Unsupervised Data Fidelity Enhancement Network For Spectral Ct Reconstruction
MEDICAL IMAGING 2020: PHYSICS OF MEDICAL IMAGING(2021)
摘要
Deep learning (DL) networks show a great potential in computed tomography (CT) imaging field. Most of them are supervised DL network greatly based on their capability and the amount of CT training data (i.e., low-dose CT measurements/high-quality ones). However, collection of large-scale CT datasets are time-consuming and expensive. In addition, the training and testing CT datasets used for supervised DL network are highly desired similarities in CT scan protocol (i.e., similar anatomical structure, and same kVp setting). These two issues are particularly critical in spectral CT imaging. In this work, to address the issues, we presents an unsupervised data fidelity enhancement network (USENet) to produce high-quality spectral CT images. Specifically, the presented USENet consists of two parts, i.e., supervised network and unsupervised network. In the supervised network, the spectral CT image pairs at 140 kVp (low-dose CT images/high-dose ones) are used for network training. It should be noted that there is a great difference of CT value between spectral CT images at 140 kVp and 80 kVp, and the supervised network trained with CT images at 140 kVp cannot be directly used for CT image reconstruction at 80 kVp. Then unsupervised network enrolls physical model and the spectral CT measurements at 80 kVp for fine-tuning the supervised network, which is the major contribution of the presented USENet method. Finally, accurate spectral CT reconstructions are achieved for the sparse-view and low-dose cases, which fully demonstrate the effectiveness of the presented USENet method.
更多查看译文
关键词
Supervised learning, Unsupervised learning, Spectral CT, CT reconstruction
AI 理解论文
溯源树
样例
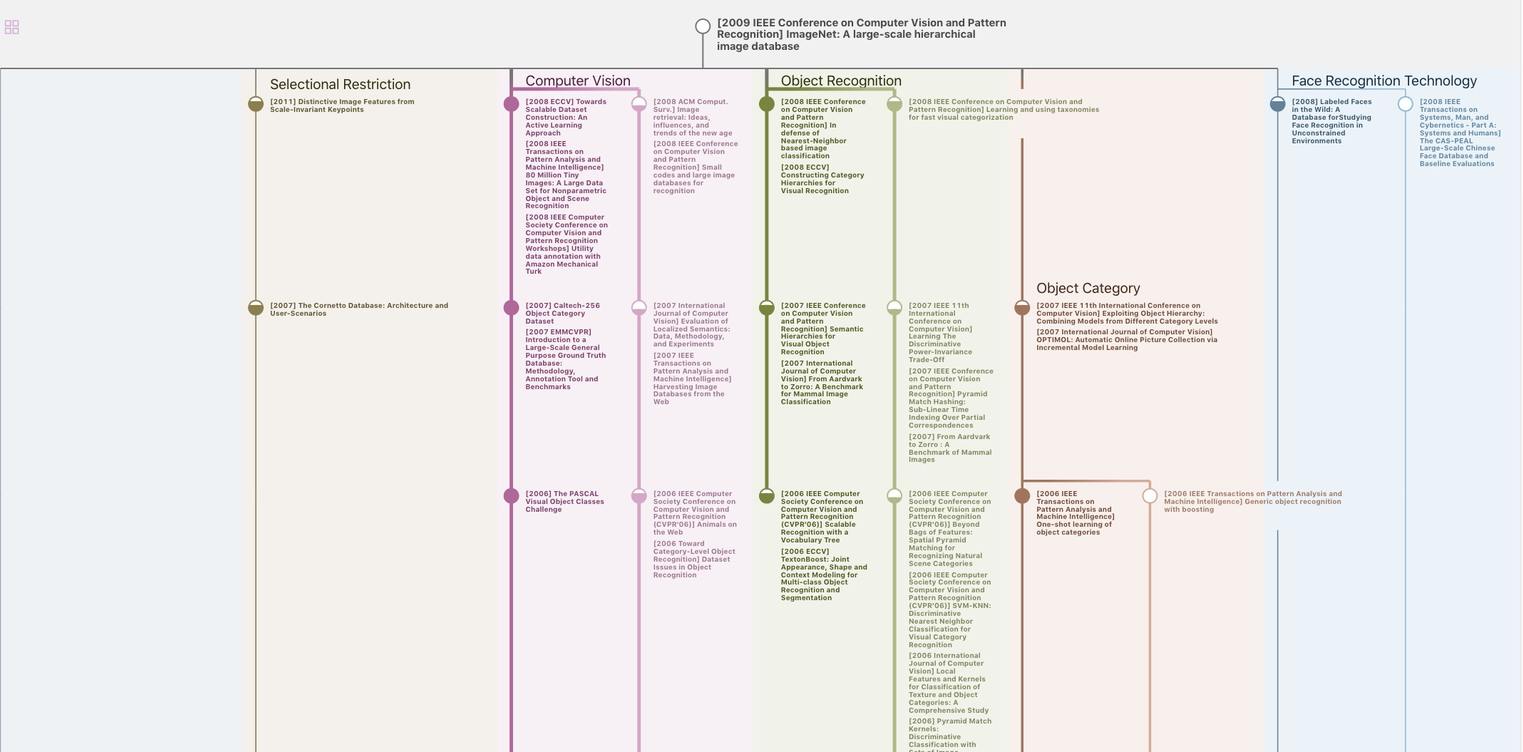
生成溯源树,研究论文发展脉络
Chat Paper
正在生成论文摘要