Classifiers Vs. Input Variables - The Drivers In Single-Date And Multi-Seasonal Image Classifications For Land Cover Mapping
IMAGIN [E,G] EUROPE(2010)
Abstract
The performance of image classifiers in large-scale land cover mapping and the relevance of input variables for classification accuracy are investigated in order to assess and to quantify the importance of these components in image classification. Specifically tested are maximum likelihood classification, artificial neural networks and discriminant analysis for landscape-scale land cover assessments in the alpine region. DEM-derived ancillary data and radiometric modifications of spectral data (Landsat7 ETM+) are incorporated step-wise into the classifications to document the relevance of these input data. Including ancillary data did show large potential for increasing classification accuracy, comparable to the selection of the classifier. Multi-temporal spectral information further increased classification accuracy, despite temporary snow cover in the images.
MoreTranslated text
Key words
Classification, land cover, land use, maximum likelihood, discriminant analysis, artificial neural network, multi-temporal data, Landsat
AI Read Science
Must-Reading Tree
Example
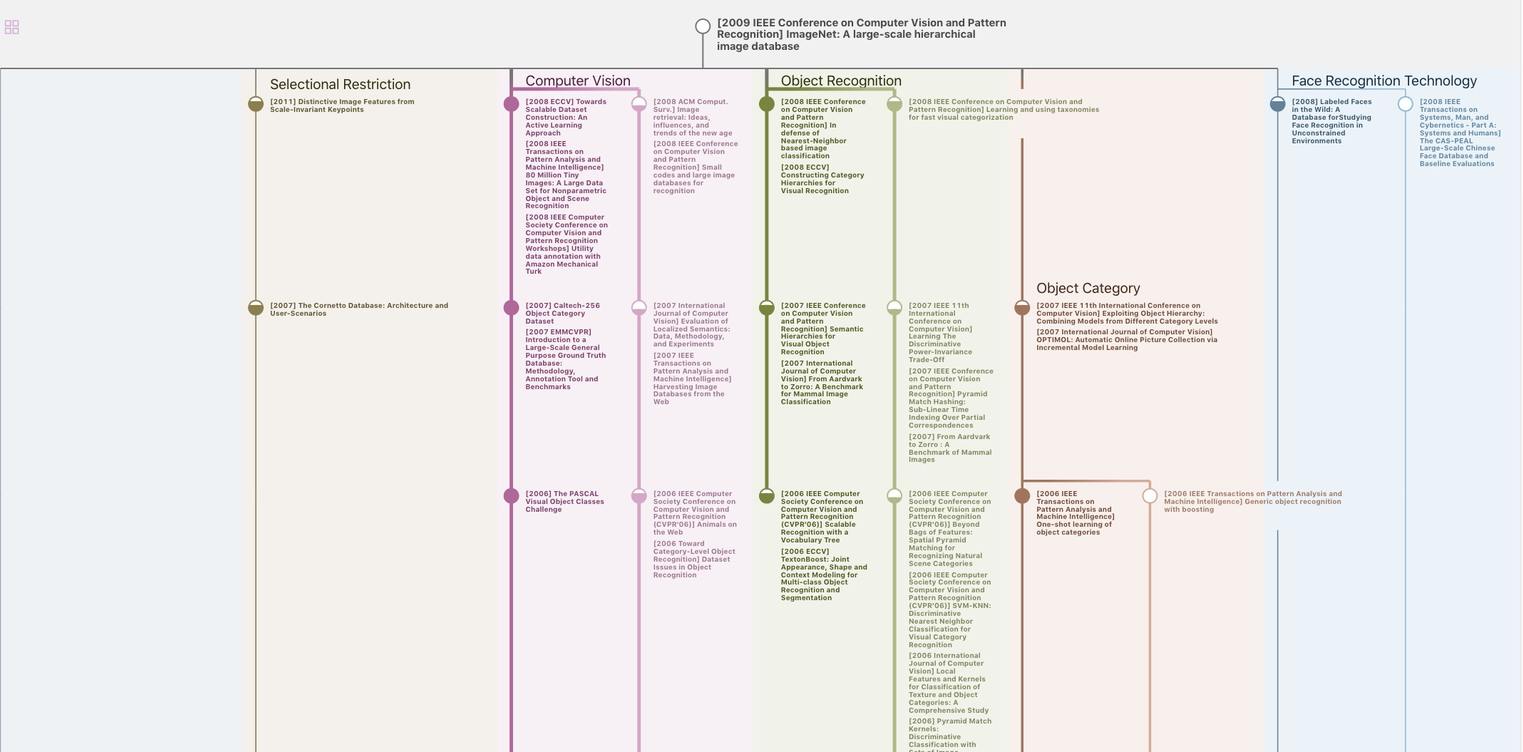
Generate MRT to find the research sequence of this paper
Chat Paper
Summary is being generated by the instructions you defined