Towards Better Explainable Deep Learning Models For Embryo Selection In Art
HUMAN REPRODUCTION(2021)
Abstract
Abstract Study question Can heatmaps generated by occlusion explain the patterns learned by deep learning (DL) models classifying the embryo viability in ART? Summary answer Occlusion experiments generate heatmaps that reveal which regions in frames of time-lapse video (TLV) are more discriminative for classification and prediction by the DL models. What is known already DL has widely been explored in ART for embryo selection. Depending upon input (video or image), different DL models classifying embryo viability are developed. However, whether the prediction is based on actual input features or random guessing is unknown. The embryo selection in ART is subjective. If the intention is using DL models’ prediction to transfer, freeze or discard the embryo, explanations of how they interpret embryonic development features brings transparency and trust. In other areas, heatmaps are used for explaining DL predictions. The heatmaps can be a tool to understand patterns learned by DL models for embryo selection. Study design, size, duration We trained two separate DL models for predicting the presence of fetal heartbeat for the transferred embryos. We further used occlusion generated heatmaps to explain the predictions. For training, retrospective data was used. The input dataset consisted of 136 TLVs and corresponding patient data for 132 participants (128: single embryo transfers and 8: double embryo transfer) from both IVF and ICSI treatment. Each video was assessed by an embryologist. Participants/materials, setting, methods DL models (A as ResNet–18, B as VGG16) are trained for predicting the presence of fetal heartbeat on a single frame extracted from TLV after day three or later. Model A has a better recall (0.7) compared to B (0.5). Heatmaps explain the reason behind models’ recall rate by visually representing patterns learned by them. Using occlusion filter size 30*30 with stride 14 and size 50*50 with stride 25, we generate heatmaps for both models. Main results and the role of chance The heatmaps generated using occlusion can represent visually the patterns discovered by the DL models when predicting the presence of a fetal heartbeat. Using occlusion filter size 30*30 with stride 14, we verified that Model B has lower recall because the heatmaps show that the model finds redundant features present outside the embryo region in many input frames. It could be interpreted that either the model has not learned relevant patterns or is more robust to noise. This representation of DL models equips us in better decision-making, whether to consider or discard the prediction or rather train the model further, preprocess training data or change network architecture. The heatmaps revealed that for frames where significant patterns learned by the models are within the embryo region, more weight was given to specific features like the inner cell mass, trophectoderm and some parts within the zona pellucida. Moreover, the heat maps generated using occlusion are independent of the underlying model’s architecture as the same experiment settings were used for both models. For occlusion filter size 50*50 with stride 25, the expanse of input regions (in or outside the embryo) considered relevant could be visualized for both models A and B. Limitations, reasons for caution Heatmaps generated by occluding input regions give a visual representation of features in individual frames not directly on videos. Explaining DL models by heatmaps besides occlusion, other techniques (Grad-Cam) exist but were not evaluated. Furthermore, there is no quantitative measure for evaluating whether heatmaps are a good explanation or not. Wider implications of the findings: The heatmaps make the patterns discovered by DL models visually recognized and bring forth the prominent portions of embryo regions. This will again improve understanding and trust in DL models’ predictions. Visual representation of DL models using heatmaps enables interpreting a prediction, performing model analysis and determining scope for improvement. Trial registration number Not applicable
MoreTranslated text
Key words
explainable deep learning models,embryo selection,deep learning models
AI Read Science
Must-Reading Tree
Example
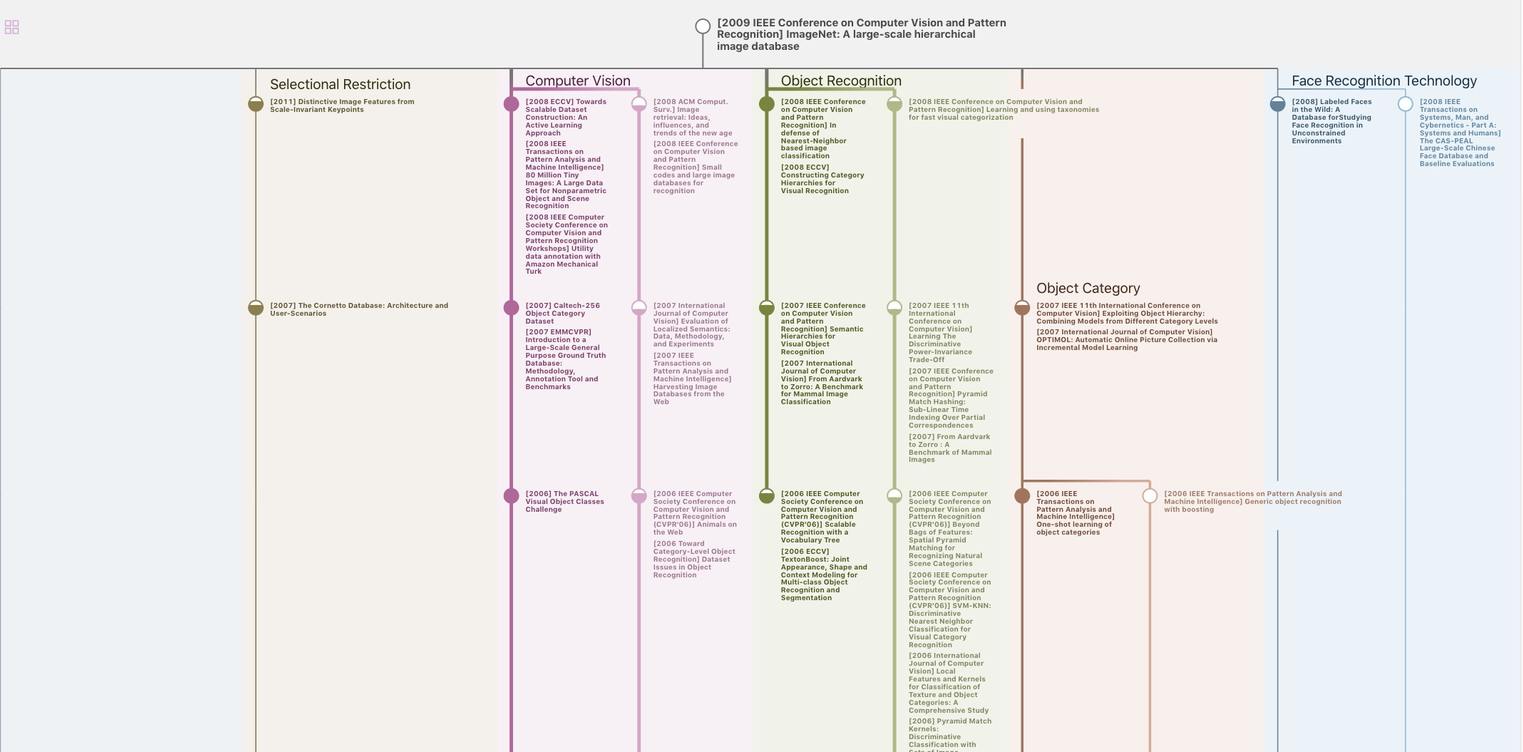
Generate MRT to find the research sequence of this paper
Chat Paper
Summary is being generated by the instructions you defined