Hierarchical Self-Attention Embedded Neural Network With Dense Connection For Remote-Sensing Image Semantic Segmentation
IEEE ACCESS(2021)
摘要
Semantic segmentation of remote-sensing imagery strives to assign a pixel-wise semantic label. Since encoder-decoder networks have demonstrated tremendous success in natural image semantic segmentation, the adoption and extension of this kind of method are transferring such superior performance for the problems in remote-sensing. Facing the high-altitude angle of imaging and complex and diverse ground objects of remote-sensing data, it is necessary to strengthen the features' distinguishability by enhancing the network's capability. Nevertheless, the existing methods suffer from the structural stereotype, leveraging the short-range and long-range contextual information insufficiently. Attempting to address the problems mentioned above, a hierarchical self-attention embedded neural network with dense connection for remote sensing image semantic segmentation (HSDCN) is proposed. In the encoder stage, multiple self-attention modules (SAM) are embedded to model pixel-wise and channel-wise relationships at various scales hierarchically, making the representations more refined and discriminative. Then the dense connections are used to fuse the heterogeneous features. Thus, the network could produce logical and reasonable clues for labeling pixels. The extensive experiments are conducted on ISPRS Vaihingen and Potsdam benchmarks. And the results reveal significant improvements in comparison with other state-of-the-art methods.
更多查看译文
关键词
Semantics, Image segmentation, Remote sensing, Task analysis, Decoding, Computer architecture, Costs, Semantic segmentation, remote-sensing imagery, self-attention, dense connection, ISPRS benchmarks
AI 理解论文
溯源树
样例
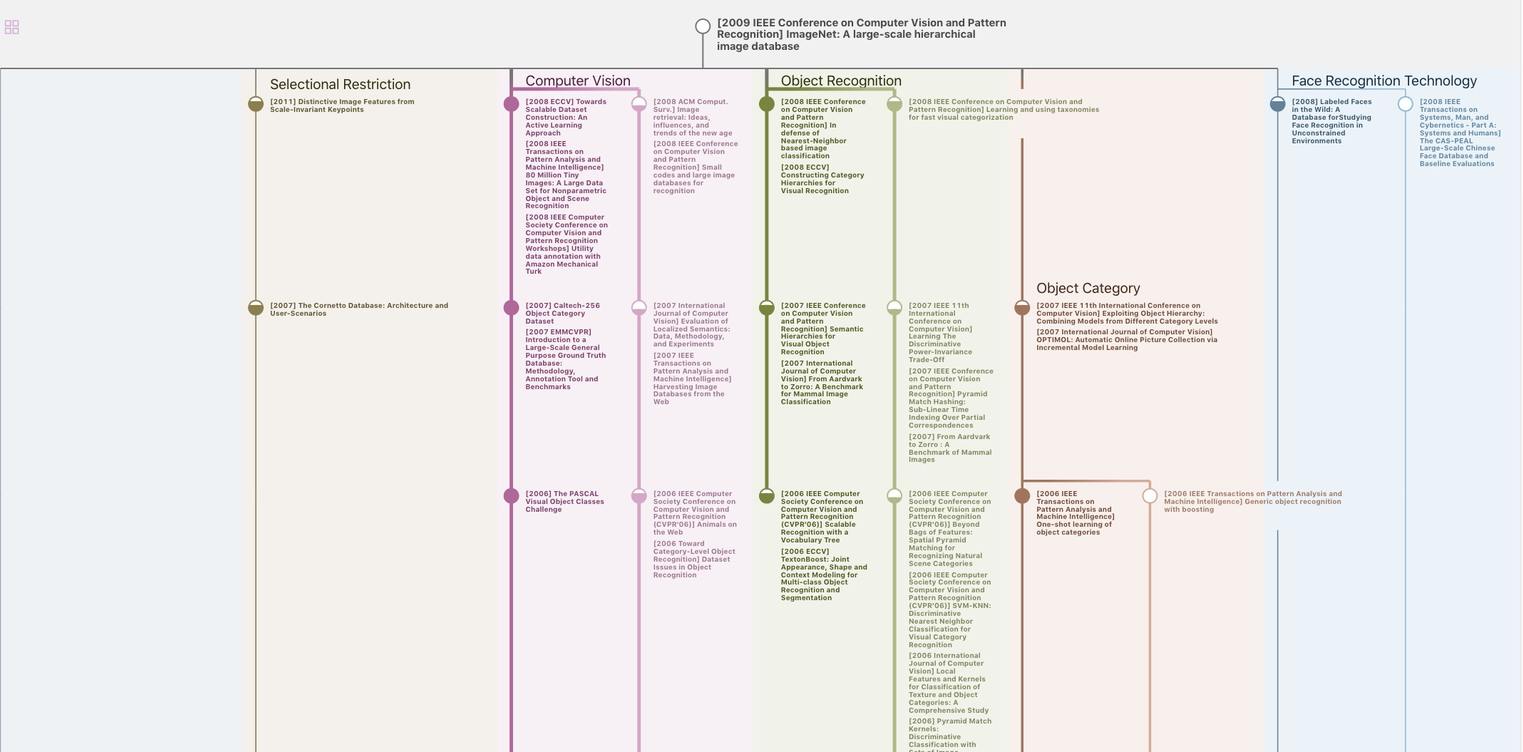
生成溯源树,研究论文发展脉络
Chat Paper
正在生成论文摘要