Deepfakenet, An Efficient Deepfake Detection Method
INTERNATIONAL JOURNAL OF ADVANCED COMPUTER SCIENCE AND APPLICATIONS(2021)
Abstract
Different CNNs models do not perform well in deepfake detection in cross datasets. This paper proposes a deepfake detection model called DeepfakeNet, which consists of 20 network layers. It refers to the stacking idea of ResNet and the split-transform-merge idea of Inception to design the network block structure, That is, the block structure of ResNeXt. The study uses some data of FaceForensics++, Kaggle and TIMIT datasets, and data enhancement technology is used to expand the datasets for training and testing models. The experimental results show that, compared with the current mainstream models including VGG19, ResNet101, ResNeXt50, XceptionNet and GoogleNet, in the same dataset and preset parameters, the proposed detection model not only has higher accuracy and lower error rate in cross dataset detection, but also has a significant improvement in performance.
MoreTranslated text
Key words
DeepfakeNet, deepfake detection, data enhancement, CNNs, cross dataset
AI Read Science
Must-Reading Tree
Example
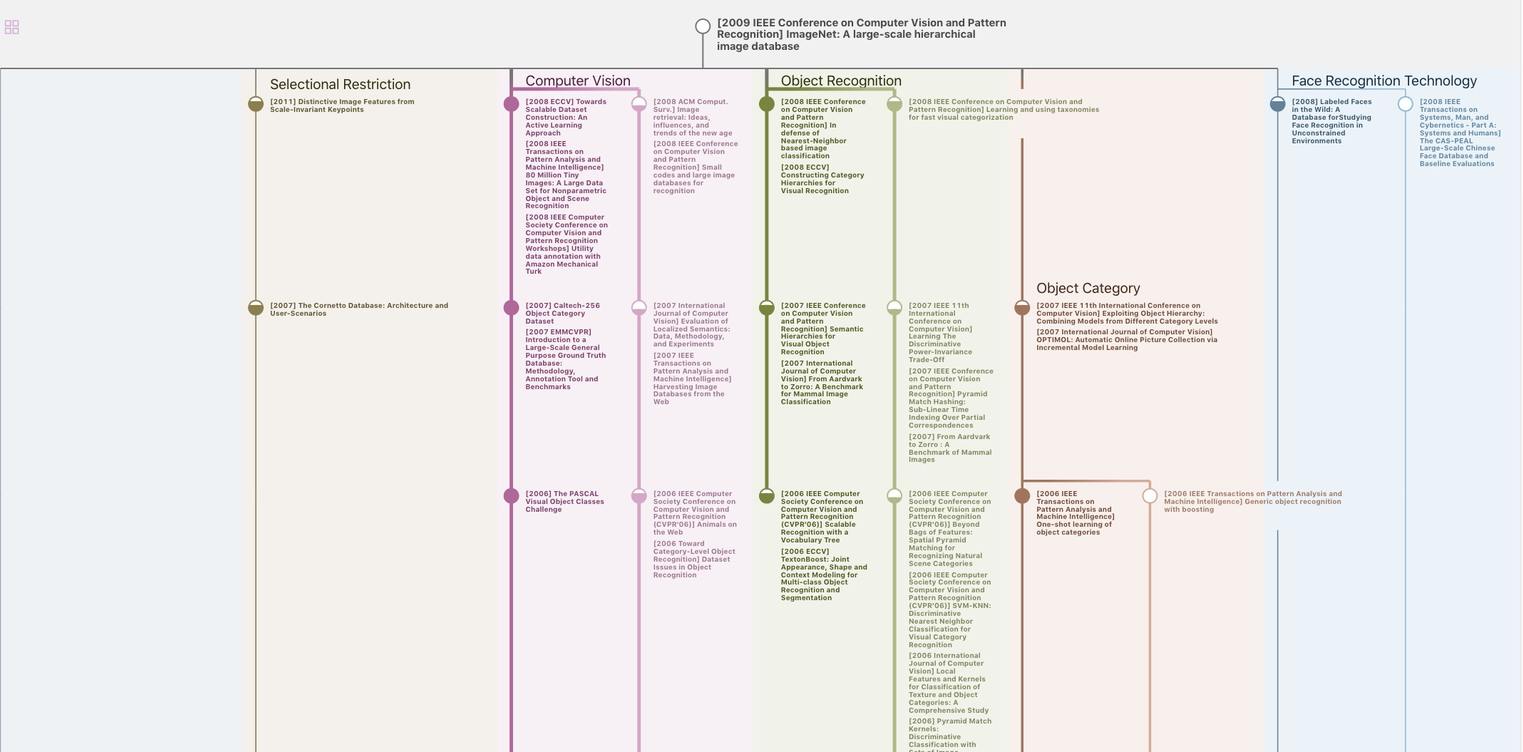
Generate MRT to find the research sequence of this paper
Chat Paper
Summary is being generated by the instructions you defined