Novel Data-Driven Models Applied To Short-Term Electric Load Forecasting
APPLIED SCIENCES-BASEL(2021)
摘要
This work brings together and applies a large representation of the most novel forecasting techniques, with origins and applications in other fields, to the short-term electric load forecasting problem. We present a comparison study between different classic machine learning and deep learning techniques and recent methods for data-driven analysis of dynamical models (dynamic mode decomposition) and deep learning ensemble models applied to short-term load forecasting. This work explores the influence of critical parameters when performing time-series forecasting, such as rolling window length, k-step ahead forecast length, and number/nature of features used to characterize the information used as predictors. The deep learning architectures considered include 1D/2D convolutional and recurrent neural networks and their combination, Seq2seq with and without attention mechanisms, and recent ensemble models based on gradient boosting principles. Three groups of models stand out from the rest according to the forecast scenario: (a) deep learning ensemble models for average results, (b) simple linear regression and Seq2seq models for very short-term forecasts, and (c) combinations of convolutional/recurrent models and deep learning ensemble models for longer-term forecasts.
更多查看译文
关键词
short-term electric load forecasting, deep learning, machine learning, dynamic mode decomposition, deep learning ensemble model
AI 理解论文
溯源树
样例
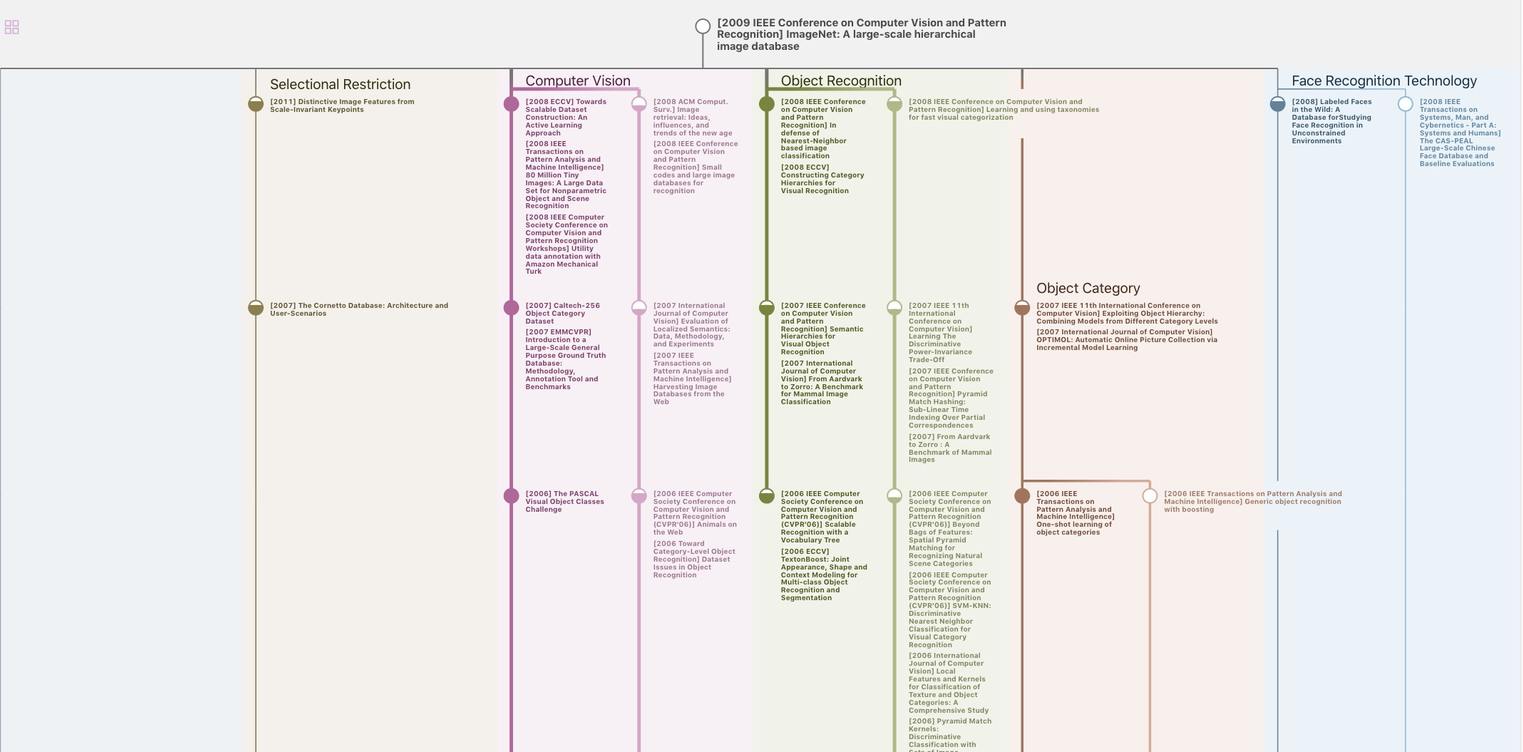
生成溯源树,研究论文发展脉络
Chat Paper
正在生成论文摘要