Optimization Of Formulations Using Robotic Experiments Driven By Machine Learning Doe
CELL REPORTS PHYSICAL SCIENCE(2021)
摘要
Formulated products are complex mixtures of ingredients whose time to market can be difficult to speed due to the lack of general predictable physical models for the desired properties. Here, we report the coupling of a machine learning classification algorithm with the Thompson sampling efficient multiobjective optimization (TSEMO) algorithm for the simultaneous optimization of continuous and discrete outputs. The methodology is successfully applied to the design of a formulated liquid product of commercial interest for which no physical models are available. Experiments are carried out in a semiautomated fashion using robotic platforms triggered by the machine learning algorithms. The procedure allows one to find nine suitable recipes meeting the customer-defined criteria within 15 working days, outperforming human intuition in the target performance of the formulations.
更多查看译文
关键词
liquid formulations,machine learning,robotic experiments,high-throughput experiments,formulations design,product design,multi-objective optimization,Bayesian optimization,lab automation
AI 理解论文
溯源树
样例
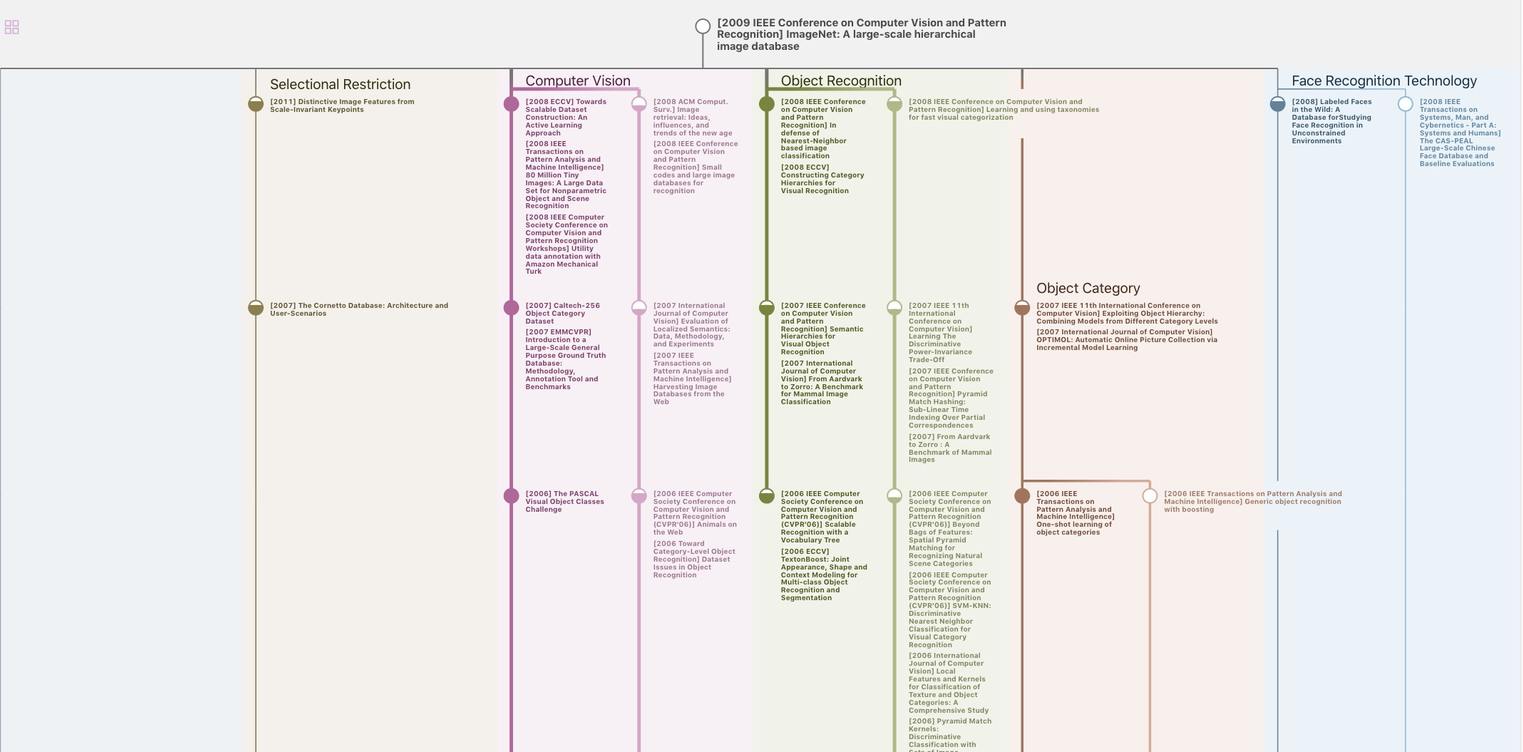
生成溯源树,研究论文发展脉络
Chat Paper
正在生成论文摘要