A Novel And Efficient Feature Extraction Method For Deep Learning Based Continuous Estimation
IEEE ROBOTICS AND AUTOMATION LETTERS(2021)
摘要
Simultaneous and proportional control (SPC) methods based on surface electromyogram (sEMG) can provide a more intuitive and natural interaction in rehabilitation assistive robots and prostheses. Recently, an increasing number of researchers have utilized deep learning methods to continuously estimate movements across multiple degrees of freedom (DoFs). It stands for higher dimensional and more complex target space, which requires more comprehensive feature extraction methods. However, the existing feature extraction methods for deep learning approaches can hardly provide adequate information for multiple DoFs complex movement estimation. Therefore, we proposed a novel sEMG feature extraction method for deep learning models to more accurately predict the joint angles simultaneously on ten DoFs and tested it on eight complex movements. The experiment was carried out on four commonly adopted deep learning methods to compare the performance of the proposed feature set with others. For the four deep learning methods, the average Pearson correlation coefficient (CC) value was around 0.85 and 0.61 for the proposed feature set and raw sEMG data, respectively. Besides, the feature extraction only takes 6 mu s per 100 ms analysis window. This study suggested that the proposed feature set would improve deep learning method's application prospect on SPC for rehabilitation robotic systems.
更多查看译文
关键词
Multifingered hands, deep learning methods, rehabilitation robotics, continuous movement estimation, feature extraction
AI 理解论文
溯源树
样例
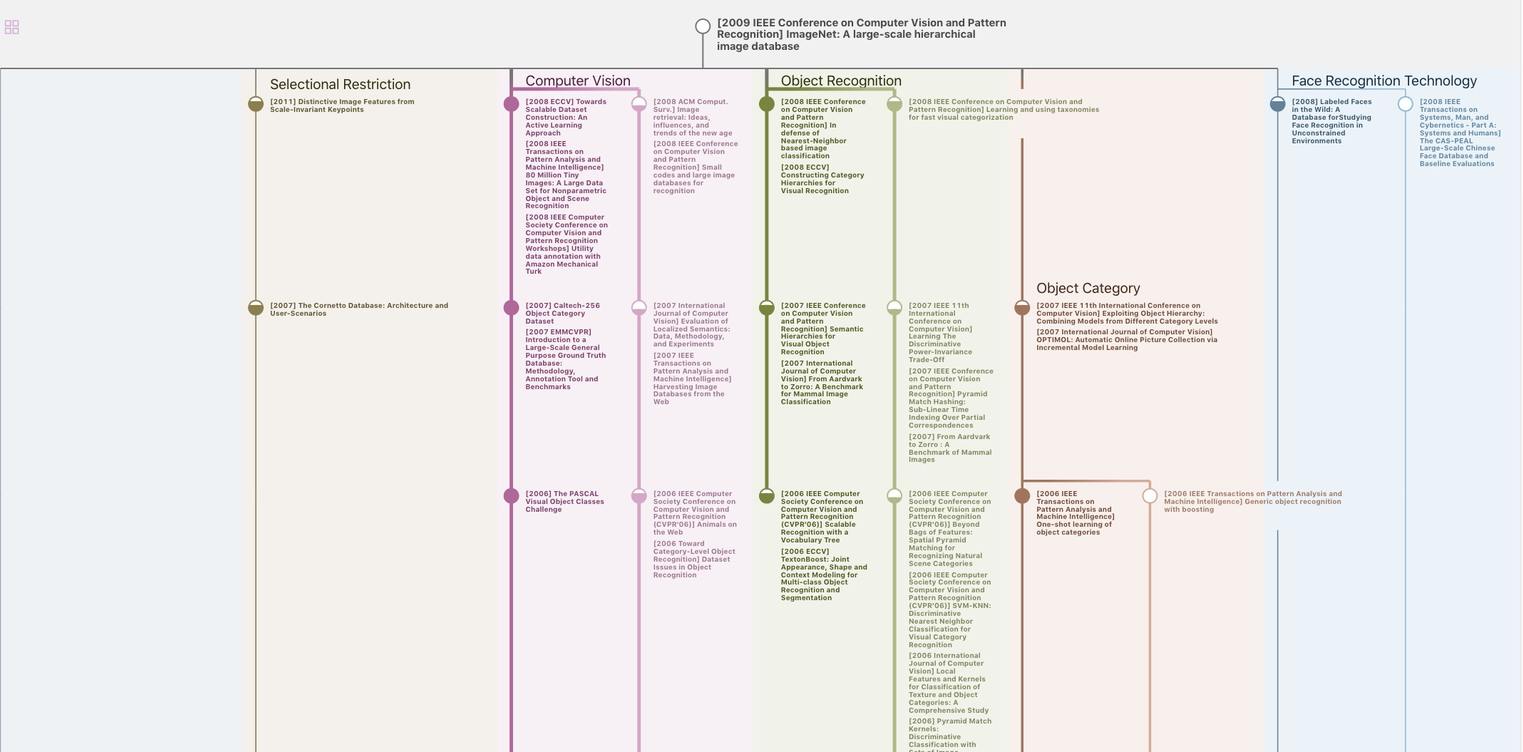
生成溯源树,研究论文发展脉络
Chat Paper
正在生成论文摘要