Quality of experience (QOE) content aware hybrid lean predictive models for medical video transmission over Internet of things (IOT) networks
INTERNATIONAL JOURNAL OF COMMUNICATION SYSTEMS(2022)
摘要
The typical applications of the Internet of things (IoT) in medical video transmission have reached its new dimension for better analysis and diagnosis. As the IoT-based services may have its peak number of end-users, achieving the quality, errorless medical video transmission in high traffic networks remains to be the darker side of the research. However, the above problem in an IoT network was overcome by the integration of most intelligent machine learning and deep learning algorithms as a predictive model for the detection of medical contents under the network properties. But these algorithms also need its tuning in terms of achieving high-quality video transmission without sacrificing the performance. With all these drawbacks, this paper proposes the new hybrid predictive model LEAN (Long Effective Adaptive Networks), which works on the principle of integrating the time predictive LSTM (Long Short Term memory) with boosting machine learning algorithms. These algorithms are modeled for an efficient medical video transmission based on the novel principle of visual saliency content clustering along with the network-centric LEAN predictive models. Sufficient experimentations such as QoS evaluations along with the proposed models are conducted on real-time testbeds, which consist of Raspberry Pi 3 Model B+ interconnected with a cloud environment. Moreover, the proposed models are compared with the other existing models, such as multi-layer perceptrons and deep LSTM networks, in which the proposed predictive model demonstrates the better performance than the traditional models.
更多查看译文
关键词
deep learning, IoT, LEAN, LSTM, machine learning, multilayer Perceptron's, support vector machines, visual saliency clustering
AI 理解论文
溯源树
样例
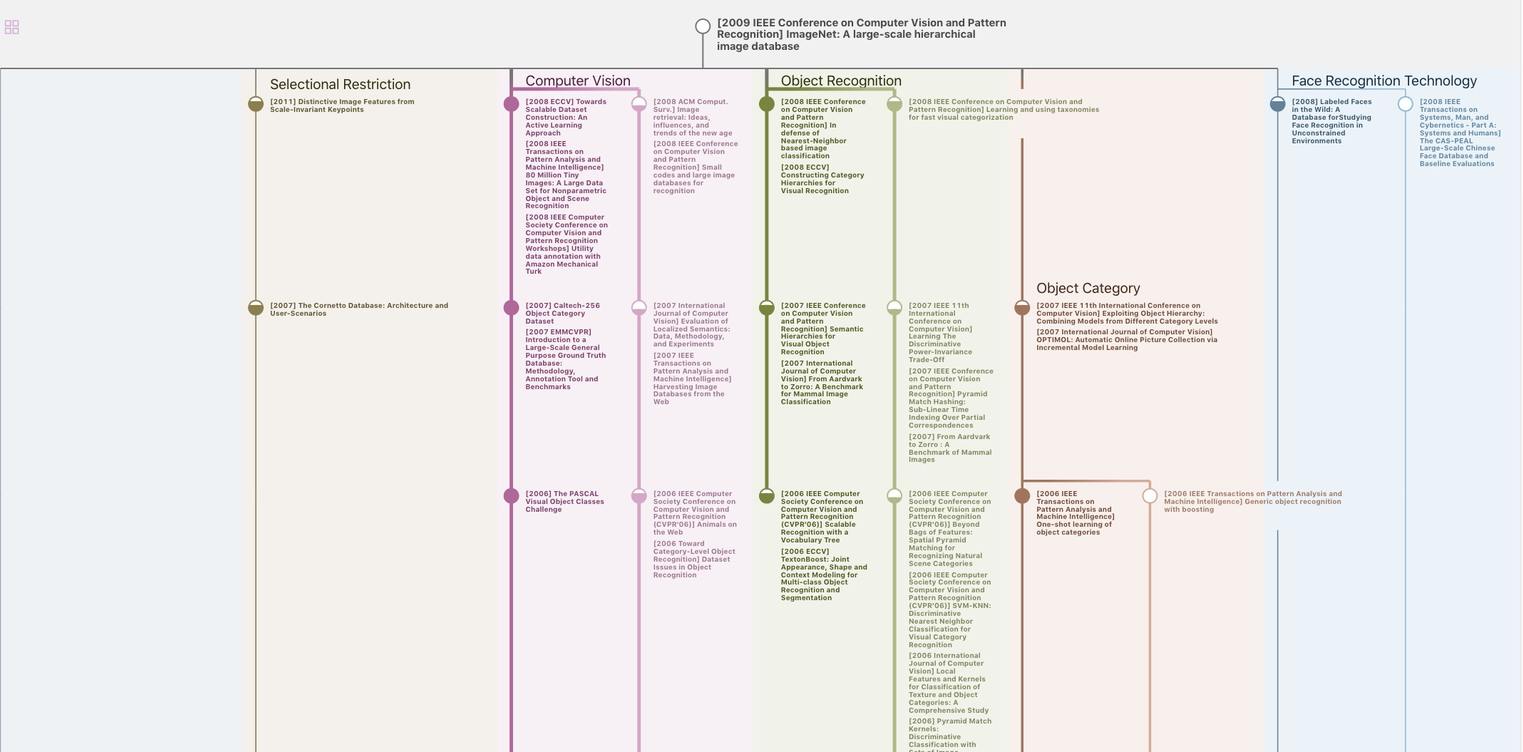
生成溯源树,研究论文发展脉络
Chat Paper
正在生成论文摘要