Keypoint Matching For Point Cloud Registration Using Multiplex Dynamic Graph Attention Networks
IEEE ROBOTICS AND AUTOMATION LETTERS(2021)
摘要
The registration of point clouds is a key ingredient of LiDAR-based SLAM systems and mapping approaches. A challenging task in this context is finding the right data association between 3D points. This paper proposes a novel and flexible graph network architecture to tackle the keypoint matching problem in an end-to-end fashion. Each layer of our multiplex dynamic graph attention network (MDGAT) utilizes an attention mechanism to dynamically construct a multiplex graph and reasons about the contextual information based on the point cloud data. It enriches the feature representation by recovering local information and by aggregating information along with the connections. We also design a scan matcher called MDGAT-matcher, which treats the registration problem as an optimal transport problem and uses the predictions of MDGAT as the cost. It builds upon sparse keypoints extracted from pairs of LiDAR scans. Eventually, MDGAT-matcher finds high-quality correspondences and at the same time handles non-matching points appropriately. We train our matcher using a novel gap loss guiding the network to learn a discriminative cognition about matching and non-matching 3D points. We thoroughly test our approach on the KITTI odometry benchmark. The experiments presented in this paper suggest that our approach outperforms state-of-the-art matching approaches and achieves a substantial improvement.
更多查看译文
关键词
SLAM, deep learning metod
AI 理解论文
溯源树
样例
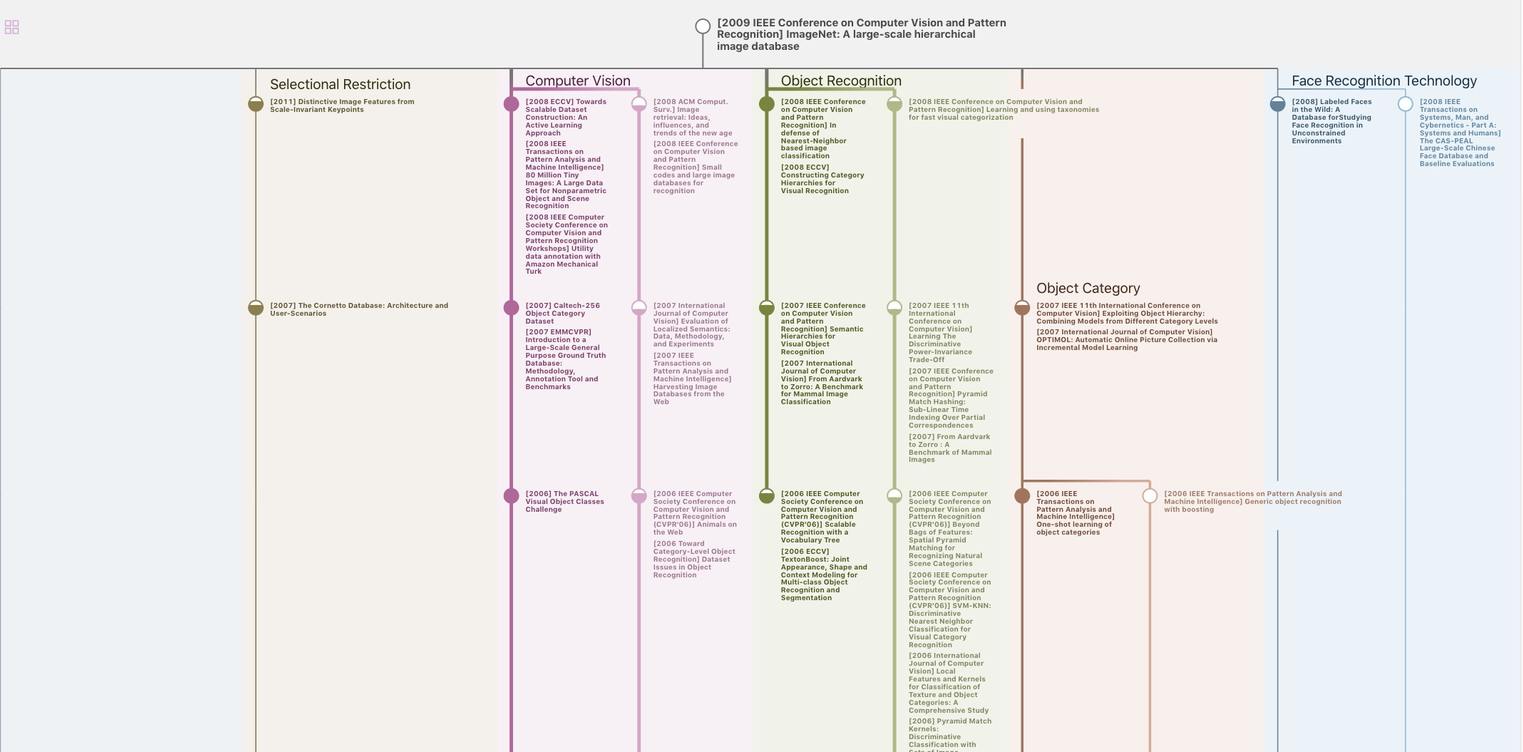
生成溯源树,研究论文发展脉络
Chat Paper
正在生成论文摘要