Importance Of Satellite Observations For High-Resolution Mapping Of Near-Surface No2 By Machine Learning
REMOTE SENSING OF ENVIRONMENT(2021)
摘要
Nitrogen dioxide (NO2) is an important air pollutant with negative health effects and a precursor of ozone and particulate matter responsible for photo-chemical smog and wintertime air pollution. To evaluate human exposure to NO2 for public health assessment, maps of near-surface NO2 concentrations at a high resolution of 100 m are desirable. In this study, we report hourly maps of gridded near-surface NO2 concentrations that are produced using an extreme gradient-boosted tree ensemble for an Alpine domain (Switzerland and northern Italy) spanning two years, from June 2018 to May 2020. To estimate the NO2 distribution at ground level, we used satellite observations of NO2 vertical column density, land use data, meteorological fields and topographical information to train models with in situ NO2 ground measurements. The best model with this approach captured up to 59% of hourly NO2 variation for 40 test stations in the domain with a mean absolute error of 7.69 mu g/m(3), performing especially well for urban regions with dense sampling. We present the first hourly maps of NO2 concentrations that reveal previously unresolved spatio-temporal variations. Local interpretations of the machine learning model demonstrate that TROPOMI NO2 satellite observations make a strong contribution to the information content of the near-surface NO2 maps besides their relatively coarse resolution (3.5 x 5.5 km(2)) and the fact that they are only available once a day under cloud-free conditions. The COVID-19 pandemic lockdown presents a case study that offers new insights into the importance of satellite data that can partially re-mediate statistical models' unsusceptibility to unusual events (like changes due to political intervention) with regard to model training.
更多查看译文
关键词
Nitrogen dioxides (NO2), TROPOspheric Monitoring Instrument (TROPOMI), Sentinel-5 precursor satellite, High resolution map of NO2, Machine learning, Extreme gradient boosting (XGBoost), Shapley additive exPlanations (SNAP), COVID-19 pandemic lockdown
AI 理解论文
溯源树
样例
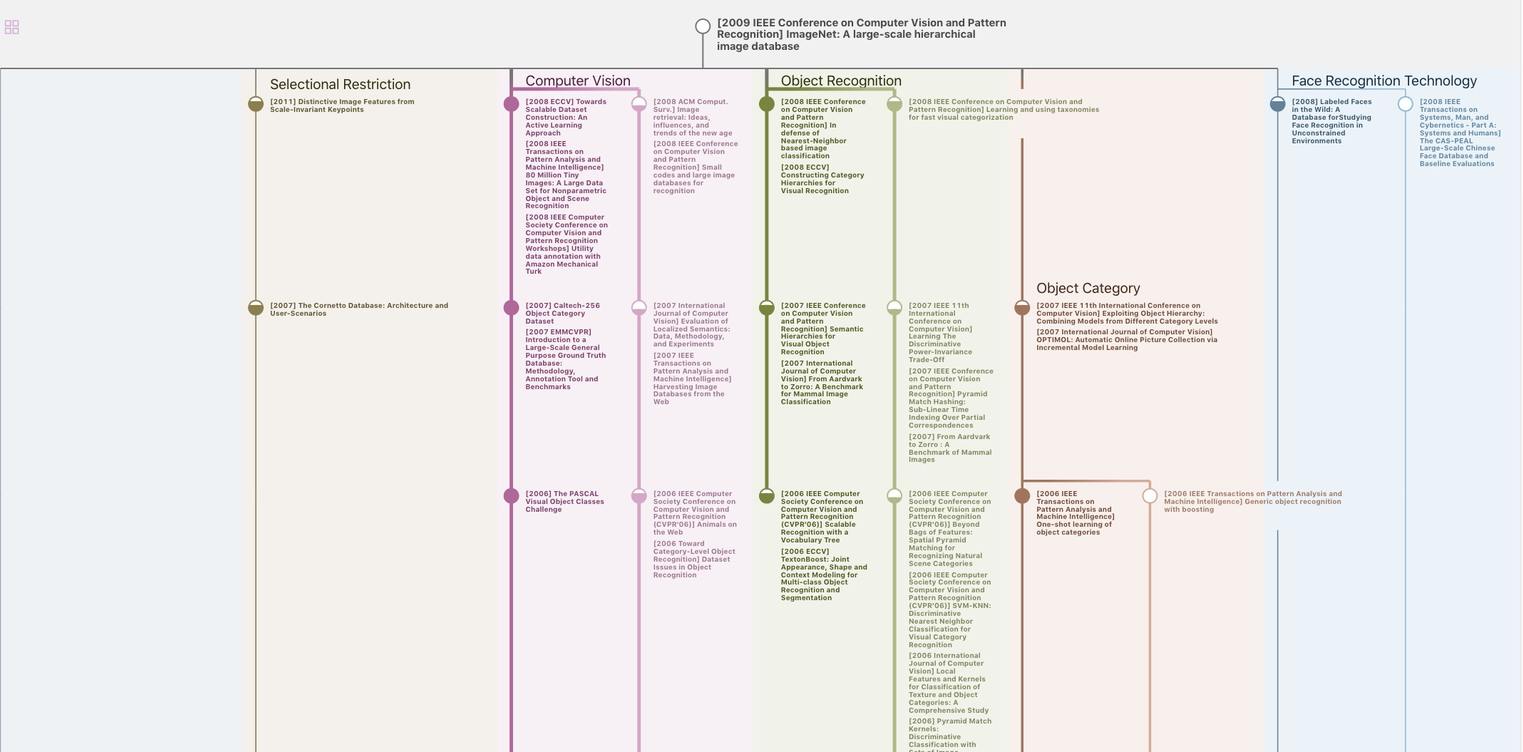
生成溯源树,研究论文发展脉络
Chat Paper
正在生成论文摘要