Deep Learning Enabled Prior Image Constrained Compressed Sensing (Dl-Piccs) Reconstruction Framework For Sparse-View Reconstruction
MEDICAL IMAGING 2020: PHYSICS OF MEDICAL IMAGING(2021)
摘要
This work aims to combine compressed sensing reconstruction with a deep learning framework to leverage their individual strengths and enable 123-view moderate-dose sparse-view reconstruction for diagnostic CT imaging systems. Specifically, linear FBP reconstruction was applied to reconstruct sparse data, followed by a trained U-Net to remove artifacts resulting from undersampling. This methodology has been exploited in many other deep learning applications for CT imaging. However, this approach may be subjected to the generalizability issue in which the reconstruction can either remove real lesions or add lesions that may not exist. This will be demonstrated in our results. In our proposed work, the output of the network is used as the prior image for a prior image constrained compressed sensing (PICCS) reconstruction. This step helps to ensure the reconstructed image is consistent with the measured data. Finally, the PICCS reconstructed image is further cleaned up by a trained light duty U-Net to improve noise texture and reduce noise to generate the final reconstructed image. Both simulation data and human subject data were used to validate the proposed image reconstruction framework. In simulation studies, it is demonstrated that the final output corrected the distorted structures in the deep learning-only reconstruction with respect to the shape, size and contrast of the structures. The final images also appeared streak-free with more natural noise texture when compared with the PICCS-only reconstruction. In human subject validation, the false positive lesion-like structures in the deep learning prior image were eliminated in the final output.
更多查看译文
AI 理解论文
溯源树
样例
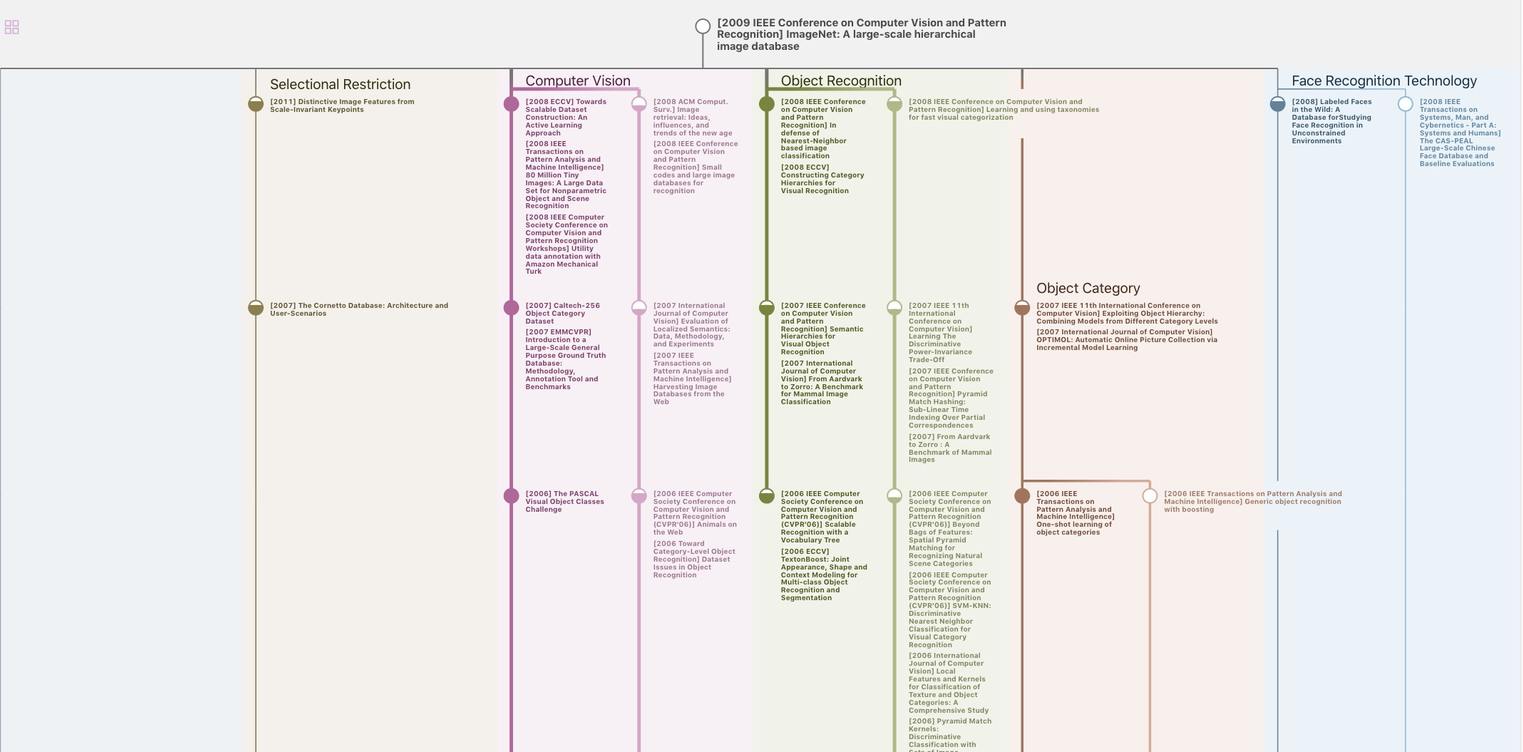
生成溯源树,研究论文发展脉络
Chat Paper
正在生成论文摘要