Combination Of Polynomial Chaos And Kriging For Reduced-Order Model Of Reacting Flow Applications
RESULTS IN ENGINEERING(2021)
摘要
The combination of Proper Orthogonal Decomposition (POD) with Kriging has been shown to be a reliable choice for the development of Reduced-Order Models (ROMs) for the prediction of combustion data at unexplored operating conditions. In this study, POD is combined with Polynomial Chaos Expansion (PCE), with a combination of PCE and Kriging (PC-Kriging) and with Artificial Neural Networks (ANN) for the development of a ROM that can predict 2D combustion data for unexplored operating conditions. The choice of Non-negative Matrix Factorization (NMF) instead of POD as compression method is also investigated. This method is chosen because it can intrinsically guarantee the non-violation of physical constraints such as positivity of chemical species mass fractions, although POD'S data reconstruction errors are lower. The performances of the POD and NMF in combination with the proposed supervised methods are compared, with prediction normalized root mean squared errors (NRMSE) being less than 10% for spatial fields of temperature, CH4 and O-2 for all approaches.
更多查看译文
关键词
PCA, Surrogate models, Polynomial chaos, Kriging
AI 理解论文
溯源树
样例
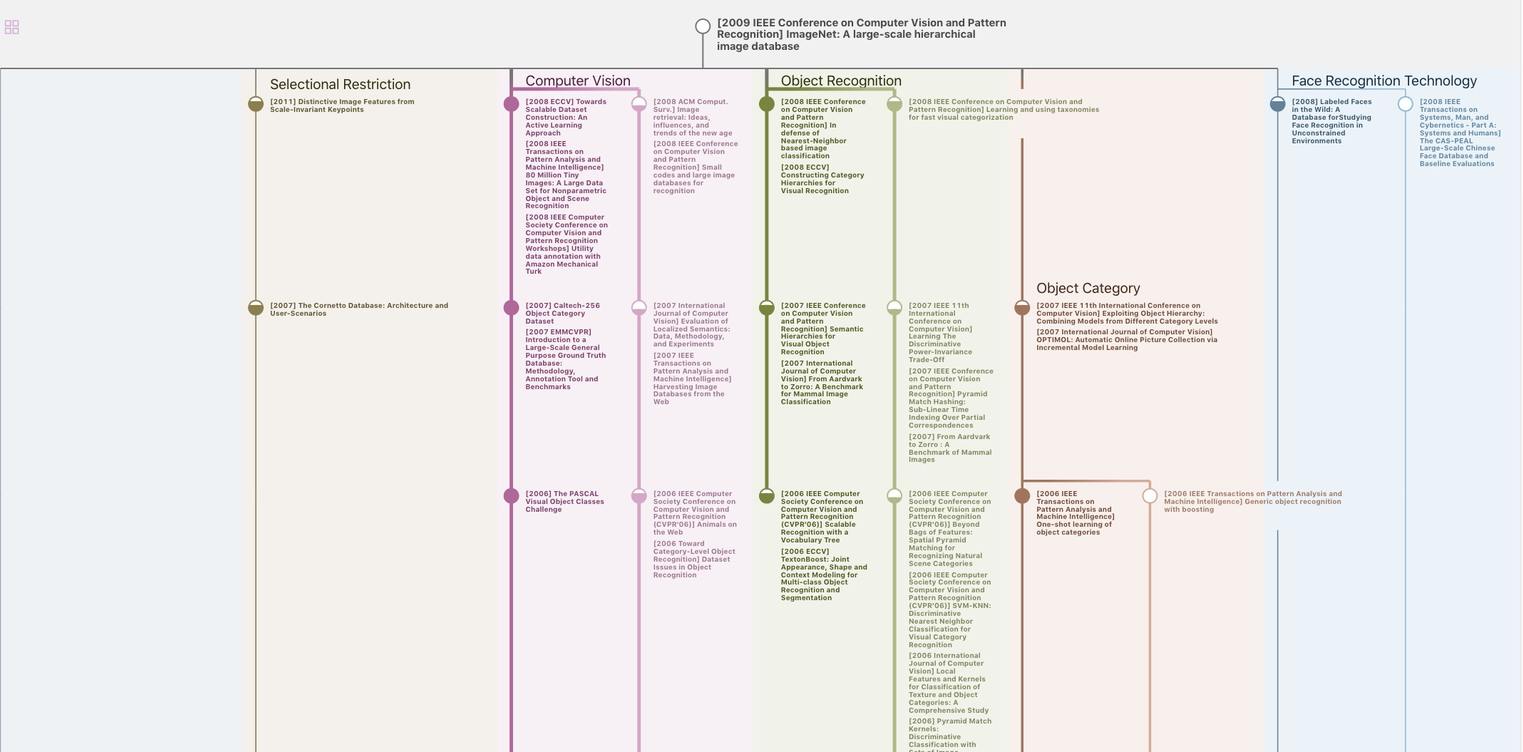
生成溯源树,研究论文发展脉络
Chat Paper
正在生成论文摘要