Power Load Forecasting Using Bilstm-Attention
2019 5TH INTERNATIONAL CONFERENCE ON ENVIRONMENTAL SCIENCE AND MATERIAL APPLICATION(2020)
Abstract
With the development of big data and artificial intelligence, the applications of smart grid have received extensive attention. Specifically, accurate power system load forecasting plays an important role in the safety and stability of the power system production scheduling process. Due to the limitations of traditional load forecasting methods in dealing with large scale nonlinear time series data, in this paper, we proposed an Attention-BiLSTM (Attention based Bidirectional Long Short-Term Memory, Attention-BiLSTM) network to do the accurate short-term power load forecasting. This model is based on BiLSTM recurrent neural network which has high robustness on time series data modeling, and the Attention mechanism which can highlight the key features playing key roles in load forecasting in input data. The verification experiments with real data in a certain area show that the proposed model outperforms other models in terms of prediction accuracy and algorithm robustness.
MoreTranslated text
Key words
Load Forecasting, Neural Networks, BiLSTM, Attention Mechanism
AI Read Science
Must-Reading Tree
Example
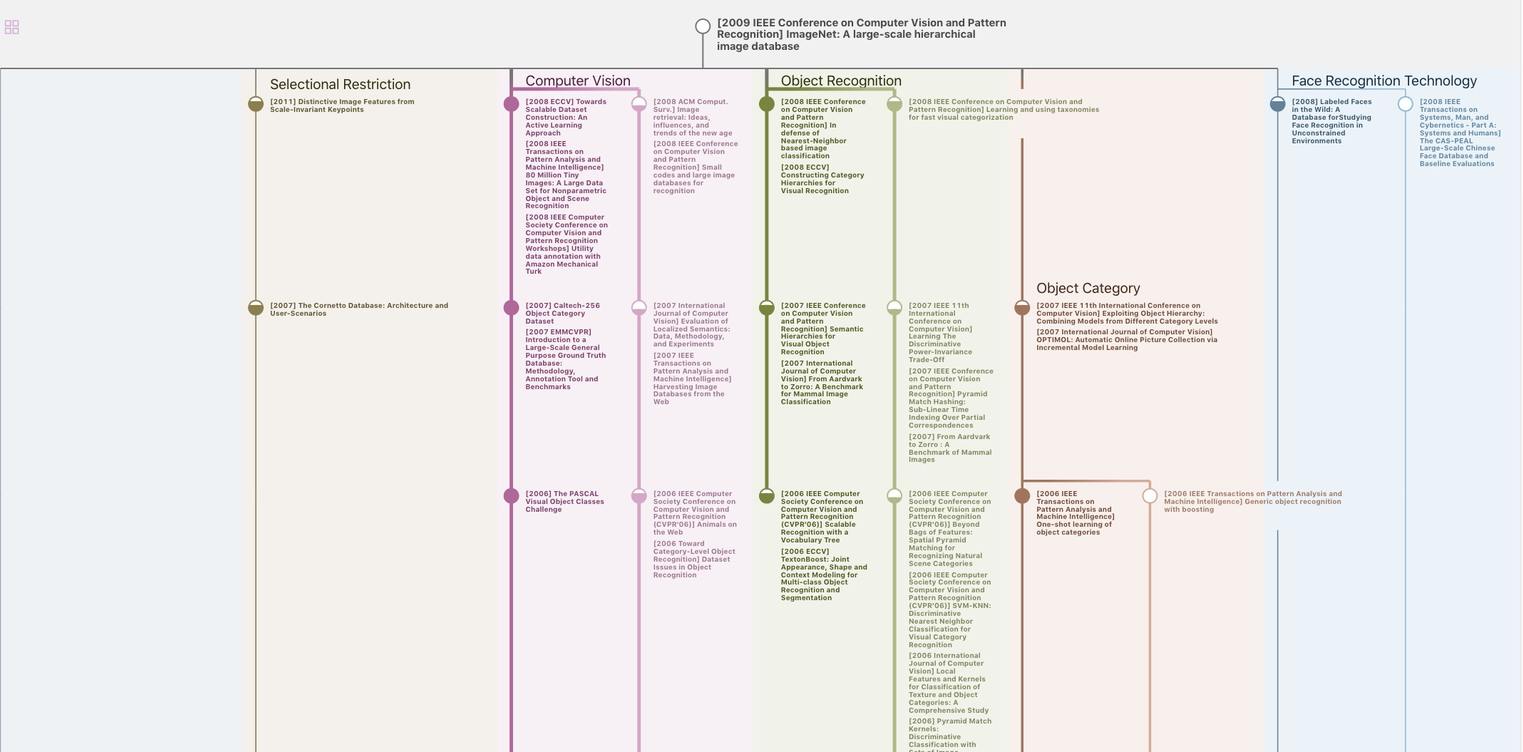
Generate MRT to find the research sequence of this paper
Chat Paper
Summary is being generated by the instructions you defined