CDFFNet: cross dimensional feature fusion network for urban land use detection
JOURNAL OF APPLIED REMOTE SENSING(2021)
摘要
The detection of urban land use is of great significance to urban planning. With the development of deep learning technology, convolutional neural networks (CNN) are widely used in remote sensing image processing. However, existing CNN models lack the ability to fuse high-dimensional semantic information and low-dimensional position information of images and thus perform poorly in feature restoration. To obtain better urban land use detection results, the cross-dimensional feature fusion network (CDFFNet) is proposed in this work. In the CDFFNet, Class Feature Attention (CFA), cross-level feature fusion (CLEF), and Same Level Feature Gate Fusion (SLFGF) are designed. The CFA aggregates multi-scale and multi-receptive field input features to capture high-dimensional semantic information. The CLEF is designed to fuse features of different resolutions. It can extract and fuse semantic information of different dimensions, aggregate contextual information of features and distinguish the contextual relevance of each pixel. The SLFGF dynamically fuses high-dimensional semantic information and low-dimensional spatial information, so it could enhance information recovery of different categories. Compared with existing methods, the CDFFNet is significantly improved in accuracy. Its mean intersection over union on the Aerial Image Segmentation Dataset reaches 0.765. (C) 2021 Society of Photo-Optical Instrumentation Engineers (SPIE)
更多查看译文
关键词
urban land use,deep learning,convolutional neural networks,feature restoration
AI 理解论文
溯源树
样例
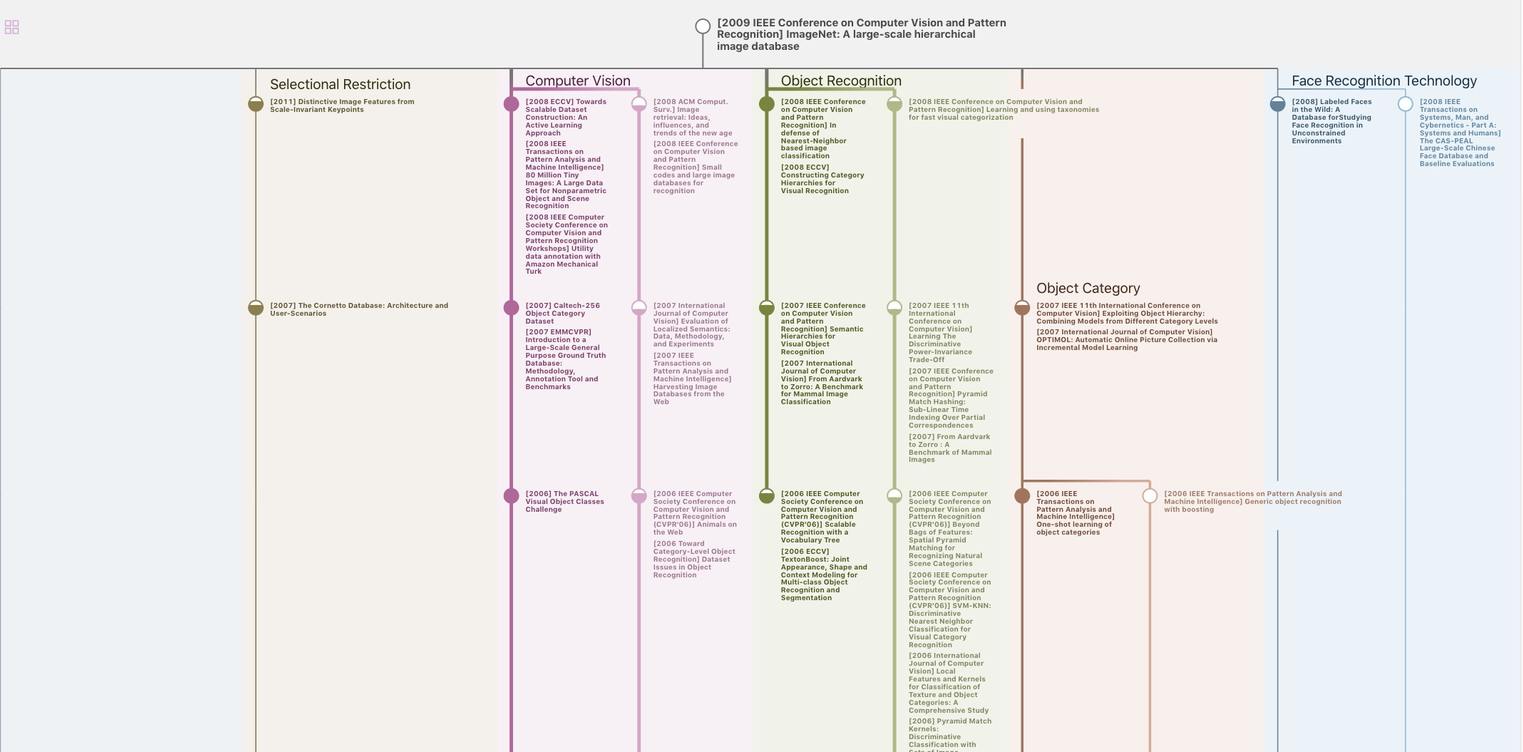
生成溯源树,研究论文发展脉络
Chat Paper
正在生成论文摘要