Privacy-Preserving Image Classification With Deep Learning and Double Random Phase Encoding
IEEE ACCESS(2021)
摘要
With the emergence of cloud computing, large amounts of private data are stored and processed in the cloud. On the other hand, data owners (users) may not want to reveal data information to cloud providers to protect their privacy. Therefore, users may upload encrypted data to the cloud or third-party platforms, such as Google Cloud, Amazon Web Service, and Microsoft Azure. Conventionally, data must be decrypted before being analyzed in the cloud, which raises privacy concerns. Moreover, decryption of big data such as images requires enormous computation resources, which is unsuitable for energy-constrained devices, particularly Internet of Things (IoT) devices. Data privacy in most popular applications, such as image query or classification, can be preserved if encrypted images can be directly classified on the cloud or IoT devices without decryption. This paper proposes a high-speed double random phase encoding (DRPE) technique of encrypting images into white-noise images. DRPE-encrypted images are then uploaded and stored in the cloud. Images that are encrypted without being decrypted are classified using deep convolutional neural networks in the cloud. The simulation results indicated the feasibility and good performance of the proposed approach. The proposed privacy-preserving image classification method can be useful in data-sensitive fields, such as medicine and transportation.
更多查看译文
关键词
Cloud computing,Encryption,Image classification,Classification algorithms,Feature extraction,Data models,Training,Double random phase encoding,information security,image encryption,deep learning,privacy preserving
AI 理解论文
溯源树
样例
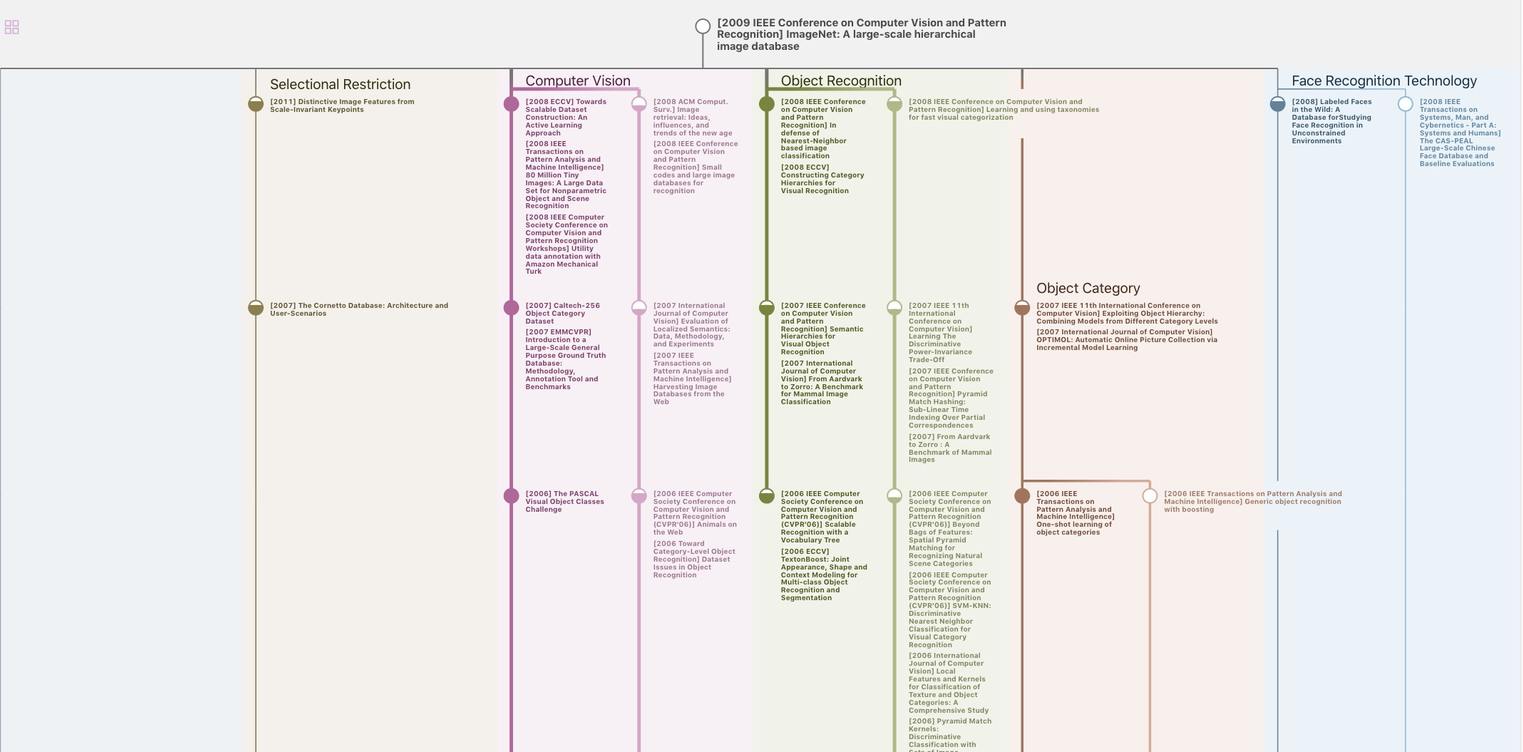
生成溯源树,研究论文发展脉络
Chat Paper
正在生成论文摘要