Machine Learning-Based Region Of Interest Detection In Airborne Lidar Fisheries Surveys
JOURNAL OF APPLIED REMOTE SENSING(2021)
Abstract
Airborne lidar data for fishery surveys often do not contain physics-based features that can be used to identify fish; consequently, the fish must be manually identified, which is a time-consuming process. To reduce the time required to identify fish, supervised machine learning was successfully applied to lidar data from fishery surveys to automate the process of identifying regions with a high probability of containing fish. Using data from Yellowstone Lake and the Gulf of Mexico, multiple experiments were run to simulate real-world scenarios. Although the human cannot be fully removed from the loop, the amount of data that would require manual inspection was reduced by 61.14% and 26.8% in the Yellowstone Lake and Gulf of Mexico datasets, respectively. (C) 2021 Society of Photo-Optical Instrumentation Engineers (SPIE)
MoreTranslated text
Key words
lidar, machine learning, fisheries, water
AI Read Science
Must-Reading Tree
Example
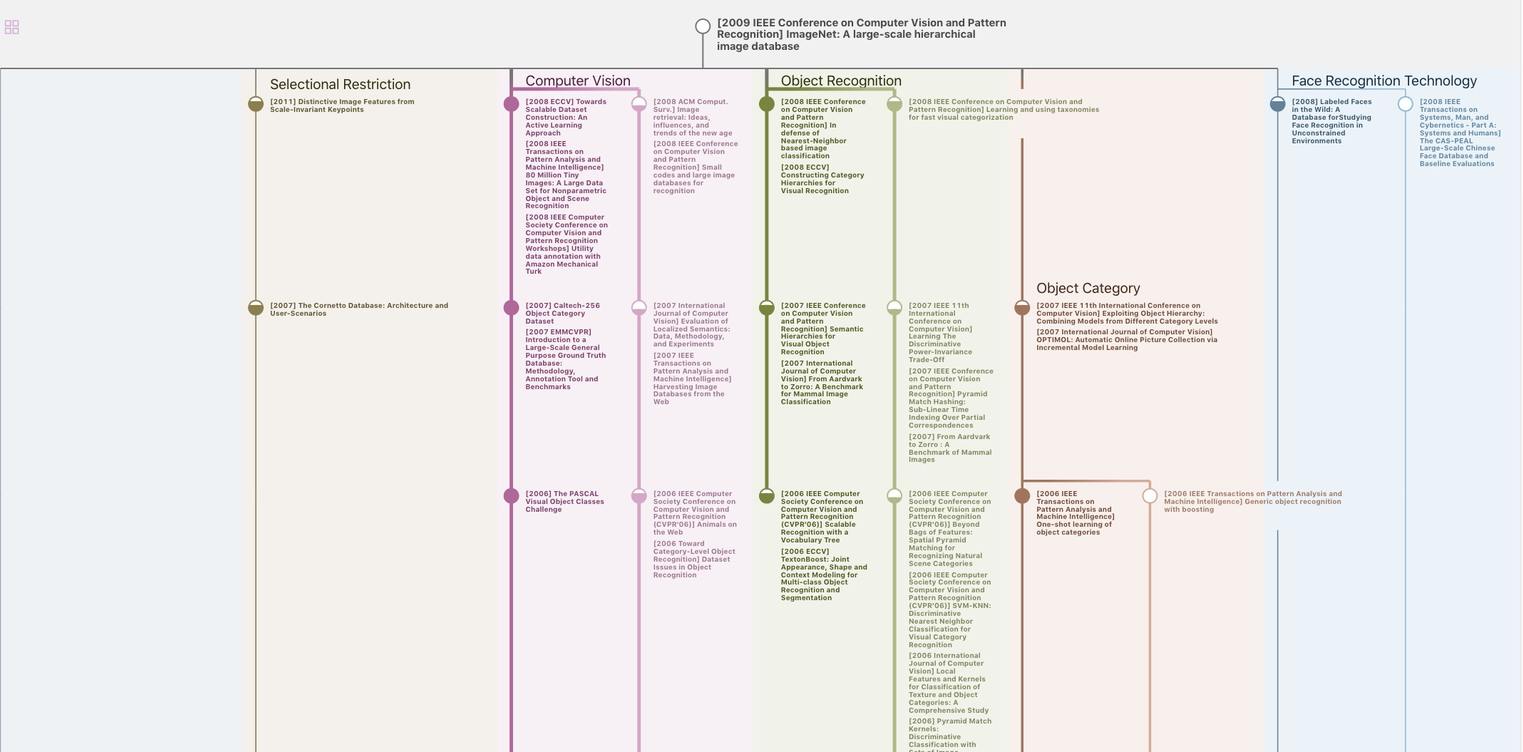
Generate MRT to find the research sequence of this paper
Chat Paper
Summary is being generated by the instructions you defined