Establishing Fast, Practical, Full-chip ILT Flows Using Machine Learning
OPTICAL MICROLITHOGRAPHY XXXIII(2021)
摘要
Since its introduction at Luminescent Technologies and continued development at Synopsys, Inverse Lithography Technology (ILT) has delivered industry leading quality of results (QOR) for mask synthesis designs. With the advent of powerful, widely deployed, and user-friendly machine learning (ML) training techniques, we are now able to exploit the quality of ILT masks in a ML framework which has significant runtime benefits. In this paper we will describe our MLILT flow including training data selection and preparation, network architectures, training techniques, and analysis tools. Typically, ILT usage has been limited to smaller areas owing to concerns like runtime, solution consistency, and mask shape complexity. We will exhibit how machine learning can be used to overcome these challenges, thereby providing a pathway to extend ILT solution to full chip logic design. We will demonstrate the clear superiority of ML-ILT QOR over existing mask synthesis techniques, such as rule based placements, that have similar runtime performance.
更多查看译文
关键词
Inverse Lithography Technology,ILT,Machine Learning,OPC,Neural Networks
AI 理解论文
溯源树
样例
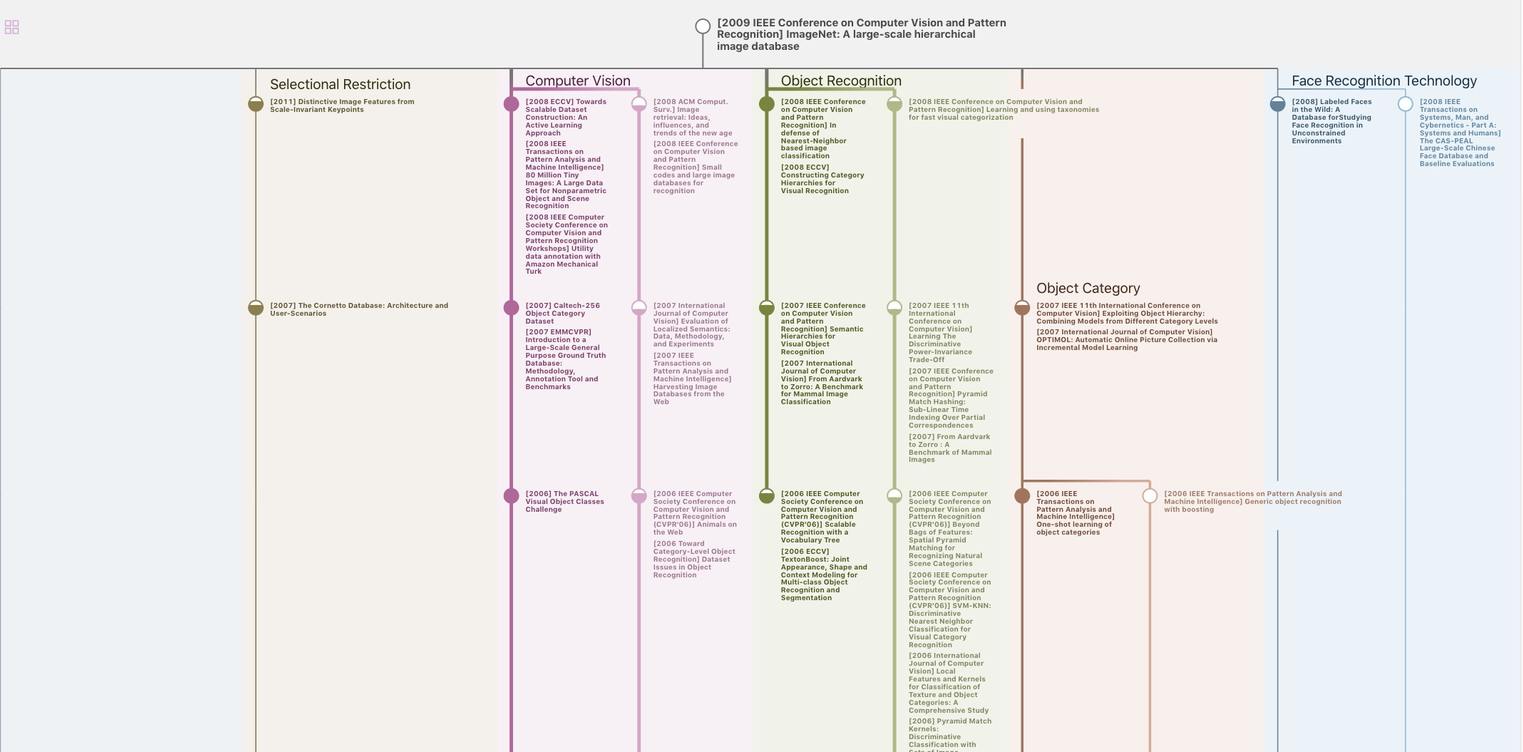
生成溯源树,研究论文发展脉络
Chat Paper
正在生成论文摘要