Value Function Guided Subgroup Identification via Gradient Tree Boosting: A Framework to Handle Multiple Outcomes for Optimal Treatment Recommendation
STATISTICS IN BIOPHARMACEUTICAL RESEARCH(2022)
Abstract
In randomized clinical trials, there has been an increasing interest in identifying subgroups with heterogeneous responses to study treatment based on baseline patient characteristics. Even though the benefit risk assessment of any patient population or subgroups is almost always a multi-facet consideration, the statistical literature of subgroup identification has largely been limited to a single clinical outcome. In the article, we propose a nonparametric method that searches for subgroup membership scores by maximizing a value function that directly reflects the subgroup-treatment interaction effect considering clinical priorities of multiple outcomes based on win difference. A gradient tree boosting algorithm is proposed to search for the individual subgroup membership scores. We conduct simulation studies to evaluate the performance of the proposed method and an application to a colon cancer adjuvant clinical trial is performed for illustration.
MoreTranslated text
Key words
Gradient tree boosting,Nonparametric,Subgroup identification,Win difference
AI Read Science
Must-Reading Tree
Example
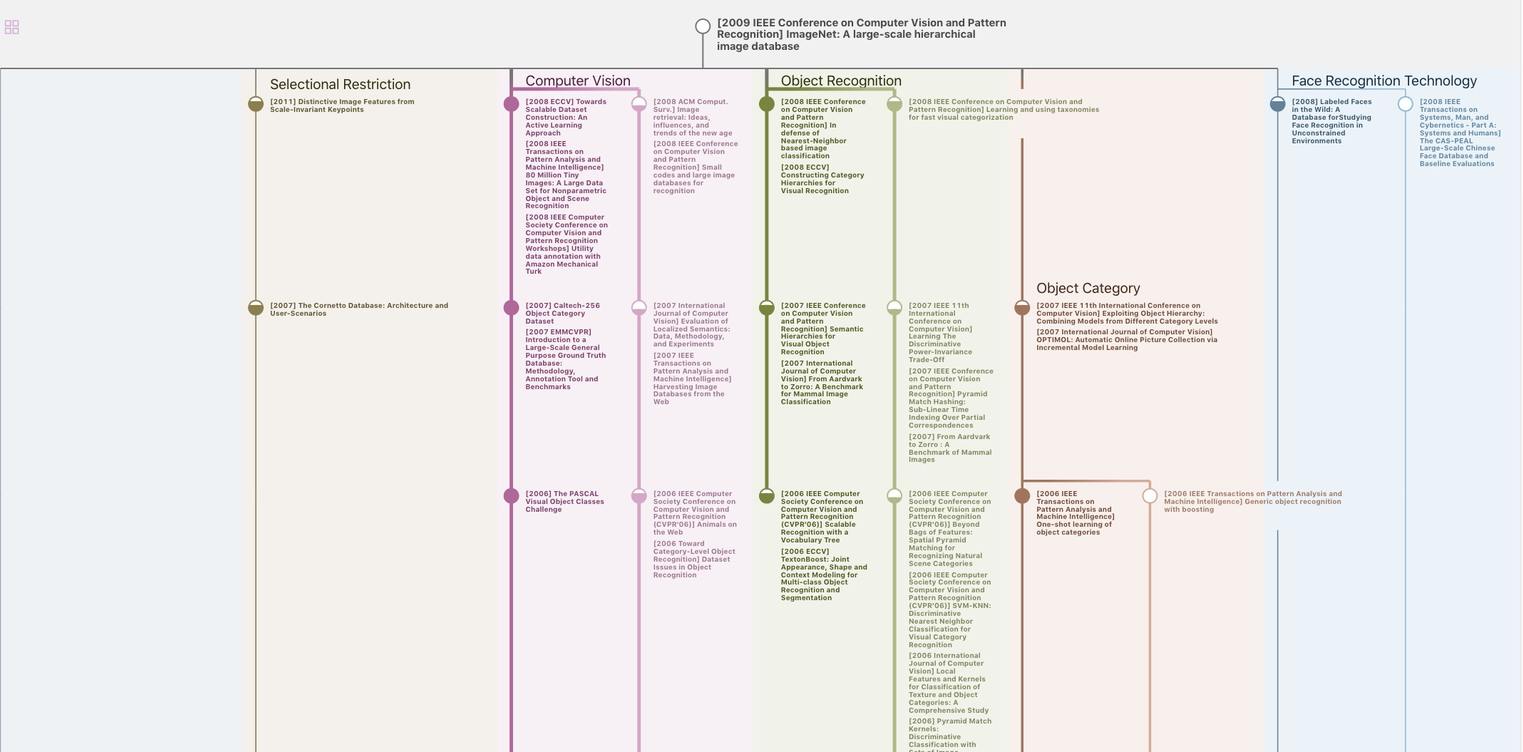
Generate MRT to find the research sequence of this paper
Chat Paper
Summary is being generated by the instructions you defined