Deep Neural Networks For Low-Dose Ct Image Reconstruction Via Cooperative Meta-Learning Strategy
MEDICAL IMAGING 2020: PHYSICS OF MEDICAL IMAGING(2021)
Abstract
Recently, deep neural networks (DNNs) have been widely applied in low-dose computed tomography (LDCT) imaging field. Their performances are highly related to the number of the pre-collected training data. Meanwhile, the training data is usually hard to obtain, especially for the high-dose CT (HDCT) images. And HDCT images sometimes contain undesired noises, which easily result in network overfitting. To address the two issues, we proposed a cooperative meta-learning strategy for CT image reconstruction (CmetaCT) combining the meta-learning strategy and Co-teaching strategy. The meta-learning (teacher/student model) strategy allows for training network with a large number of LDCT images without the corresponding HDCT images and only a small number of labeled CT data in a semi-supervised learning manner. And the Co-teaching strategy is able to make a trade-off between overfitting and introducing extra errors, which includes a part of samples in every mini-batch for updating model parameters. Due to the capacity of meta-learning, the presented CmetaCT method is flexible enough to utilize any existing CT restoration/reconstruction network in meta-learning framework. Finally, both quantitative and visual results indicated that the proposed CmetaCT method achieves a superior performance on low-dose CT imaging compared with the DnCNN method.
MoreTranslated text
Key words
Low-dose CT, image reconstruction, deep neural network, meta-learning, Co-teaching
AI Read Science
Must-Reading Tree
Example
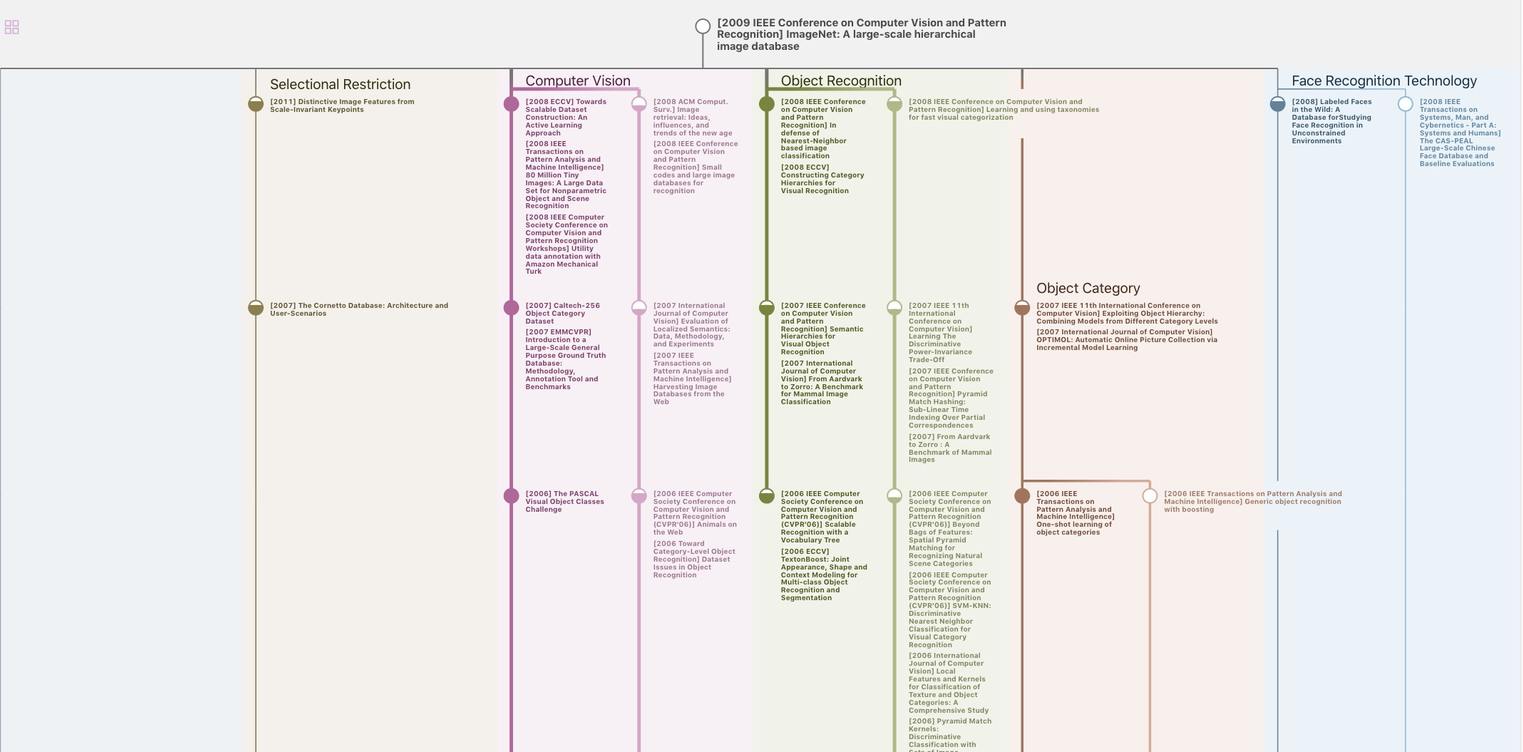
Generate MRT to find the research sequence of this paper
Chat Paper
Summary is being generated by the instructions you defined