Renal parenchyma segmentation in abdominal MR images based on cascaded deep convolutional neural network with signal intensity correction
MEDICAL IMAGING 2021: COMPUTER-AIDED DIAGNOSIS(2021)
摘要
Segmentation of renal parenchyma responsible for renal function is necessary to evaluate contralateral renal hypertrophy and to predict renal function after renal partial nephrectomy (RPN). Although most studies have segmented the kidney on CT images to analyze renal function, renal function analysis is required without radiation exposure by segmenting the renal parenchyma on MR images. However, renal parenchyma segmentation is difficult due to small area in the abdomen, blurred boundary, large variations in the shape of kidney among patients, similar intensities with nearby organs such as the liver, spleen and vessels. Furthermore, signal intensity is different for each data due to a lot of movement when taking abdominal MR even when photographed with the same device. Therefore, we propose cascaded deep convolutional neural network for renal parenchyma segmentation with signal intensity correction in abdominal MR images. First, intensity normalization is performed in the whole MR image. Second, kidney is localized using 2D segmentation networks based on attention U - Net on the axial, coronal, sagittal planes and combining through a majority voting. Third, signal intensity correction between each data is performed in the localized kidney area. Finally, renal parenchyma is segmented using 3D segmentation network based on U-Net++. The average DSC of renal parenchyma was 91.57%. Our method can be used to assess contralateral renal hypertrophy and to predict renal function by measuring volume change of the renal parenchyma on MR images without radiation exposure instead of CT images, and can establish basis for treatment after RPN.
更多查看译文
关键词
Abdominal MR images,Renal parenchyma segmentation,Deep convolutional neural network,Majority voting,Signal intensity correction
AI 理解论文
溯源树
样例
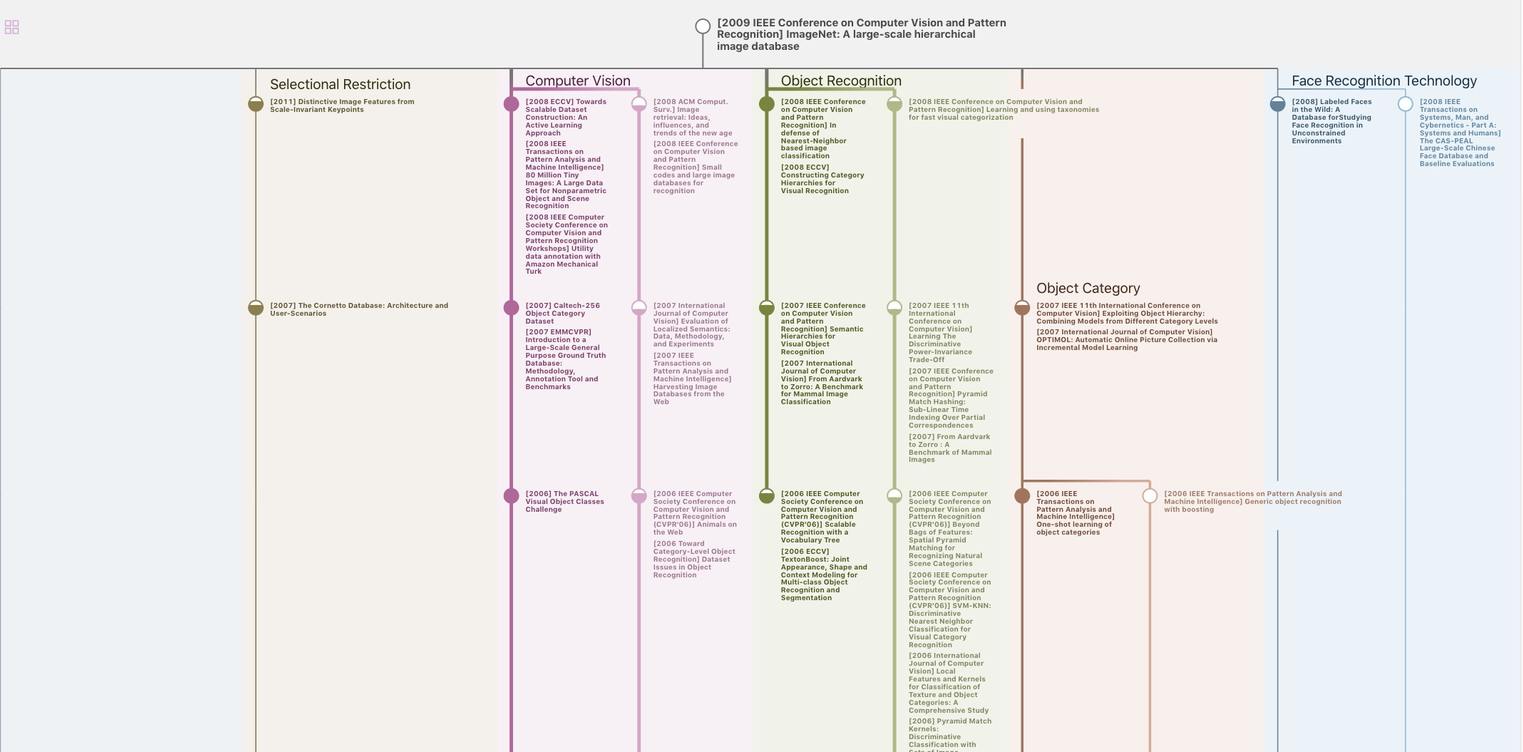
生成溯源树,研究论文发展脉络
Chat Paper
正在生成论文摘要