Moving Target Classification Based On Micro-Doppler Signatures Via Deep Learning
2021 IEEE RADAR CONFERENCE (RADARCONF21): RADAR ON THE MOVE(2021)
摘要
Radar-based classification of ground moving targets relies on Doppler information. Therefore, the classification between humans and animals is a challenging task due to their similar Doppler signatures. This work proposes a Deep Learning-based approach for ground-moving radar targets classification. The proposed algorithm learns the radar targets' micro-Doppler signatures in the 2D fast-time slow-time radar echoes domain. This work shows that the convolutional neural network (CNN) can achieve high classification performance. Also, it shows that efficient data augmentation and regularization significantly improve classification performance and reduce over-fit.
更多查看译文
关键词
ground-moving radar,radar targets,microDoppler signatures,2D fast-time slow-time radar echoes domain,high classification performance,target classification,radar-based classification,ground moving targets,Doppler information,similar Doppler signatures,Deep Learning-based approach
AI 理解论文
溯源树
样例
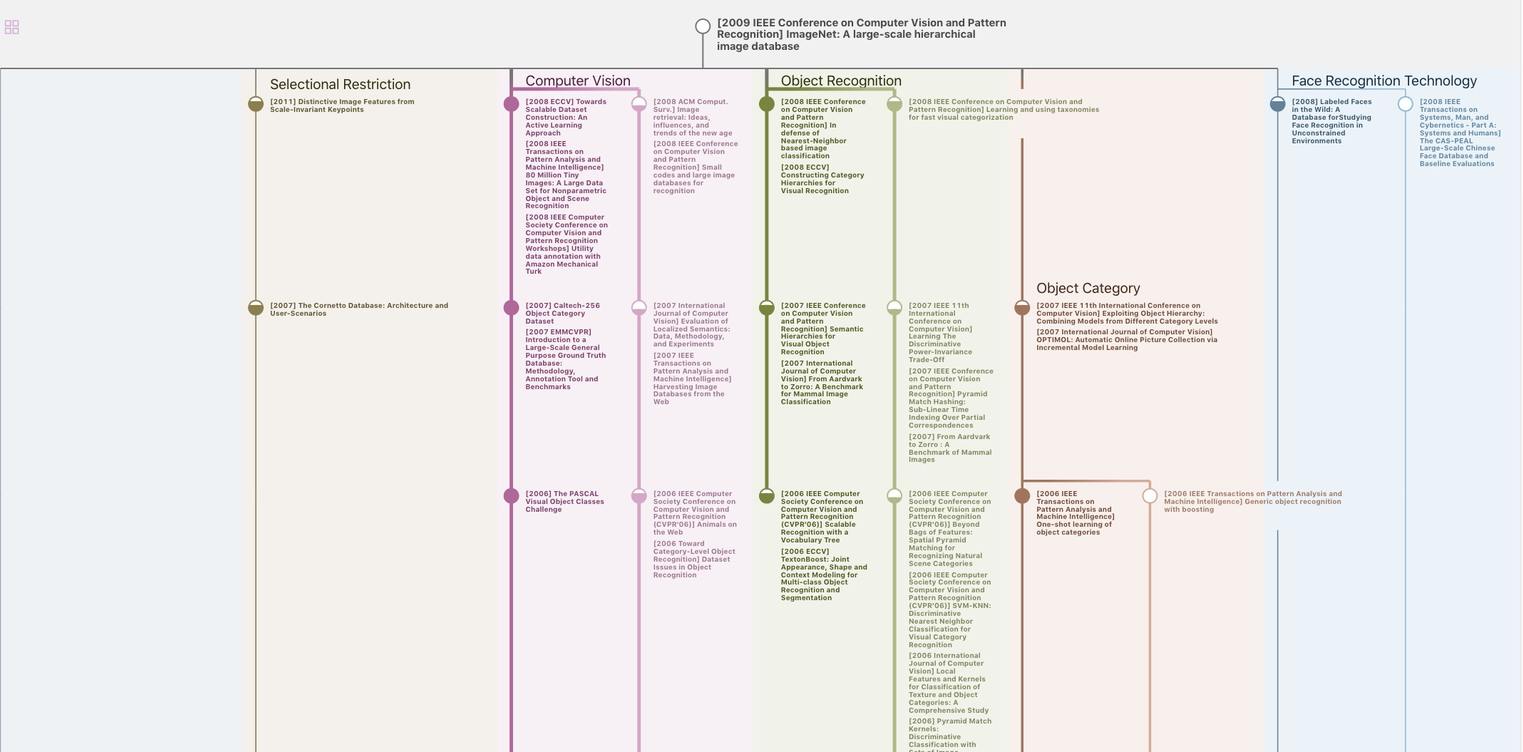
生成溯源树,研究论文发展脉络
Chat Paper
正在生成论文摘要