Interacting Multiple Model Strategy For Electric Vehicle Batteries State Of Charge/Health/ Power Estimation
IEEE ACCESS(2021)
摘要
States estimation of lithium-ion batteries is an essential element of Battery Management Systems (BMS) to meet the safety and performance requirements of electric and hybrid vehicles. Accurate estimations of the battery's State of Charge (SoC), State of Health (SoH), and State of Power (SoP) are essential for safe and effective operation of the vehicle. They need to remain accurate despite the changing characteristics of the battery as it ages. This paper proposes an online adaptive strategy for high accuracy estimation of SoC, SoH and SoP to be implemented onboard of a BMS. A third-order equivalent circuit model structure is considered with its state vector augmented with two more variables for estimation including the internal resistance and SoC bias. An Interacting Multiple Model (IMM) strategy with a Smooth Variable Structure Filter (SVSF) is then employed to determine the SoC, internal resistance, and SoC bias of a battery. The IMM strategy results in the generation of a mode probability that is related to battery aging. This mode probability is then combined with an estimation of the battery's internal resistance to determine the SoH. The estimated internal resistance and the SoC are then used to determine the battery SoP which provides a complete estimation of the battery states of operation and condition. The efficacy of the proposed condition-monitoring strategy is tested and validated using experimental data obtained from accelerated aging tests conducted on Lithium Polymer automotive battery cells.
更多查看译文
关键词
Batteries, Estimation, Battery charge measurement, Resistance, Integrated circuit modeling, Adaptation models, Temperature measurement, Lithium-ion battery, battery management system, interacting multiple model, smooth variable structure filter, state of charge, state of health, state of power
AI 理解论文
溯源树
样例
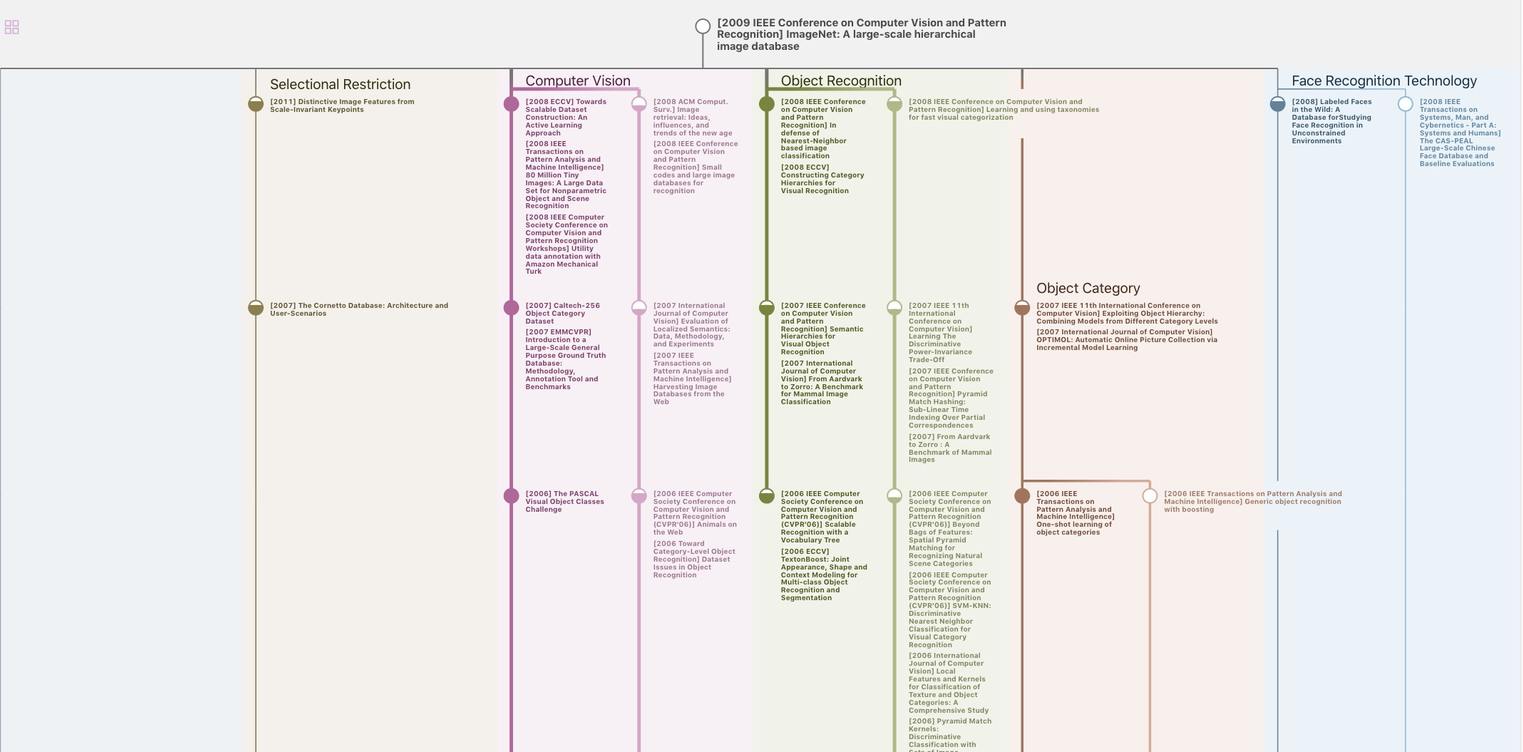
生成溯源树,研究论文发展脉络
Chat Paper
正在生成论文摘要