The Application Of Machine Learning To Sensor Signals For Machine Tool And Process Health Assessment
PROCEEDINGS OF THE INSTITUTION OF MECHANICAL ENGINEERS PART B-JOURNAL OF ENGINEERING MANUFACTURE(2021)
摘要
Due to the latest advancements in monitoring technologies, interest in the possibility of early-detection of quality issues in components has grown considerably in the manufacturing industry. However, implementation of such techniques has been limited outside of the research environment due to the more demanding scenarios posed by production environments. This paper proposes a method of assessing the health of a machining process and the machine tool itself by applying a range of machine learning (ML) techniques to sensor data. The aim of this work is not to provide complete diagnosis of a condition, but to provide a rapid indication that the machine tool or process has changed beyond acceptable limits; making for a more realistic solution for production environments. Prior research by the authors found good visibility of simulated failure modes in a number of machining operations and machine tool fingerprint routines, through the defined sensor suite. The current research set out to utilise this system, and streamline the test procedure to obtain a large dataset to test ML techniques upon. Various supervised and unsupervised ML techniques were implemented using a range of features extracted from the raw sensor signals, principal component analysis and continuous wavelet transform. The latter were classified using convolutional neural networks (CNN); both custom-made networks, and pre-trained networks through transfer learning. The detection and classification accuracies of the simulated failure modes across all classical ML and CNN techniques tested were promising, with all approaching 100% under certain conditions.
更多查看译文
关键词
Digital manufacturing, optimisation, machine learning, machine tool, fault detection, fault classification, fingerprint routine, process monitoring, machining
AI 理解论文
溯源树
样例
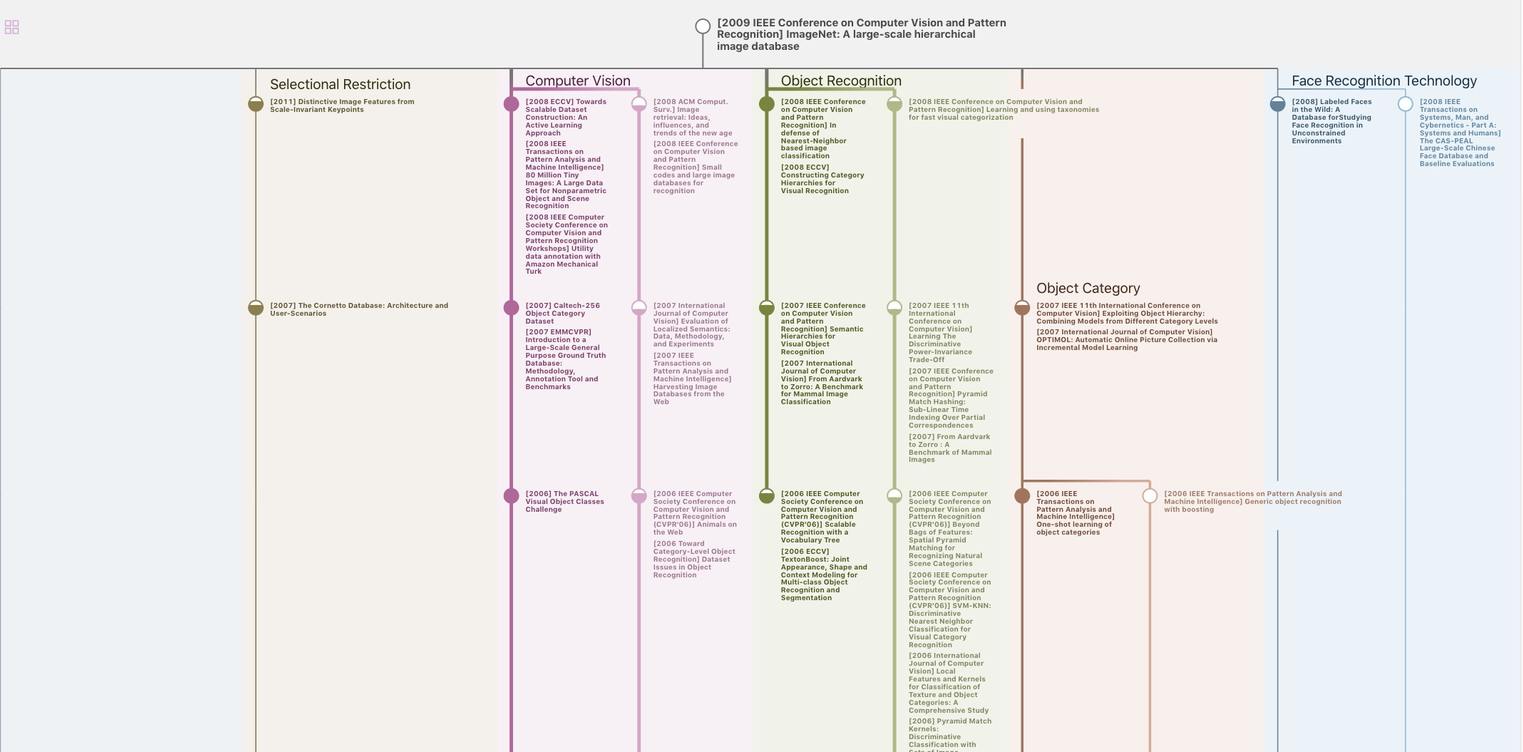
生成溯源树,研究论文发展脉络
Chat Paper
正在生成论文摘要