Evaluating Fish Feeding Intensity In Aquaculture With Convolutional Neural Networks
AQUACULTURAL ENGINEERING(2021)
摘要
This paper presents a novel method to evaluate fish feeding intensity for aquaculture fish farming. Determining the level of fish appetite helps optimize fish production and design more efficient aquaculture smart feeding systems. Given an aquaculture surveillance video, our goal is to improve fish feeding intensity evaluation by proposing a two-stage approach: an optical flow neural network is first applied to generate optical flow frames, which are then inputted to a 3D convolution neural network (3D CNN) for fish feeding intensity evaluation. Using an aerial drone, we capture RGB water surface images with significant optical flows from an aquaculture site during the fish feeding activity. The captured images are inputs to our deep optical flow neural network, consisting of the leading neural network layers for video interpolation and the last layer for optical flow regression. Our optical flow detection model calculates the displacement vector of each pixel across two consecutive frames. To construct the training dataset of our CNNs and verify the effectiveness of our proposed approach, we manually annotated the level of fish feeding intensity for each training image frame. In this paper, the fish feeding intensity is categorized into four, i.e., 'none,' 'weak,' 'medium' and 'strong.' We compared our method with other state-of-the-art fish feeding intensity evaluations. Our proposed method reached up to 95 % accuracy, which outperforms the existing systems that use CNNs to evaluate the fish feeding intensity.
更多查看译文
关键词
Fish feeding intensity, Convolutional neural networks, Video interpolation, Optical flow, Aquaculture
AI 理解论文
溯源树
样例
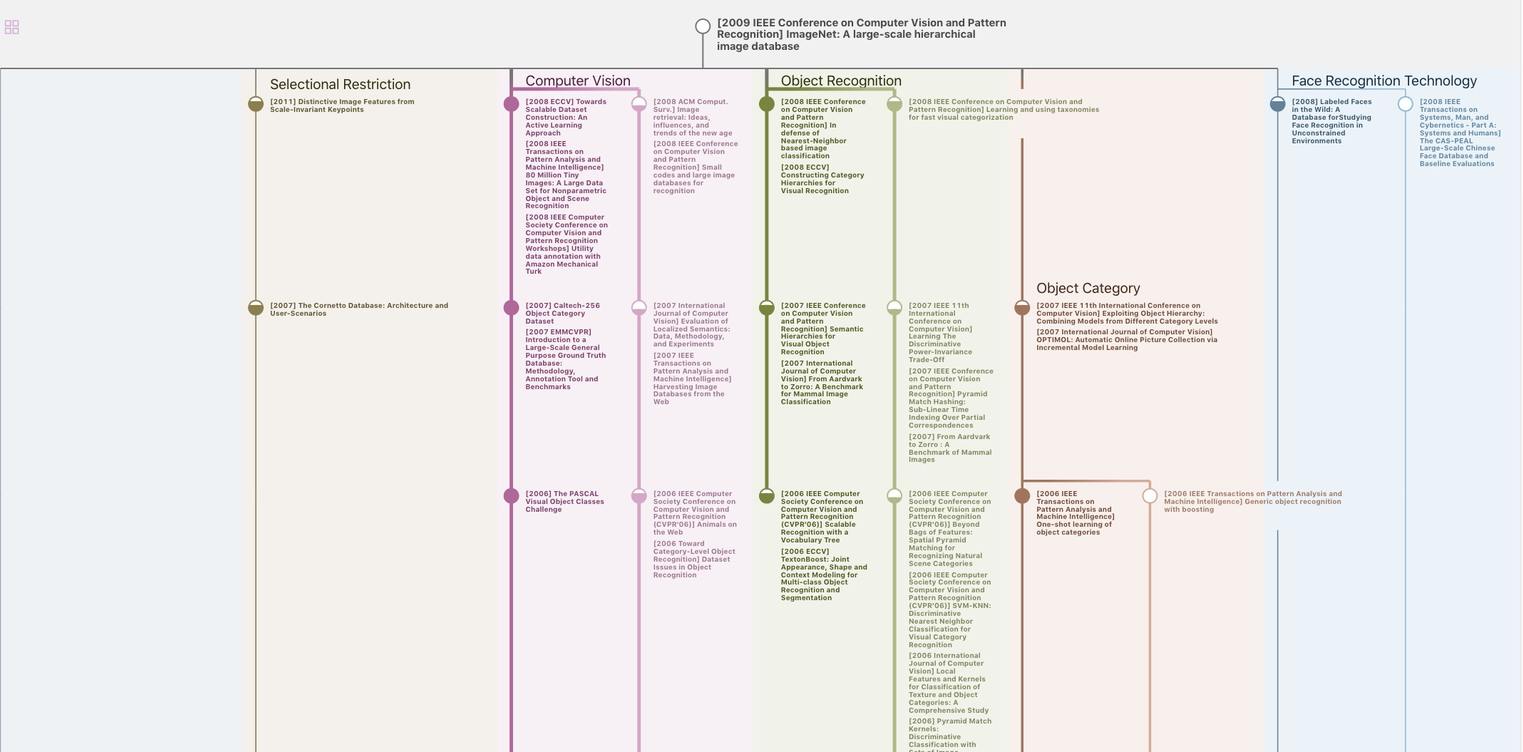
生成溯源树,研究论文发展脉络
Chat Paper
正在生成论文摘要