A physics-informed deep learning method for solving direct and inverse heat conduction problems of materials
MATERIALS TODAY COMMUNICATIONS(2021)
Abstract
Due to the complex physical and chemical changes of thermal conductive materials during the heat transfer process, the research on heat transfer performance has inevitably been a hot point in thermal analysis. In this paper, a novel data-driven framework based on Physical Information Neural Networks (PINNs) is proposed to accomplish the direct analysis and parameter inversion of heat conduction problems. For the first time, two kinds of heat conduction problems are discussed under the PINNs framework simultaneously. In the phase of direct analysis, a modified PINNs framework based on adaptive activation functions is proposed. The case studies of wood and steel indicate that the proposed framework can achieve satisfactory accuracy and has the potential to replace finite element modeling to a certain extent. In the inverse analysis, inversion problems of constant and variable parameters are studied in detail. Coupled neural network frameworks with skip connections are proposed to predict unknown parameters. Experimental results represent that unknown parameters in the heat conduction equation can be accurately inversed with high computational efficiency. Compared with conventional methods, the proposed framework can solve both the direct and inverse heat conduction problems in a unified and concise form and has a broad application prospect in materials science.
MoreTranslated text
Key words
Heat conduction,Physical information neural network,Partial differential equation
AI Read Science
Must-Reading Tree
Example
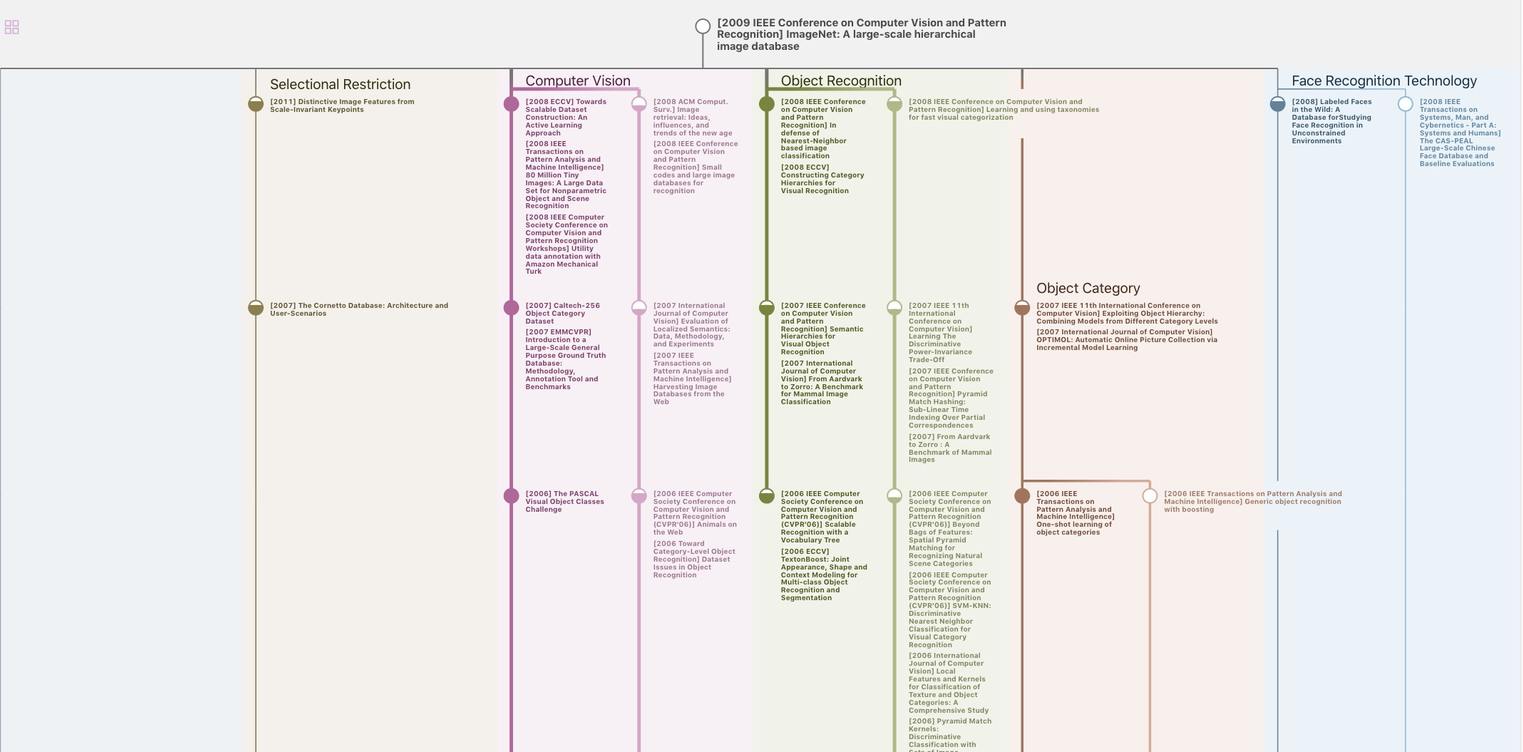
Generate MRT to find the research sequence of this paper
Chat Paper
Summary is being generated by the instructions you defined