Neural network autoregression and classical time series approaches for rice yield forecasting
JOURNAL OF ANIMAL AND PLANT SCIENCES-JAPS(2021)
摘要
This study deals with the application of classical time series models such as double moving average method, exponential smoothing (Brown's double exponential smoothing method and Holt's double exponential smoothing method) along with neural network autoregression model for rice yield prediction in Karnal district of Haryana (India). The district annual time series data on rice productivity were divided into the training data set from 1980-81 to 2013-14 and the test data set from 2014-15 to 2019-20. Among the models fitted, the neural network model had a significantly lower absolute error and the root mean square error. During the test period, a markedly low mean absolute percent deviation was observed for the moving average (7x7) model. However, Future forecast values by this model surpass a fair threshold for rice yield in the country. Considering both the error analysis and model validation results it is found that neural network forecasting models are best fit for forecasting of rice yield in Karnal district followed by Holts double exponential method.
更多查看译文
关键词
Artificial neural network,Exponential smoothing,Moving average,Crop yield forecasting
AI 理解论文
溯源树
样例
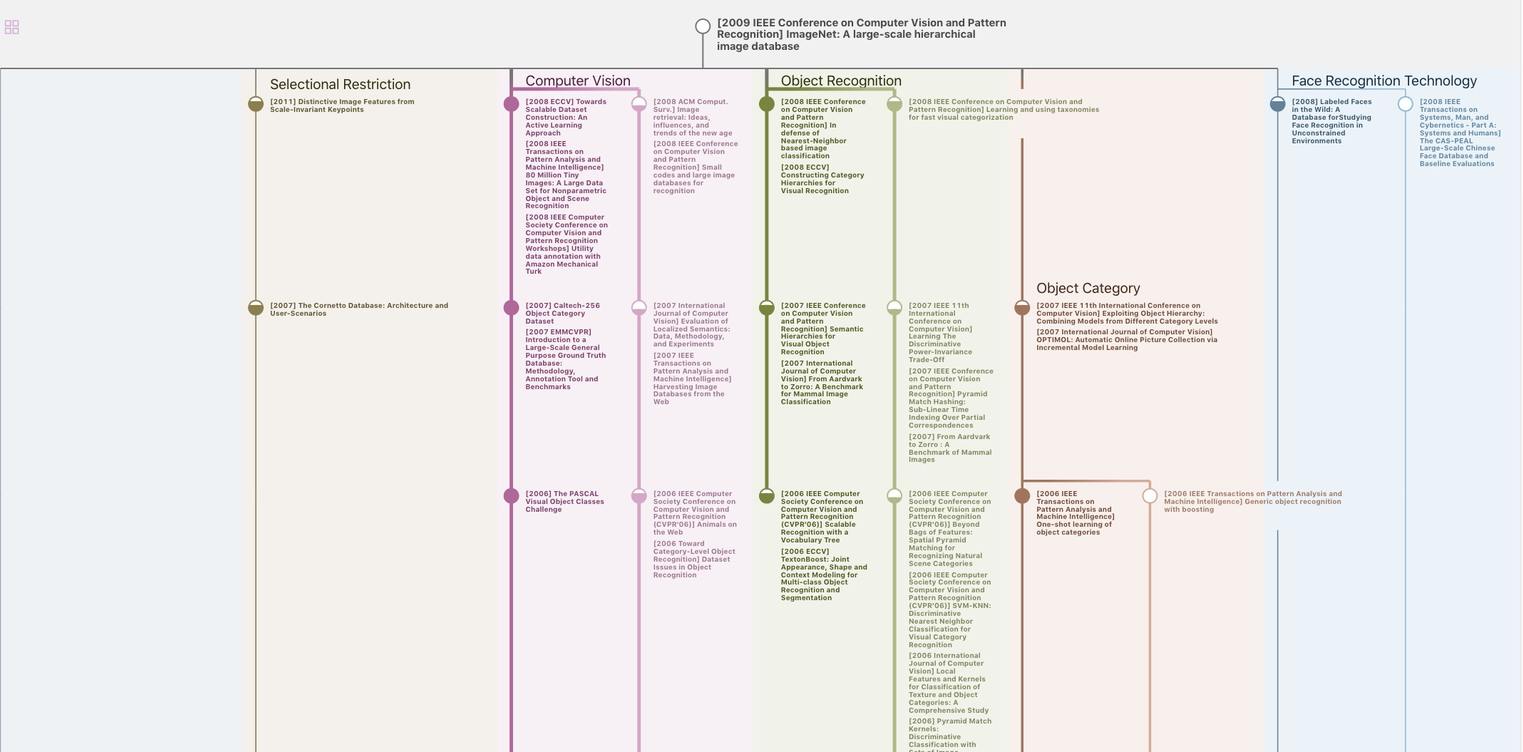
生成溯源树,研究论文发展脉络
Chat Paper
正在生成论文摘要