A novel deep learning network for accurate lane detection in low-light environments
PROCEEDINGS OF THE INSTITUTION OF MECHANICAL ENGINEERS PART D-JOURNAL OF AUTOMOBILE ENGINEERING(2022)
Abstract
Lane detection algorithms play a key role in Advanced Driver Assistance Systems (ADAS), which are however unable to achieve accurate lane recognition in low-light environments. This paper presents a novel deep network structure, namely LLSS-Net (low-light images semantic segmentation), to achieve accurate lane detection in low-light environments. The method integrates a convolutional neural network for low-light image enhancement and a semantic segmentation network for lane detection. The image quality is firstly improved by a low-light image enhancement network and lane features are then extracted using semantic segmentation. Fast lane clustering is finally performed by using the KD tree models. Cityscapes and Tusimple datasets are utilized to demonstrate the robustness of the proposed method. The experimental results show that the proposed method has an excellent performance for lane detection in low-light roads.
MoreTranslated text
Key words
Lane detection,semantic segmentation,convolutional neural networks,low-light image enhancement,deep learning
AI Read Science
Must-Reading Tree
Example
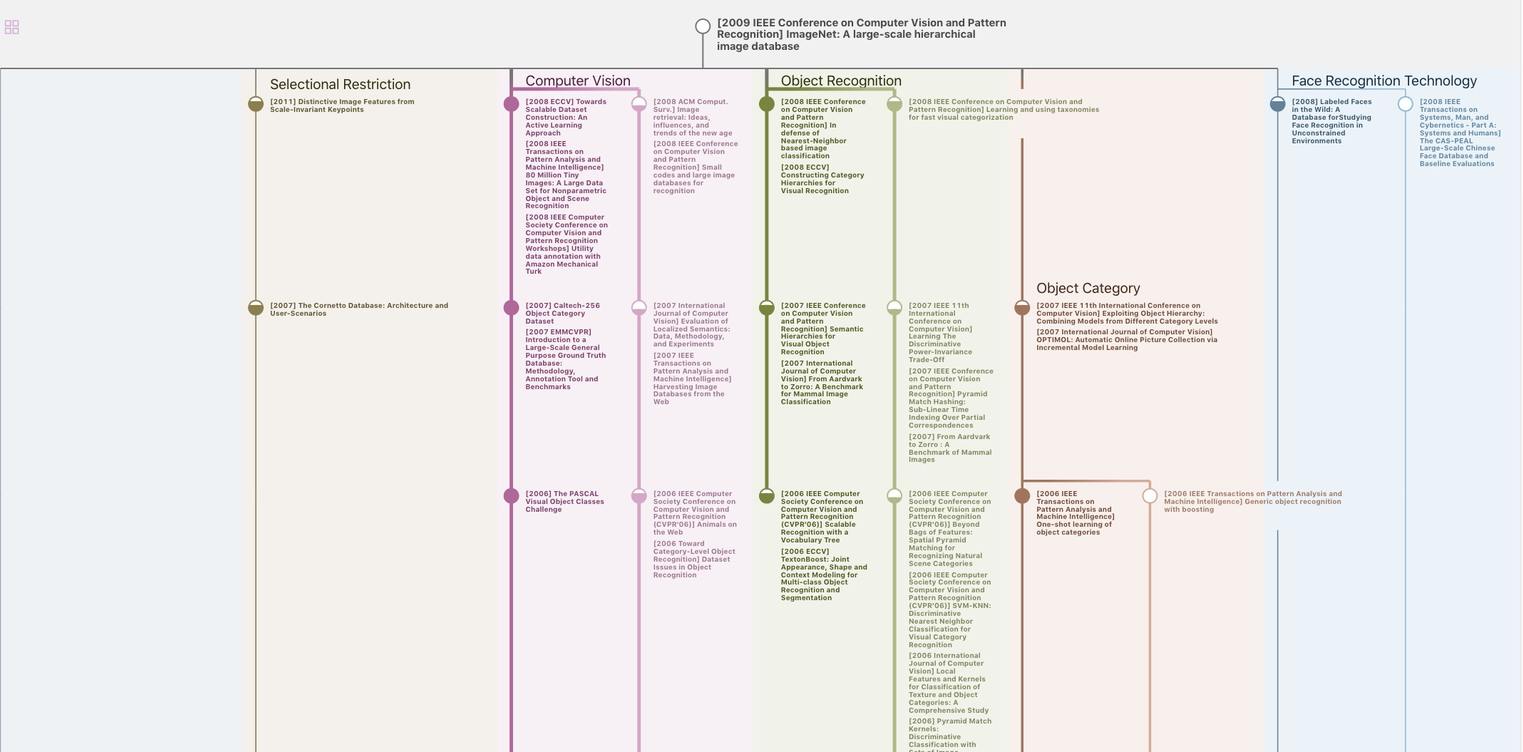
Generate MRT to find the research sequence of this paper
Chat Paper
Summary is being generated by the instructions you defined