement, which causes the insufficient training sample problems in lithological classification using hyperspectral data. The semisupervised self-learning (SSL) method provides an alternative way of addressing this ill-posed problem by enlarging the training set from unlabeled samples using the limited labeled samples as a priori. This study evaluates and analyzes the SSL method in lithological mapping using EO-1 Hyperion hyperspectral image over a remote area. The performance of SSL with limited training samples was validated by comparing with multinomial logistic regression (MLR) and random forest (RF) classification using full training samples. The experimental results indicate that SSL method with limited training samples can produce results comparable with the MLR with all the training samples and better results than those of the RF with all the training samples. Therefore, the SSL method provides a useful way for hyperspectral lithological mapping with limited training samples over remote and inaccessible areas. (C) 2021 Society of Photo-Optical Instrumentation Engineers (SPIE)
MoreTranslated text
Key words
hyperspectral data, semisupervised self-learning, multinomial logistic regression, lithological mapping
AI Read Science
Must-Reading Tree
Example
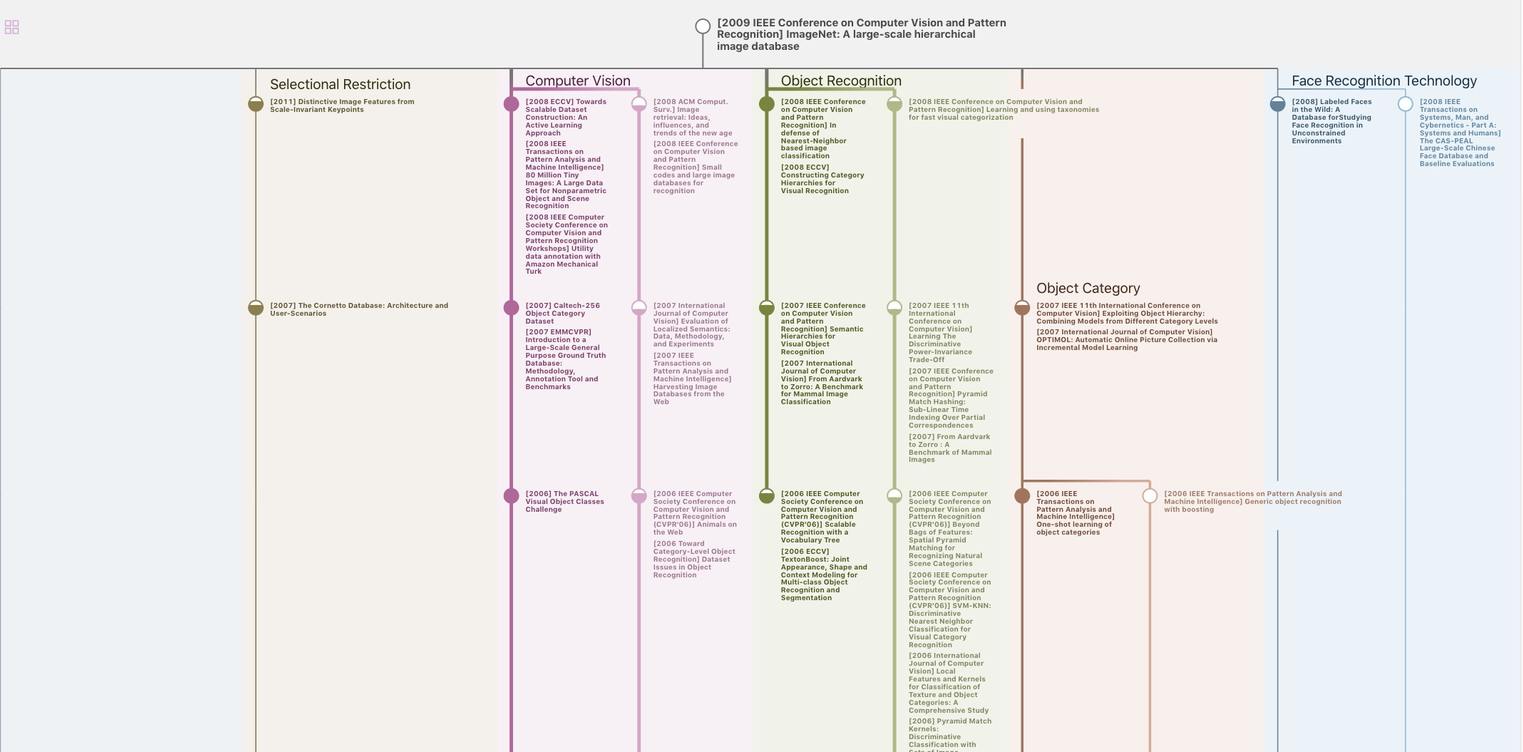
Generate MRT to find the research sequence of this paper
Chat Paper
Summary is being generated by the instructions you defined