Elaa: An Efficient Local Adversarial Attack Using Model Interpreters
INTERNATIONAL JOURNAL OF INTELLIGENT SYSTEMS(2022)
Abstract
Modern deep neural networks are highly vulnerable to adversarial examples, which attracts more and more researchers' attention to craft powerful adversarial examples. Most of these generation algorithms create global perturbations that would affect the visual quality of adversarial examples. To mitigate such drawbacks, some attacks attempt to generate local perturbations. However, existing local adversarial attacks are time-consuming and the generated adversarial examples are still distinguishable from clean images. In this paper, we propose a novel efficient local adversarial attack (ELAA) using model interpreters to generate severe local perturbations and improve the imperceptibly of the generated adversarial examples. Specifically, we take advantage of model interpretation methods to search the discriminative regions of clean images. Then, we generate local adversarial examples by adding masks to original clean images. We also propose a new optimization method to reduce the redundancy of local perturbations. Through extensive experiments, we show our ELAA can maintain a high attack ability while preserving the visual quality of clean images. Experimental results also demonstrate our local attack outperforms state-of-the-art local attack methods under various system settings.
MoreTranslated text
Key words
adversarial example, local attack, machine learning interpreter, saliency map
AI Read Science
Must-Reading Tree
Example
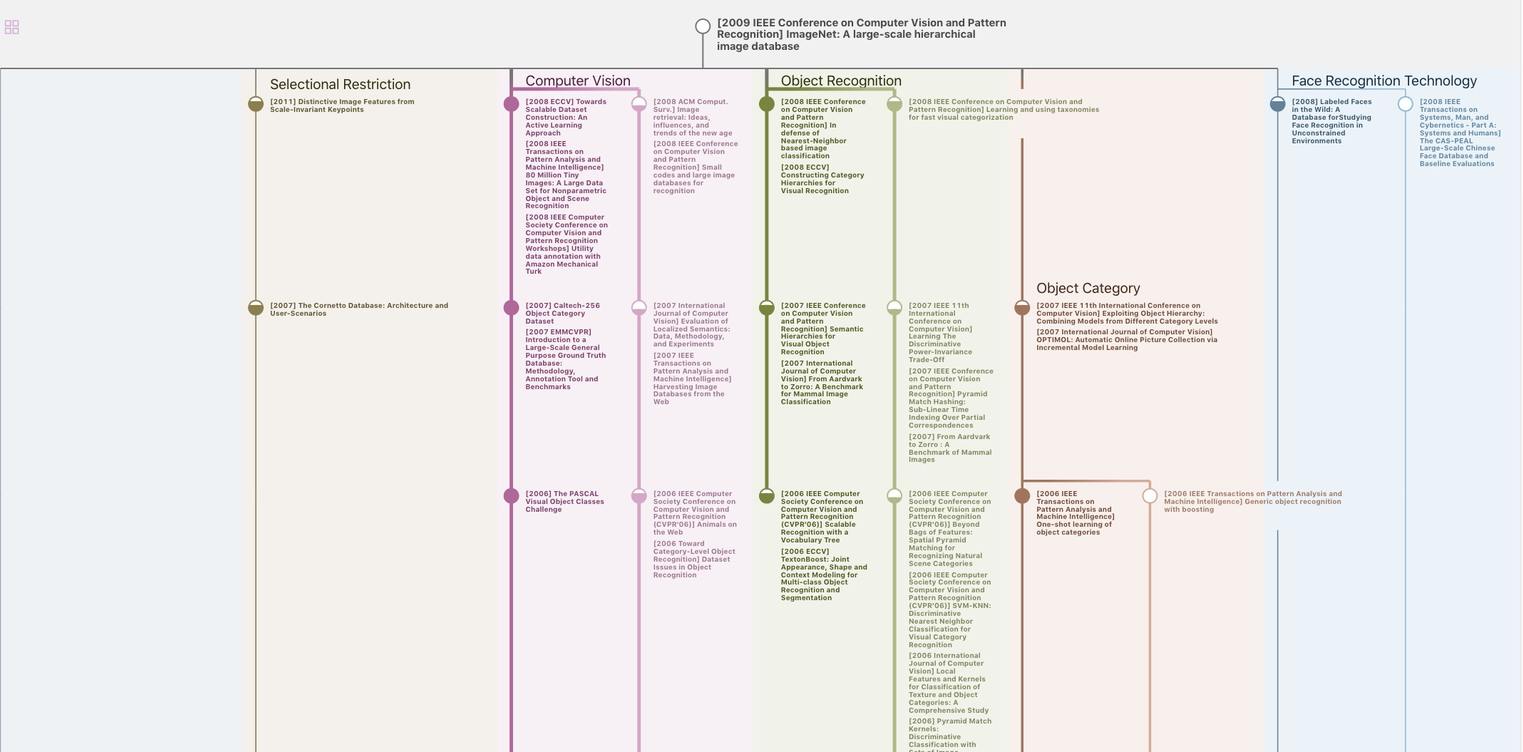
Generate MRT to find the research sequence of this paper
Chat Paper
Summary is being generated by the instructions you defined