Assessing the Generalizability of Temporally-Coherent Echocardiography Video Segmentation
MEDICAL IMAGING 2021: IMAGE PROCESSING(2021)
摘要
Existing deep-learning methods achieve state-of-art segmentation of multiple heart substructures from 2D echocardiography videos, an important step in the diagnosis and management of cardiovascular disease. However, these methods generally perform frame-level segmentation, ignoring the temporal coherence in heart motion between frames, which is a useful signal in clinical protocols. In this work, we implement temporally consistent video segmentation, which has recently been shown to improve performance on the multi-structure annotated CAMUS dataset. We show that data augmentation further improves results, which are consistent with prior state-ofart works. Our 10-fold cross-validation shows that video segmentation improves the automatic comparison to clinical indices including smaller mean absolute errors for left ventricular end-diastolic volume (8.7 mL vs 9.9 mL), end-systolic volume (6.3 mL vs 6.6 mL), and ejection fraction (EF) (4.6% vs 5.3%). In segmenting key cardiac structures, video segmentation achieves mean Dice overlap of 0.93 on left ventricular endocardium, 0.95 on left ventricular epicardium, and 0.88 on left atrium. To assess clinical generalizability, we further apply the CAMUS-trained video segmentation models, without tuning, to a larger, recently published EchoNet-Dynamic clinical dataset. On 1274 patients in the test set, we obtain absolute errors of 6.3% +/- 5.4 in EF, confirming the reliability of this scheme. In that the EchoNet-Dynamic videos contain limited annotation only for left ventricle endocardium, this effort extends at little cost generalizable, multi-structure video segmentation to a large clinical dataset.
更多查看译文
关键词
Echocardiography,Segmentation,Quantitative Image Analysis,Neural Networks
AI 理解论文
溯源树
样例
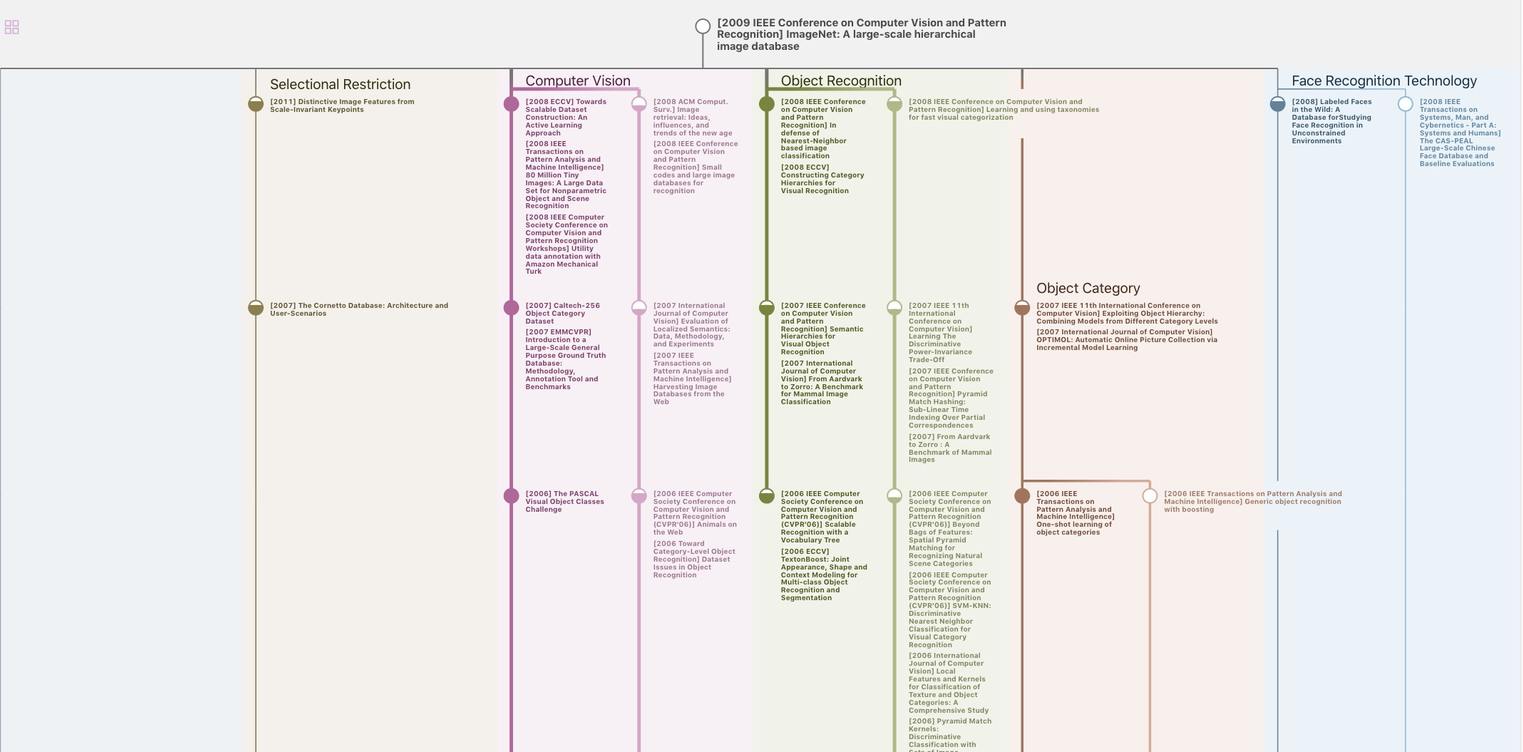
生成溯源树,研究论文发展脉络
Chat Paper
正在生成论文摘要