Group penalized quantile regression
Statistical Methods & Applications(2021)
Abstract
Quantile regression models have become a widely used statistical tool in genetics and in the omics fields because they can provide a rich description of the predictors’ effects on an outcome without imposing stringent parametric assumptions on the outcome-predictors relationship. This work considers the problem of selecting grouped variables in high-dimensional linear quantile regression models. We introduce a group penalized pseudo quantile regression (GPQR) framework with both group-lasso and group non-convex penalties. We approximate the quantile regression check function using a pseudo-quantile check function. Then, using the majorization–minimization principle, we derive a simple and computationally efficient group-wise descent algorithm to solve group penalized quantile regression. We establish the convergence rate property of our algorithm with the group-Lasso penalty and illustrate the GPQR approach performance using simulations in high-dimensional settings. Furthermore, we demonstrate the use of the GPQR method in a gene-based association analysis of data from the Alzheimer’s Disease Neuroimaging Initiative study and in an epigenetic analysis of DNA methylation data.
MoreTranslated text
Key words
Coordinate descent algorithm,Group penalized regression,Heterogeneous,Pseudo-quantile,Variable selection,Quantile regression
AI Read Science
Must-Reading Tree
Example
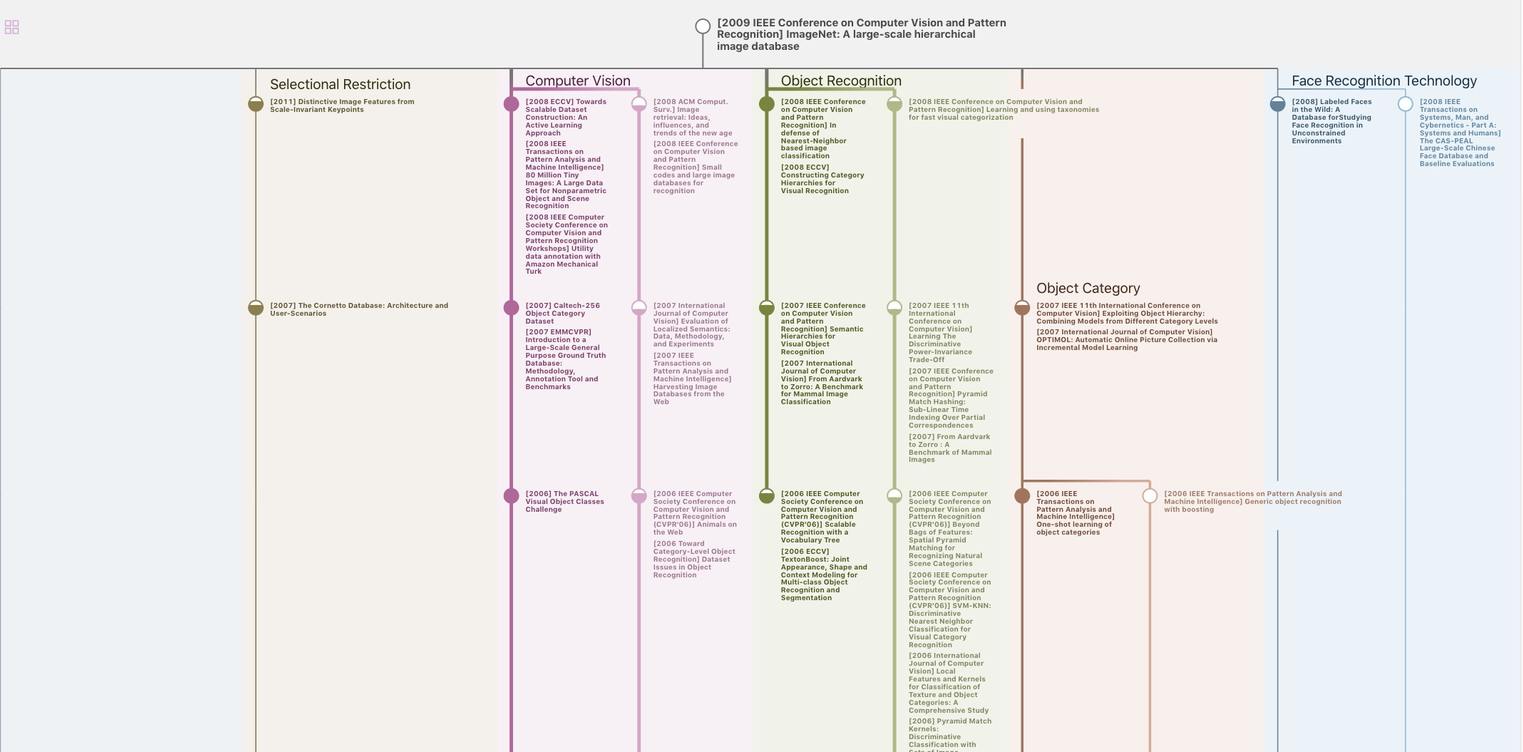
Generate MRT to find the research sequence of this paper
Chat Paper
Summary is being generated by the instructions you defined