An Efficient Subset Simulation based on the Active Learning Kriging model for Structural Reliability Analysis
2020 3RD WORLD CONFERENCE ON MECHANICAL ENGINEERING AND INTELLIGENT MANUFACTURING (WCMEIM 2020)(2020)
摘要
Active learning Kriging model-based Monte Carlo simulation (AK-MCS) is a powerful reliability analysis method. However, the AK-MCS is time-consuming due to the large size of sample pool under the analysis of a rare failure event. Therefore, an efficient reliability analysis method combining with the active learning Kriging model and Subset simulation (SS) is proposed in this research. In the proposed strategy, the failure probability is transformed as the product of a series of larger conditional probability of the adaptively selected intermediate failure events. The approximated models of these limit state surfaces are accurately constructed by a novel active learning Kriging model in the proposed method. By the new learning function extracted from the variance of the corresponding conditional failure probability, the accuracy of the corresponding approximated model can efficiently improve. The proposed method can significantly reduce the calculational burden of evaluating the actual complicated performance function compared with AK-MCS. Two examples are employed to demonstrate the efficiency and accuracy of the proposed method.
更多查看译文
关键词
Structural reliability analysis, Active Learning Kriging model, Subset simulation, Error measure formula
AI 理解论文
溯源树
样例
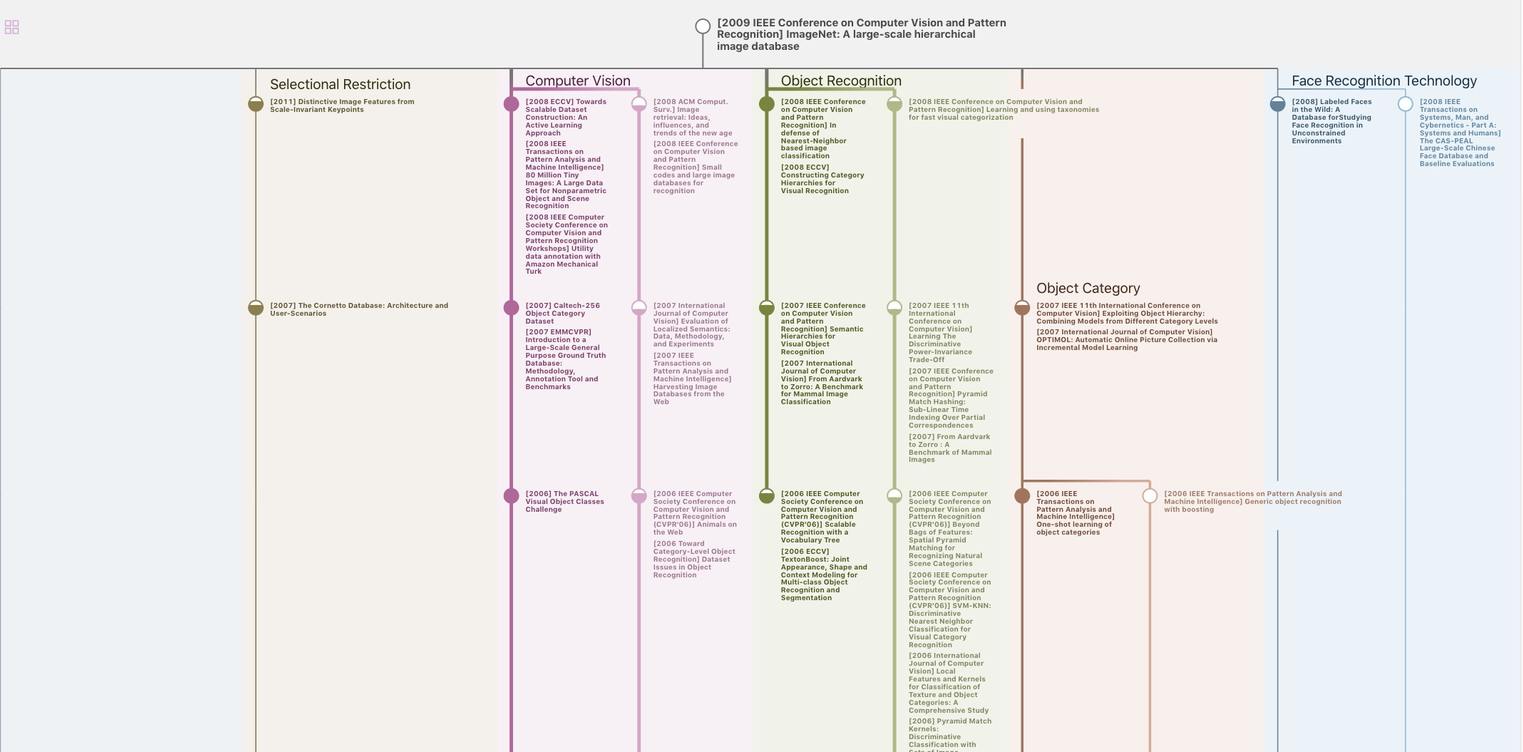
生成溯源树,研究论文发展脉络
Chat Paper
正在生成论文摘要