Fault Classification Via Energy Based Features Of Two-Dimensional Image Data
COMMUNICATIONS IN STATISTICS-THEORY AND METHODS(2023)
Abstract
Automated anomaly detection is the prerequisite to minimize human errors and costs caused by manual inspection. Recently, image-based anomaly detections have gained more attention by widely adopting machine vision systems and computer-aided detections. We propose a classification method using spectral features based on 2D discrete wavelet packet transform under the hierarchical structure of wavelet energies. By capturing the self-similar and long-range dependent characteristics of 2D fractional Brownian field (fBf), wavelet packet spectra are derived to construct a linear model representing the relationship between wavelet energies and resolution levels. 2D DWPT-based energy features effectively preserve irregular oscillations in original images at high-frequency domains as well as at low-frequency domains under a pyramidal structure. In comparison with the existing 2D discrete wavelet transform method, the proposed method shows a potential in efficiently classifying normal and abnormal image data in a numerical example and a real industrial application.
MoreTranslated text
Key words
Discrete wavelet packet transform, fractional Brownian field, image classification, long-range dependence, self-similarity, spectral analysis
AI Read Science
Must-Reading Tree
Example
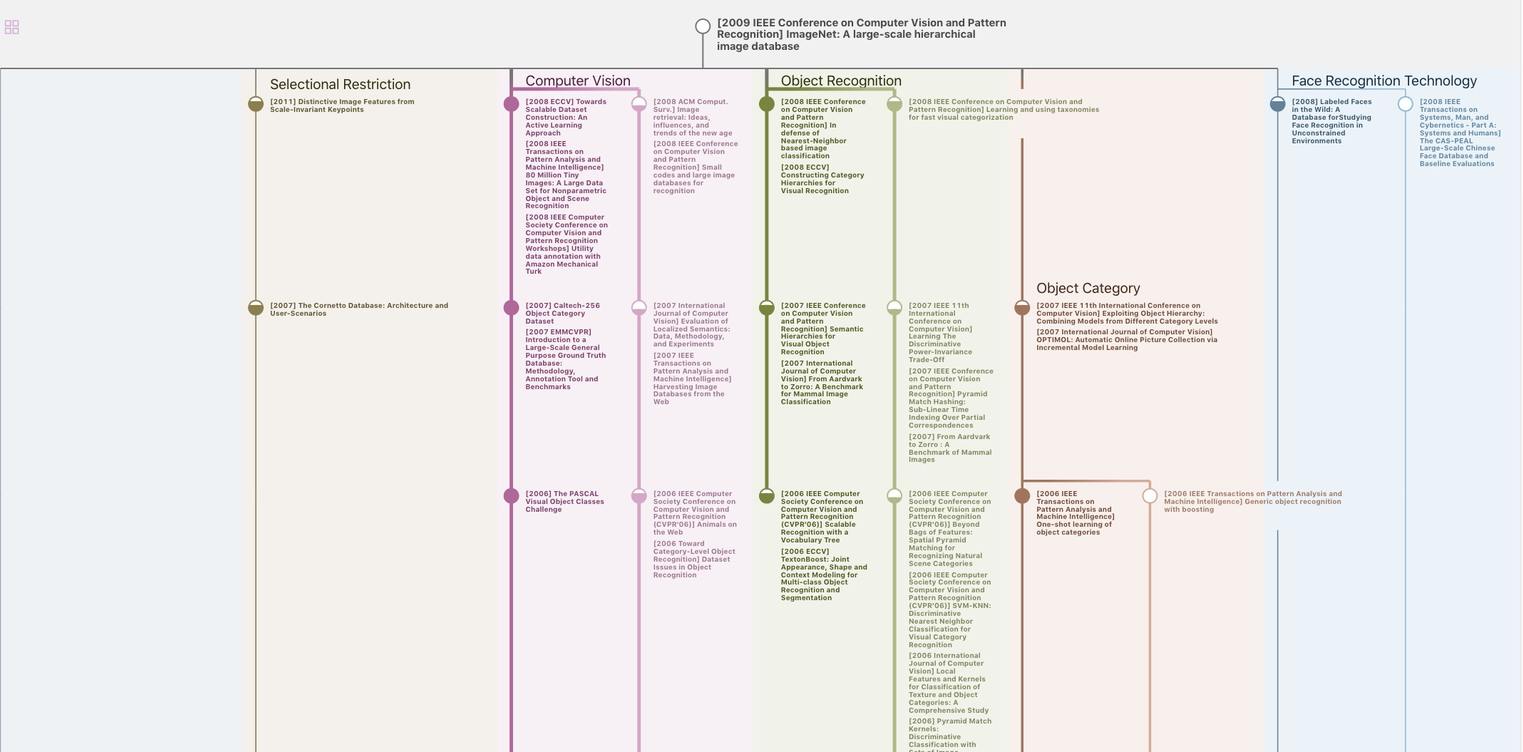
Generate MRT to find the research sequence of this paper
Chat Paper
Summary is being generated by the instructions you defined