Entropy-Based Time Window Features Extraction For Machine Learning To Predict Acute Kidney Injury In Icu
APPLIED SCIENCES-BASEL(2021)
摘要
Featured Application The proposed method has been applied and landed in the ICU at Taichung Veterans General Hospital since 2021. Acute kidney injury (AKI) refers to rapid decline of kidney function and is manifested by decreasing urine output or abnormal blood test (elevated serum creatinine). Electronic health records (EHRs) is fundamental for clinicians and machine learning algorithms to predict the clinical outcome of patients in the Intensive Care Unit (ICU). Early prediction of AKI could automatically warn the clinicians to review the possible risk factors and act in advance to prevent it. However, the enormous amount of patient data usually consists of a relatively incomplete data set and is very challenging for supervised machine learning process. In this paper, we propose an entropy-based feature engineering framework for vital signs based on their frequency of records. In particular, we address the missing at random (MAR) and missing not at random (MNAR) types of missing data according to different clinical scenarios. Regarding its applicability, we applied it to establish a prediction model for future AKI in ICU patients using 4278 ICU admissions from a tertiary hospital. Our result shows that the proposed entropy-based features are feasible to be used in the AKI prediction model and its performance improves as the data availability increases. In addition, we study the performance of AKI prediction model by comparing different time gaps and feature windows with the proposed vital sign entropy features. This work could be used as a guidance for feature windows selection and missing data processing during the development of a prediction model in ICU.
更多查看译文
关键词
acute kidney injury (AKI), machine learning, entropy
AI 理解论文
溯源树
样例
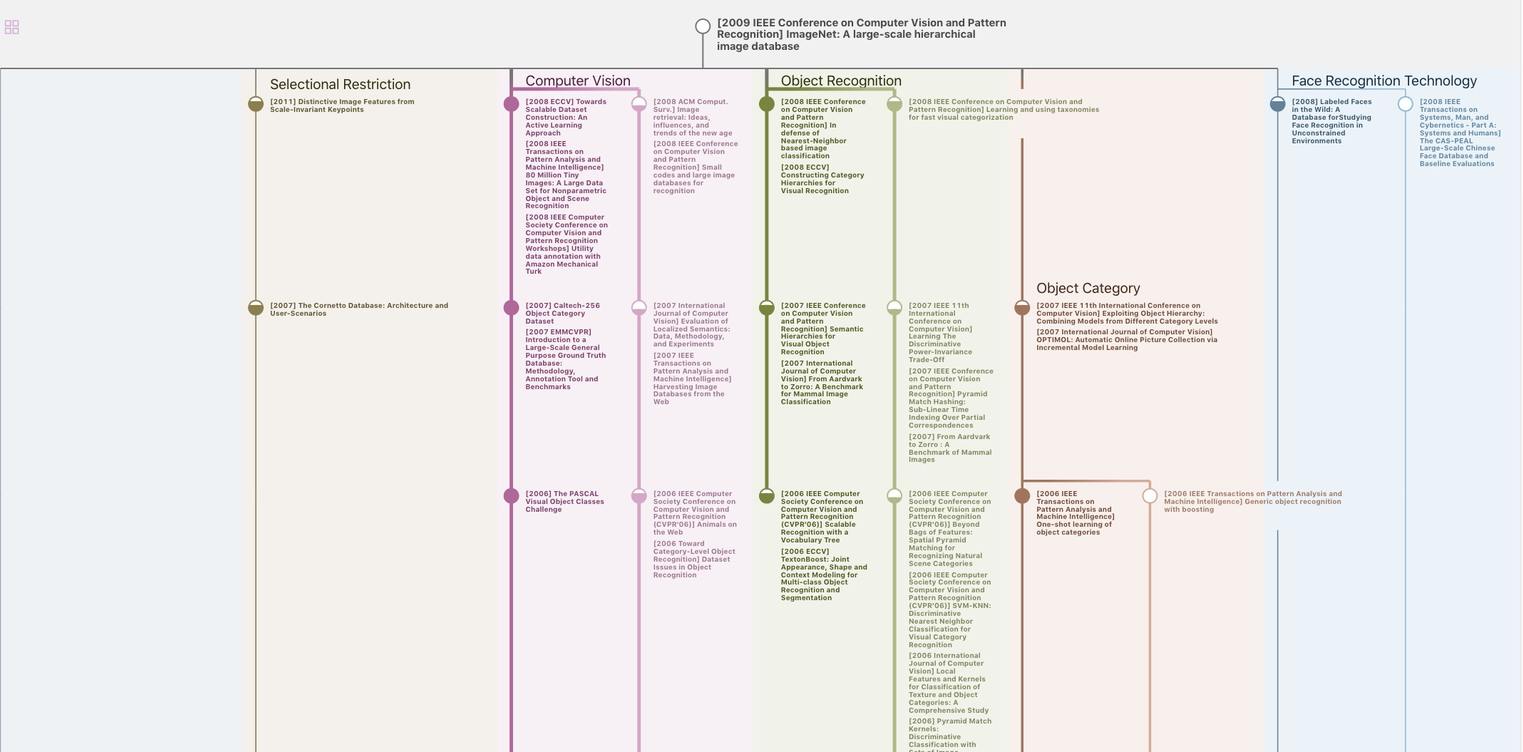
生成溯源树,研究论文发展脉络
Chat Paper
正在生成论文摘要