Network Anomaly Detection Using Memory-Augmented Deep Autoencoder
IEEE ACCESS(2021)
摘要
In recent years, attacks on network environments continue to rapidly advance and are increasingly intelligent. Accordingly, it is evident that there are limitations in existing signature-based intrusion detection systems. In particular, for novel attacks such as Advanced Persistent Threat (APT), signature patterns have problems with poor generalization performance. Furthermore, in a network environment, attack samples are rarely collected compared to normal samples, creating the problem of imbalanced data. Anomaly detection using an autoencoder has been widely studied in this environment, and learning is through semi-supervised learning methods to overcome these problems. This approach is based on the assumption that reconstruction errors for samples that are not used for training will be large, but an autoencoder is often over-generalized and this assumption is often broken. In this paper, we propose a network intrusion detection method using a memory-augmented deep auto-encoder (MemAE) that can solve the over-generalization problem of autoencoders. The MemAE model is trained to reconstruct the input of an abnormal sample that is close to a normal sample, which solves the generalization problem for such abnormal samples. Experiments were conducted on the NSL-KDD, UNSW-NB15, and CICIDS 2017 datasets, and it was confirmed that the proposed method is better than other one-class models.
更多查看译文
关键词
Decoding, Anomaly detection, Network intrusion detection, Data models, Memory modules, Mathematical model, Machine learning, Network intrusion detection, autoencoder, anomaly detection, memory-augmented autoencoder
AI 理解论文
溯源树
样例
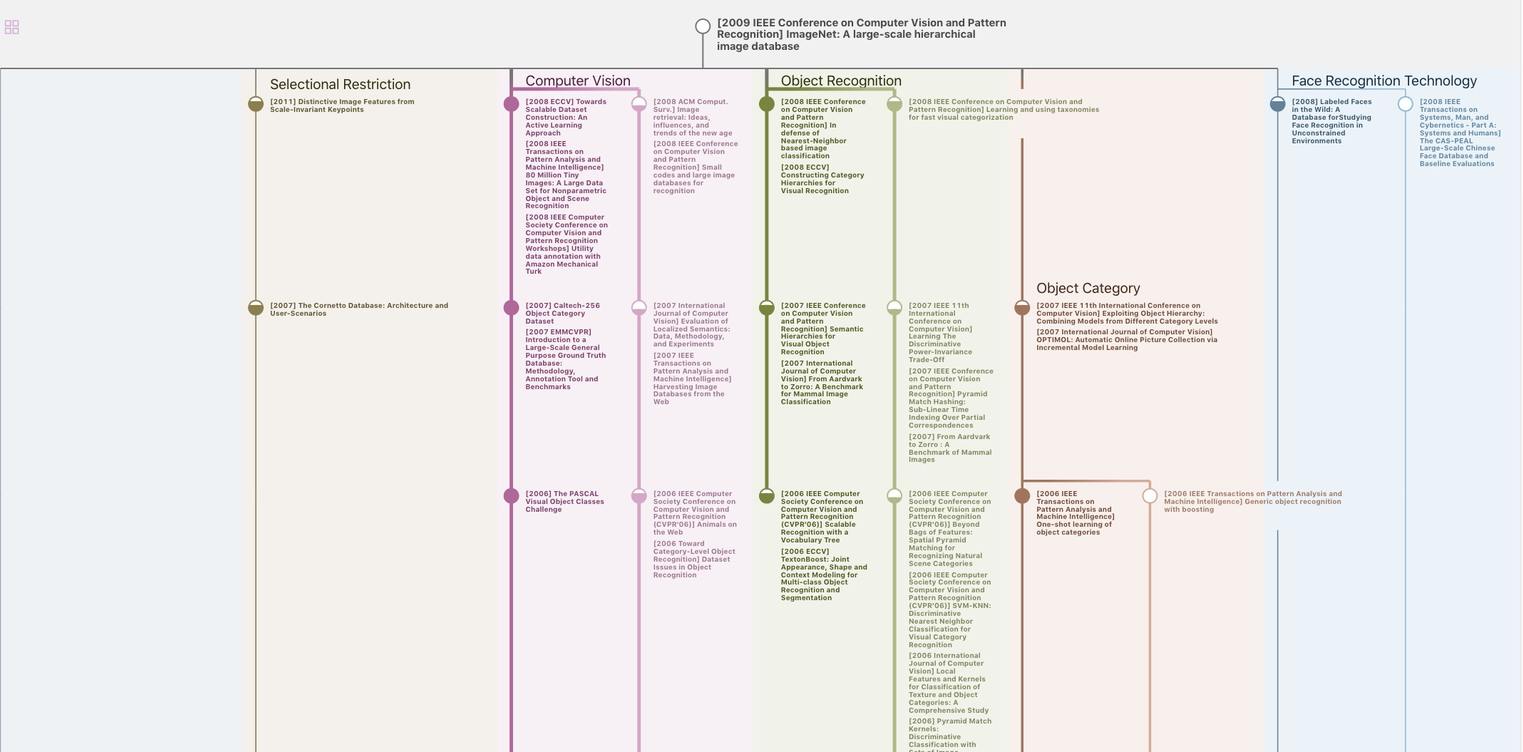
生成溯源树,研究论文发展脉络
Chat Paper
正在生成论文摘要