Zernike Coefficient Prediction Technique for Interference Based on Generation Adversarial Network
APPLIED SCIENCES-BASEL(2021)
Abstract
In the paper, we propose a novel prediction technique to predict Zernike coefficients from interference fringes based on Generative Adversarial Network (GAN). In general, the task of GAN is image-to-image translation, but we design GAN for image-to-number translation. In the GAN model, the Generator's input is the interference fringe image, and its output is a mosaic image. Moreover, each piece of the mosaic image links to the number of Zernike coefficients. Root Mean Square Error (RMSE) is our criterion for quantifying the ground truth and prediction coefficients. After training the GAN model, we use two different methods: the formula (ideal images) and optics simulation (simulated images) to estimate the GAN model. As a result, the RMSE is about 0.0182 +/- 0.0035 lambda with the ideal image case and the RMSE is about 0.101 +/- 0.0263 lambda with the simulated image case. Since the outcome in the simulated image case is poor, we use the transfer learning method to improve the RMSE to about 0.0586 +/- 0.0035 lambda. The prediction technique applies not only to the ideal case but also to the actual interferometer. In addition, the novel prediction technique makes predicting Zernike coefficients more accurate than our previous research.
MoreTranslated text
Key words
generation adversarial network,interference fringe,Zernike coefficient,transfer learning
AI Read Science
Must-Reading Tree
Example
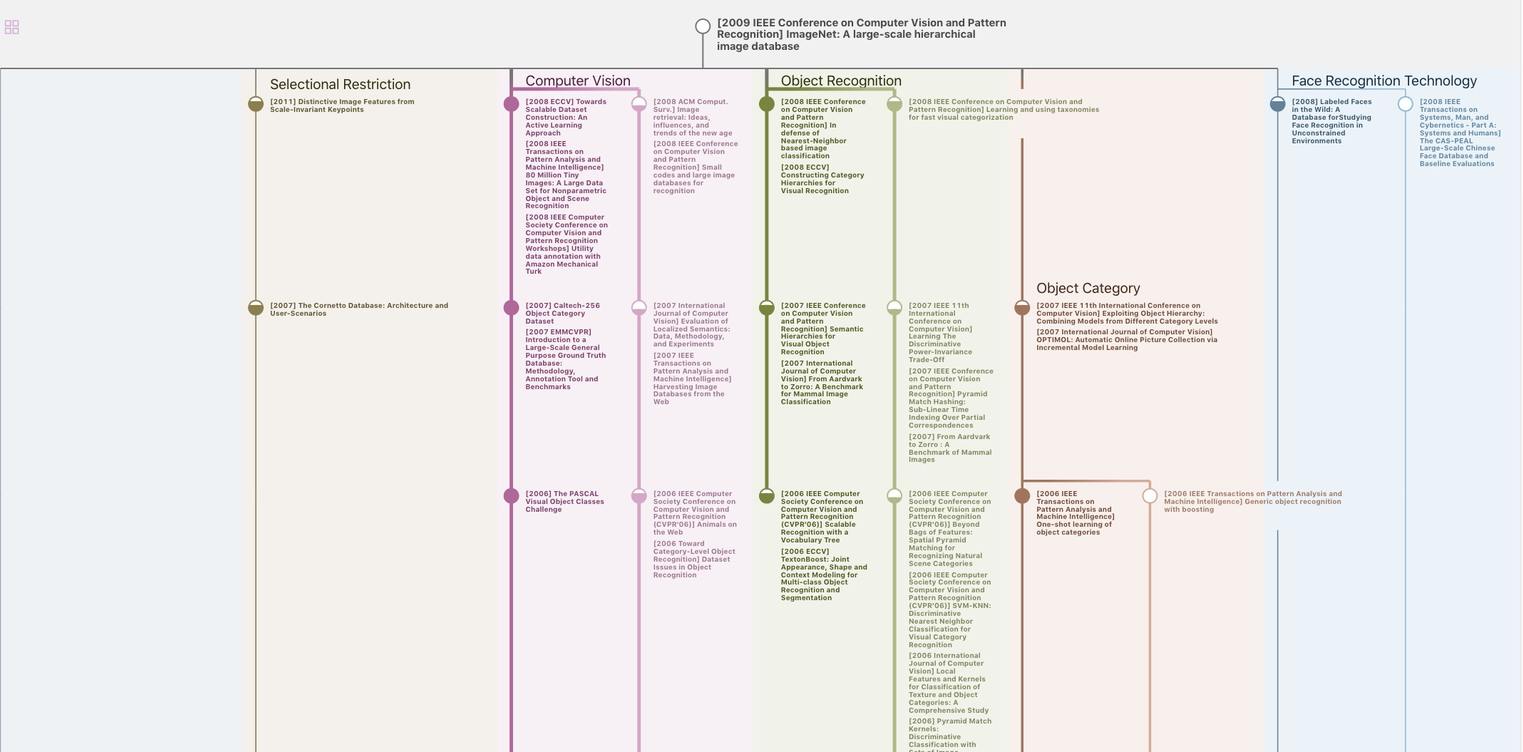
Generate MRT to find the research sequence of this paper
Chat Paper
Summary is being generated by the instructions you defined