Autonomous Crack And Bughole Detection For Concrete Surface Image Based On Deep Learning
IEEE ACCESS(2021)
Abstract
Cracks and bugholes (surface air voids) are common factors that affect the quality of concrete surfaces, so it is necessary to detect them on concrete surfaces. To improve the accuracy and efficiency of the detection, this research implements a novel deep learning technique based on DeepLabv3+ to detect cracks and bugholes on concrete surfaces. Firstly, in the decoder, the 3 x 3 convolution of the feature fusion part is improved to a 3-layer depth separable convolution to reduce the information loss during up sampling. Secondly, the original expansion rate combination is changed from 1, 6, 12, 18 to 1, 2, 4, 8 to improve the segmentation effect of the model on the image. Thirdly, a weight value is added to each channel of the Atrous Spatial Pyramid Polling (ASSP) module, and the feature maps that contribute significantly to the target prediction are learned and screened. To use this method, a database is built containing 16, 662, 256 x 256 pixel images of bugholes and cracks on concrete surfaces. The two defects included in those images are labeled manually. The DeepLabv3+ architecture is then modified, trained, validated and tested using this database. A strategy of model-based transfer learning is applied to optimize and accelerate the learning efficiency of the model. The weights and biases of the Xception part of the model are initialized by the pretrained backbones. The results are 97.63% (crack), 93.53% (bughole) Average Precision (AP), 95.58% Mean Average Precision (MAP) and 81.87% Mean Intersection over Union (MIoU). A comparative study is conducted to verify the performance of the proposed method, and the results demonstrate that the proposed approach performs significantly better in crack and bughole detection on concrete surfaces.
MoreTranslated text
Key words
Crack detection, bughole detection, deep learning, concrete surface, semantic segmentation
AI Read Science
Must-Reading Tree
Example
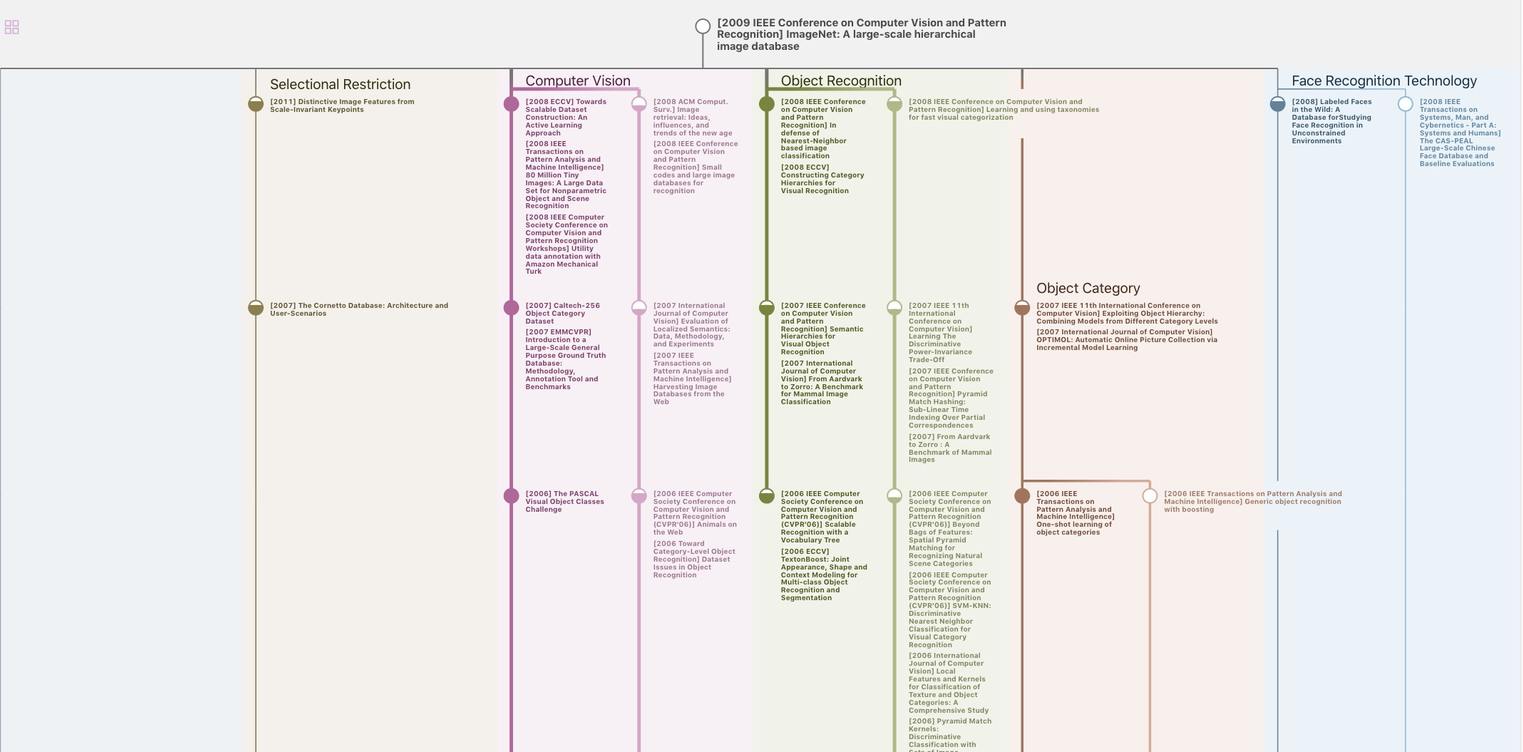
Generate MRT to find the research sequence of this paper
Chat Paper
Summary is being generated by the instructions you defined