Interpretable Machine Learning for Texture-Dependent Constitutive Models with Automatic Code Generation for Topological Optimization
INTEGRATING MATERIALS AND MANUFACTURING INNOVATION(2021)
摘要
Genetic programming-based symbolic regression (GPSR) is a machine learning method which produces symbolic models that can be readily interpreted. This study utilized GPSR to derive uniaxial texture-based constitutive models for an additively manufactured alloy which were evaluated in post hoc analyses. Training data consisted of microscopy and mechanical testing data provided by the Air Force Research Laboratory (AFRL) which was supplemented using a viscoplastic model calibrated to the observed data. The validity of the calibrated crystal plasticity viscoplastic model is demonstrated as part of the 2019 AFRL Additive Manufacturing Modeling Challenge Series. Additionally, an expression evaluator was developed to integrate the constitutive models into the topology optimization software package Plato. A significant aspect of this paper is the presentation of these topics as components within a highly automated framework that allows efficient incorporation of microstructural characteristics into design activities. A topology optimization example was conducted using the GPSR results that constitutes application of the automated framework and post hoc analyses of the GPSR models demonstrate interpretability, suitability, and a probabilistic method to quantify domain bounds.
更多查看译文
关键词
Symbolic regression, Constitutive model, Texture, Topology optimization, Viscoplastic, Parameter homogenization
AI 理解论文
溯源树
样例
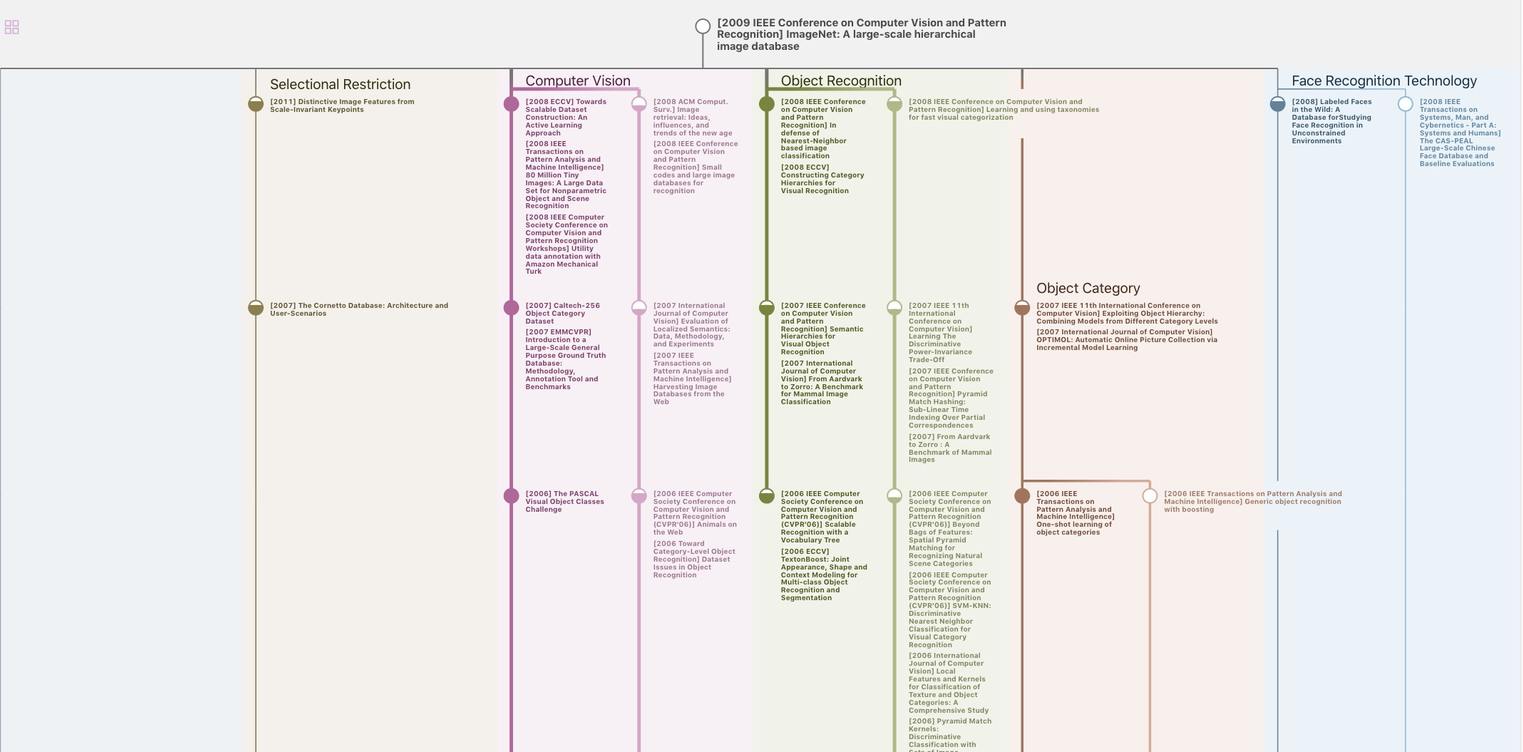
生成溯源树,研究论文发展脉络
Chat Paper
正在生成论文摘要