Physics Based Feature Vector Design: A Critical Step Towards Machine Learning Based Inverse Lithography
OPTICAL MICROLITHOGRAPHY XXXIII(2021)
摘要
Computational lithography has been playing a critical role in enabling the semiconductor industry. After source mask co-optimization ( SMO), inverse lithography has become the ultimate frontier of computational lithography. Full chip implementation of rigorous inverse lithography remains impractical because of enormous computational hardware resource requirements and long computational time, the situation exacerbates for EUV computational lithography where mask 3D effect is more pronounced. One very promising technique to overcome the barrier is to take full advantage of the maturing machine learning techniques based on neural network architecture. Some success has been achieved using deep convolution neural network (DCNN) to obtain inverse lithography technology (ILT) solution with significantly less computational time. In DCNN, to extract features with sufficient resolution and nearly complete representation, the feature extract layers are very complicated and lack of physical meaning. More importantly, the training requires large number of well balanced samples, which makes the training more difficult and time consuming. To alleviate the difficulties relating to DCNN, we have proposed the physics based optimal feature vector design for machine learning based computational lithography. The innovative physics based feature vector design eliminates the need of feature extraction layers in neural network, only layers for mapping function construction are needed, which greatly reduces the NN training time and accelerates the NN model SRAF generation for full chip. In this paper, we will present our machine learning based inverse lithography results with adaptive and dynamical sampling scheme for neural network training.
更多查看译文
关键词
Optimal feature vector design, inverse lithography technology (ILT), deep convolution neural network (DCNN)
AI 理解论文
溯源树
样例
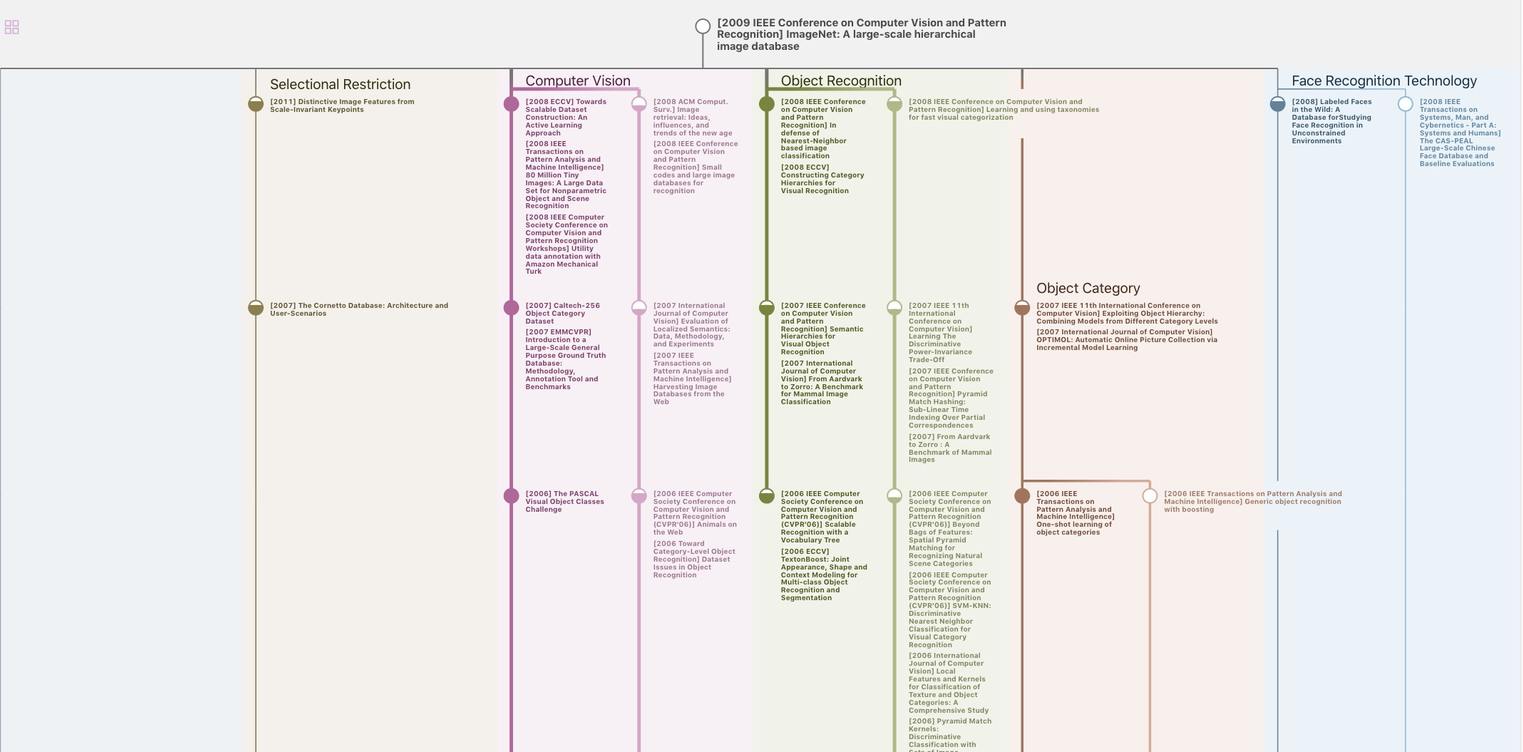
生成溯源树,研究论文发展脉络
Chat Paper
正在生成论文摘要