Transfer Learning-Based Synthetic Ct Generation For Mr-Only Proton Therapy Planning In Children With Pelvic Sarcomas
MEDICAL IMAGING 2021: PHYSICS OF MEDICAL IMAGING(2021)
Abstract
Proton therapy planning requires Hounsfield unit (HU) data from CT images to calculate dose and, in the case of pelvic sarcomas, accurate registration of the CT to MRI to delineate tumor. MR-only proton therapy planning would eliminate the uncertainty associated with CT/MR image registration and the need for CT, reducing exposure to radiation and anesthesia in children. We determined whether MR-only proton therapy planning is feasible by introducing a transfer learning based cycleGAN (TL-cycleGAN) method to convert pelvic MRI to synthetic CT (sCT) for dose calculation and to specifically address the challenge of a small training dataset, commonly associated with pediatric studies. The TL-cycleGAN was designed to transfer knowledge gained from converting a large number (n=125) of pediatric brain MRI studies to sCT and finetune the well-trained model on pelvic data. Sixteen patients (aged 1.1-21.3 years, 7 females) who received proton therapy to the pelvis were randomly divided into training (n=11) and testing (n=5) groups. sCT generated from T1- T2-weighted fat suppression MR images was compared to the real CT in terms of peak signal-to-noise ratio (PSNR), structural similarity (SSIM) index, mean error (ME), and mean absolute error (MAE) in HU. The mean +/- standard deviation of PSNR, SSIM, ME and MAE were 30.6 +/- 3.0, 0.93 +/- 0.04 -3.4 +/- 10.2 HU, and 52.4 +/- 17.6 HU, respectively. for TIW MRI; 29.2 +/- 1.5, 0.93 +/- 0.02, -6.6 +/- 24.8 HU, and 85.4 +/- 18.8 HU, respectively, for T2W MR1. Transfer learning facilitates MR-only pediatric pelvic proton therapy planning by generating highly accurate sCT for a small training dataset and a large variation.
MoreTranslated text
Key words
synthetic CT, transfer learning, proton therapy treatment, pediatric patient, deep learning, Cycle generative adversarial network
AI Read Science
Must-Reading Tree
Example
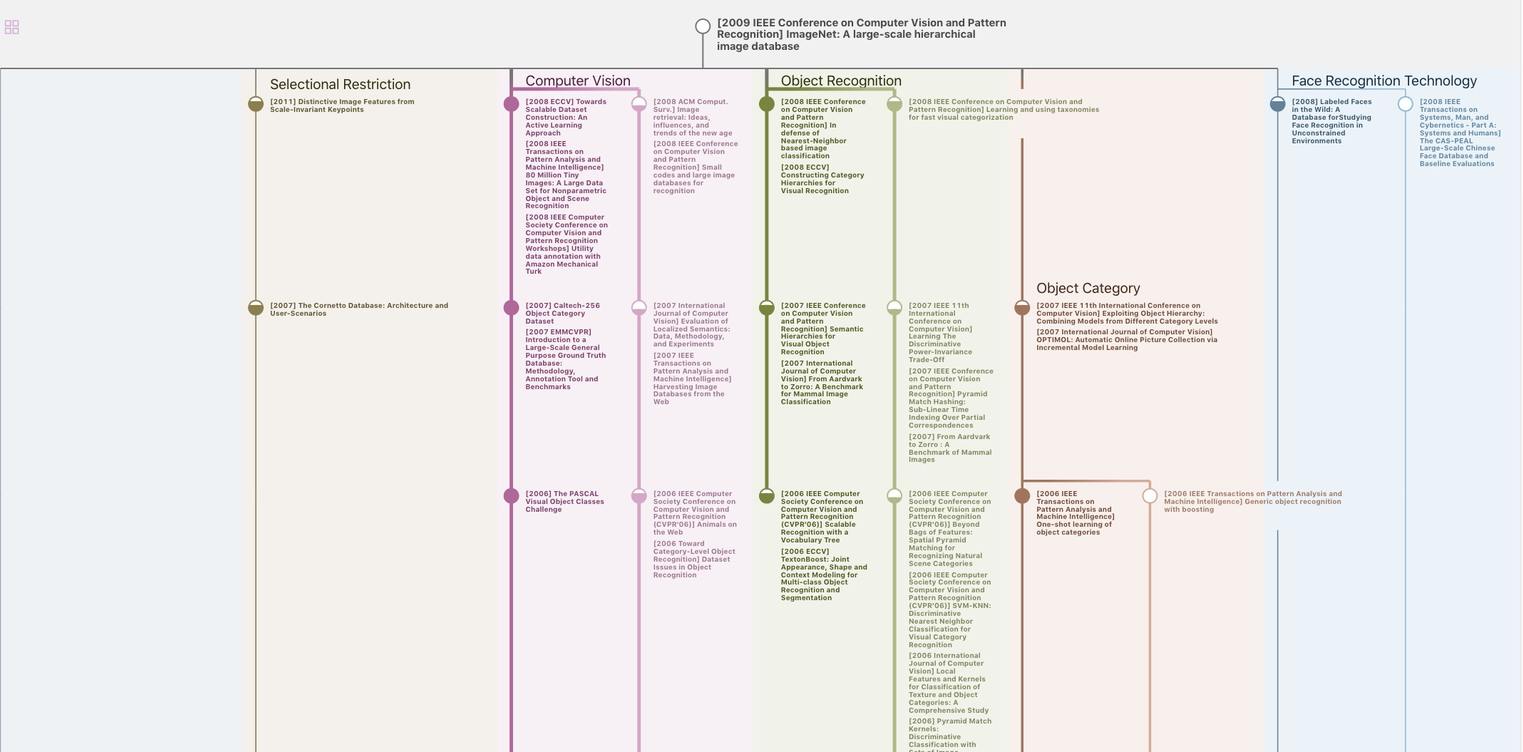
Generate MRT to find the research sequence of this paper
Chat Paper
Summary is being generated by the instructions you defined