SRNet: Scale-Aware Representation Learning Network for Dense Crowd Counting
IEEE ACCESS(2021)
Abstract
Huge variations in the scales of people in images create an extremely challenging problem in the task of crowd counting. Currently, many researchers apply multi-column structures to solve the scale variation problem. However, multi-column structures usually have complex structures with large numbers of parameters and are difficult to optimize. To this end, we propose a scale-aware representation learning network (SRNet) that uses a commonly used encoder-decoder framework. An image is converted into deep features by the first ten layers of VGG16 in the encoder. Then, the features are regressed to a crowd density map via the decoder. The decoder mainly consists of two modules: the scale-aware feature learning module (SAM) and the pixel-aware upsampling module (PAM). SAM models the multi-scale features of a crowd at each level with different sizes of receptive fields, and PAM enlarges the spatial resolution and enhances the pixel-level semantic information, thereby improving the overall counting accuracy. We conduct extensive crowd counting experiments on ShanghaiTech Part_A, UCF-QNRF, and UCF_CC_50 datasets. Furthermore, to obtain the locations of each person, we conduct crowd localization experiments on UCF-QNRF and NWPU-Crowd datasets. The qualitative and quantitative results prove the effectiveness of the SRNet in dense crowd counting and crowd localization tasks.
MoreTranslated text
Key words
Feature extraction,Task analysis,Convolution,Estimation,Decoding,Semantics,Location awareness,Dense crowd counting,multi-scale feature learning,deep learning,convolution neural network
AI Read Science
Must-Reading Tree
Example
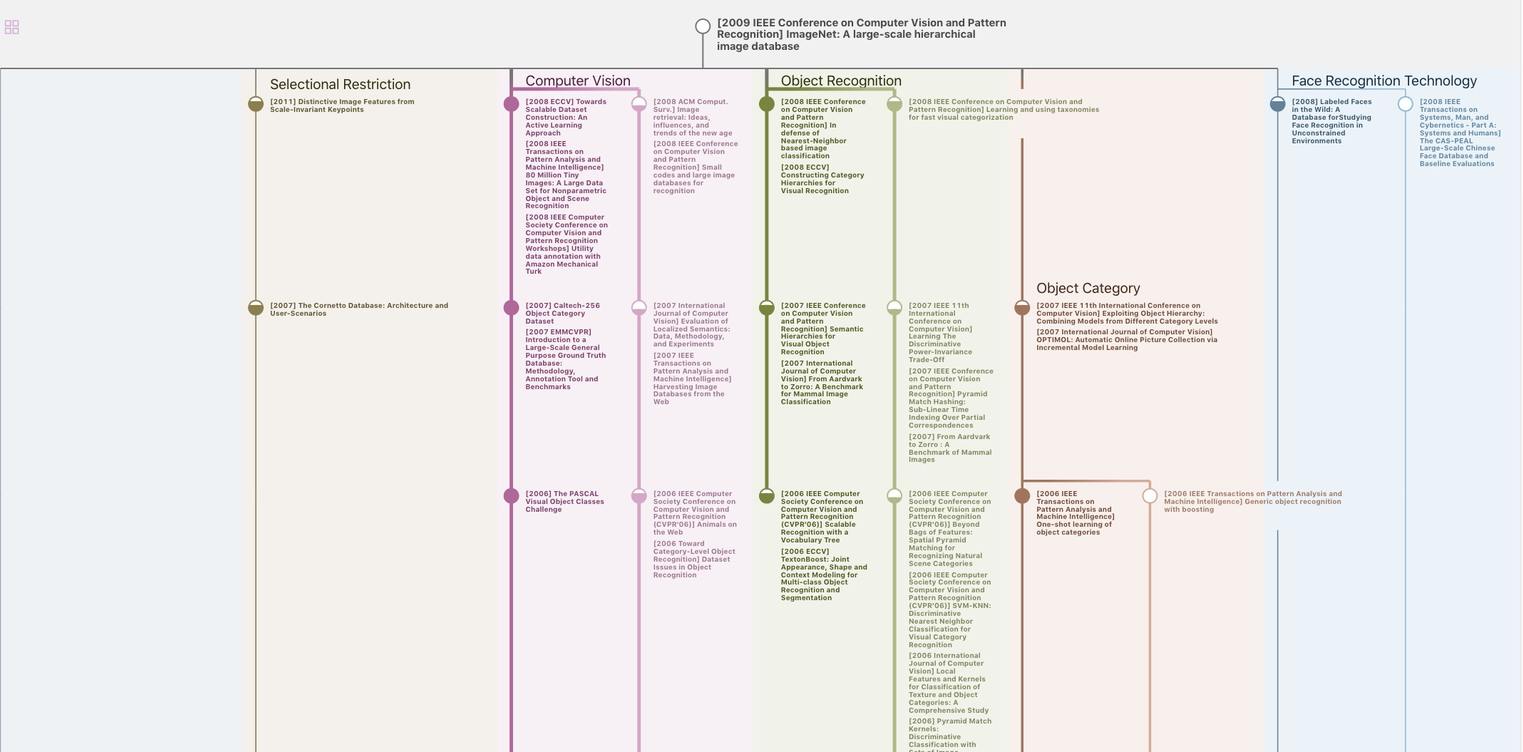
Generate MRT to find the research sequence of this paper
Chat Paper
Summary is being generated by the instructions you defined