Large spatial data modeling and analysis: A Krylov subspace approach
SCANDINAVIAN JOURNAL OF STATISTICS(2022)
Abstract
Estimating the parameters of spatial models for large spatial datasets can be computationally challenging, as it involves repeated evaluation of sizable spatial covariance matrices. In this paper, we aim to develop Krylov subspace-based methods that are computationally efficient for large spatial data. Specifically, we approximate the inverse and the log-determinant of the spatial covariance matrix in the log-likelihood function via conjugate gradient and stochastic Lanczos on a Krylov subspace. These methods reduce the computational complexity from O(N3) to O(N2logN) and O(NlogN) for dense and sparse matrices, respectively. Moreover, we quantify the difference between the approximated log-likelihood function and the original log-likelihood function and establish the consistency of parameter estimates. Simulation studies are conducted to examine the computational efficiency as well as the finite-sample properties. For illustration, our methodology is applied to analyze a large dataset comprising LiDAR estimates of forest canopy height in western Alaska.
MoreTranslated text
Key words
computational efficiency,conjugate gradient,maximum likelihood estimation,spatial linear models,spatial statistics
AI Read Science
Must-Reading Tree
Example
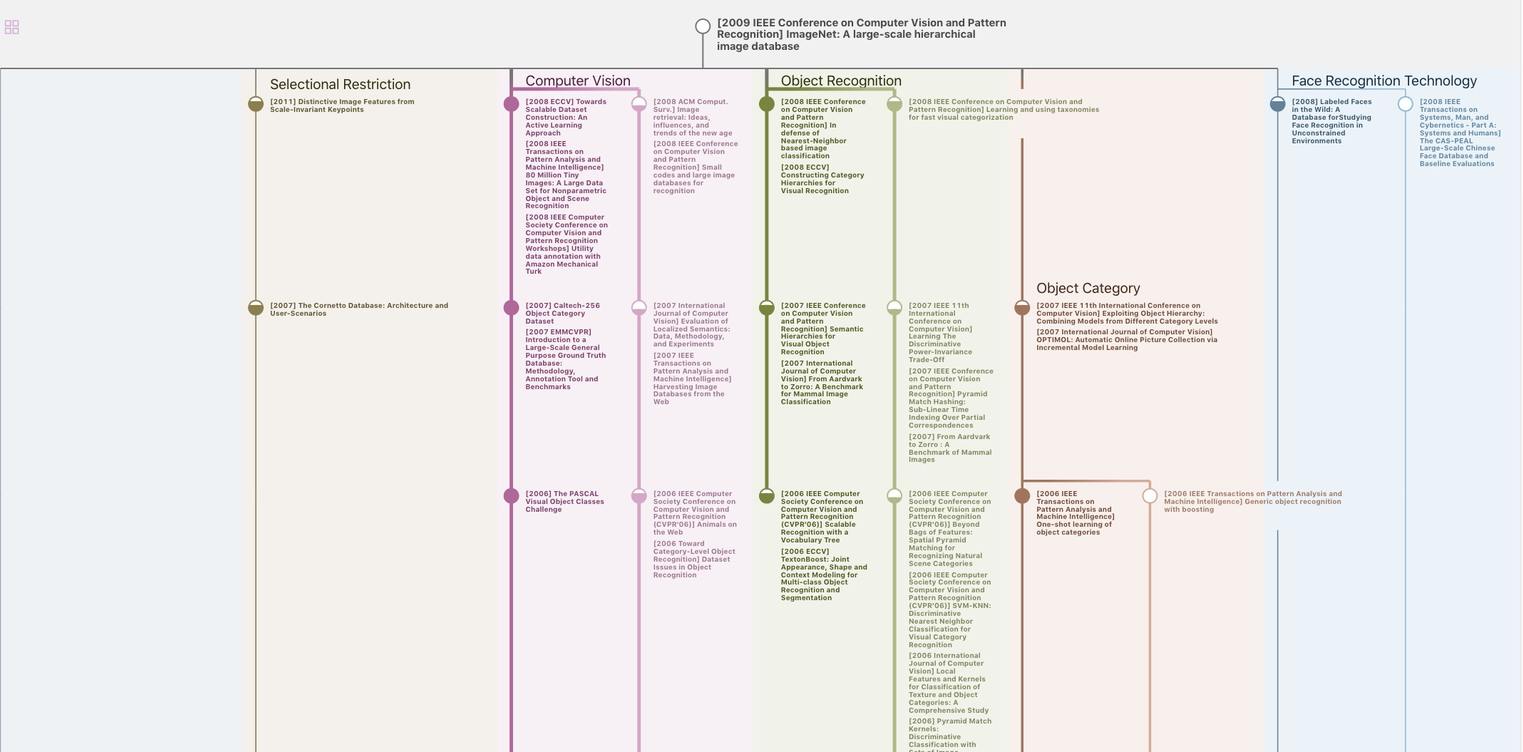
Generate MRT to find the research sequence of this paper
Chat Paper
Summary is being generated by the instructions you defined