VirusBERTHP: Improved Virus Host Prediction Via Attention-based Pre-trained Model Using Viral Genomic Sequences.
2023 IEEE International Conference on Bioinformatics and Biomedicine (BIBM)(2023)
摘要
Virus has become the most prominent cause of infectious diseases which greately threaten human health. Determining whether a viral genome can possess human host infectivity would be of great value to epidemic prevention. However, due to the highly diversified and unstructured nature of virus genomes, current bioinformatic and machine learning methods for prediction virus host infectivities are rather limited in performance. In this paper we propose an accurate virus human host infectivity prediction tool, VirusBERTHP, using an attention-based pretraining mechanism following the well-known BERT architecture, which is capable of predicting the human infectivity of a novel virus species whose genome is not in the training database. We develop a BERT-based representation learning scheme, VirusBERT, to efficiently extract the complex feature among versatile virus sequences, which show greate seperability in the feature space. We created a large curated database containing 2,948,656 unlabelled virus sequences to efficiently pre-train the VirusBERT model. Then, the VirusBERTHP model is trained with a relatively smaller set of labelled sequences corresponding to specific tasks, using a full-connected deep neural network. We adopted the model on four published virus-host classification datasets and showed that our model outperforms previous state-of-the-art methods in prediction performance. On three datasets with open-view setting where no restriction is imposed on the taxonomy of the input virus sequences, our model achieved more than 99% accuracy in predicting human host infectivity, justifying the efficiency of our method. In addition to accuracy boost, our model is adaptive to various virus sequence prediction task by seperating the pretraining and supervised learning phases. In addition, the model is adaptable to a wide range of sequence lengths from 250bps to 10k bps, expanding the application field of the model. Source code and data of our paper is available at https://github.com/wyzwyzwyz/virusBert/.
更多查看译文
关键词
Virus host prediction,gene sequence,deep learning,pre-training,bert,attention mechanism,representation learning
AI 理解论文
溯源树
样例
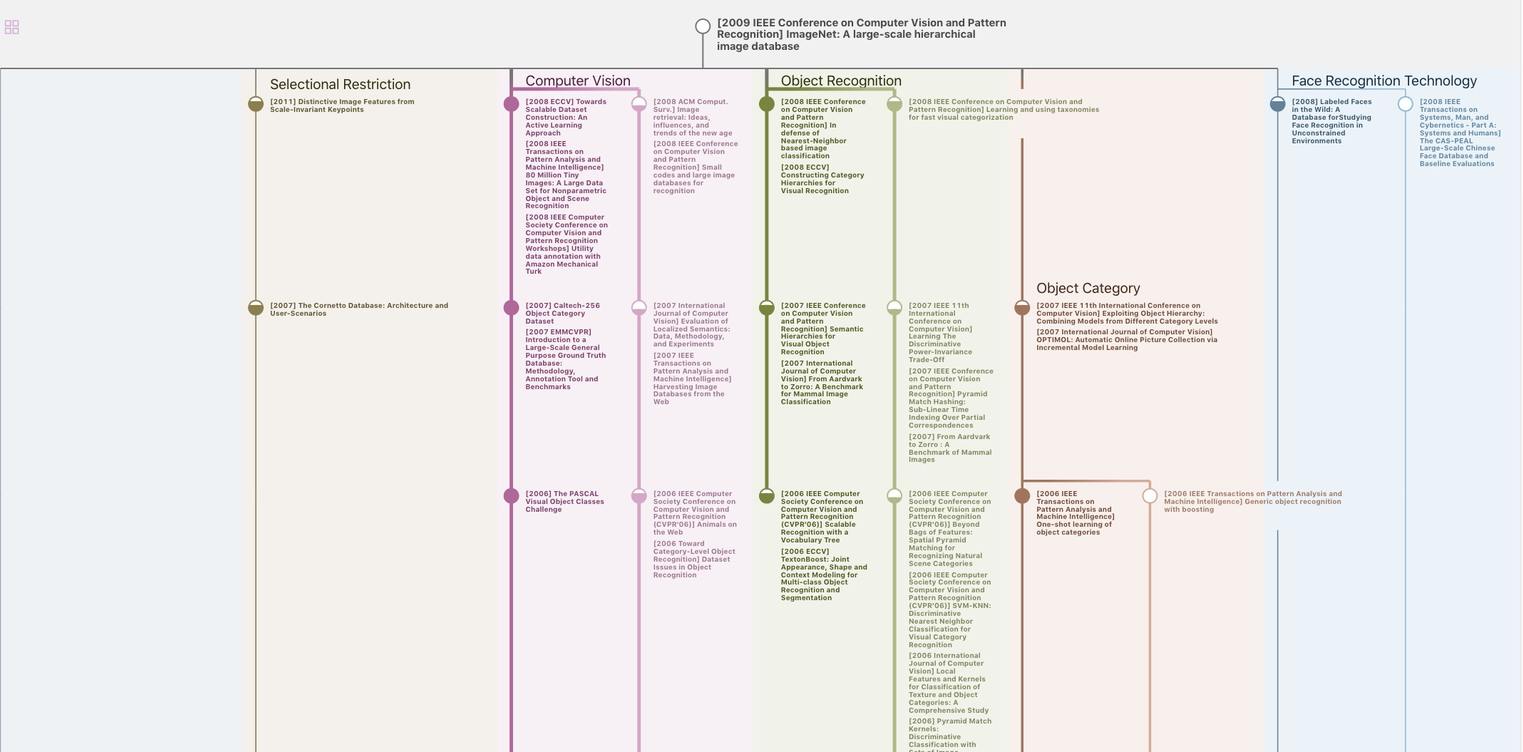
生成溯源树,研究论文发展脉络
Chat Paper
正在生成论文摘要