Novel insights for streamflow forecasting based on deep learning models combined the evolutionary optimization algorithm
PHYSICAL GEOGRAPHY(2023)
摘要
Various hybrid approaches combined the different deep learning and machine learning models with evolutionary optimization algorithms and have improved the accuracy of streamflow forecasting problem. In this article, three deep learning models were investigated for streamflow forecasting with various lag times at both stations (i.e. Sidi Aich and Ponteba Defluent), Algeria. Also, a machine learning [i.e. feedforward neural network (FFNN)] model was implemented to compare the forecasting accuracy of deep learning models. The particle swarm optimization algorithm was combined to determine the hyperparameters (i.e. model structure) automatically based on adaptive moment estimation algorithm. The addressed two-stage hybrid models were assessed and evaluated by root mean square error (RMSE), signal-to-noise ratio (SNR), and Nash-Sutcliffe efficiency (NSE) statistical indices. Evaluating all models explained that the GRU II two-stage hybrid model (RMSE = 35.241 m(3)/s, SNR = 0.5159, and NSE = 0.7337 at Sidi Aich and RMSE = 11.074 m(3)/s, SNR = 0.3600, and NSE = 0.8703 at Ponteba Defluent) was found to produce more accurate results compared to the Elman recurrent neural network, long short-term memory, and FFNN two-stage hybrid models during testing phase for forecasting streamflow.
更多查看译文
关键词
Streamflow forecasting,feedforward neural network,Elman recurrent neural network,long short-term memory,gated recurrent unit,particle swarm optimization
AI 理解论文
溯源树
样例
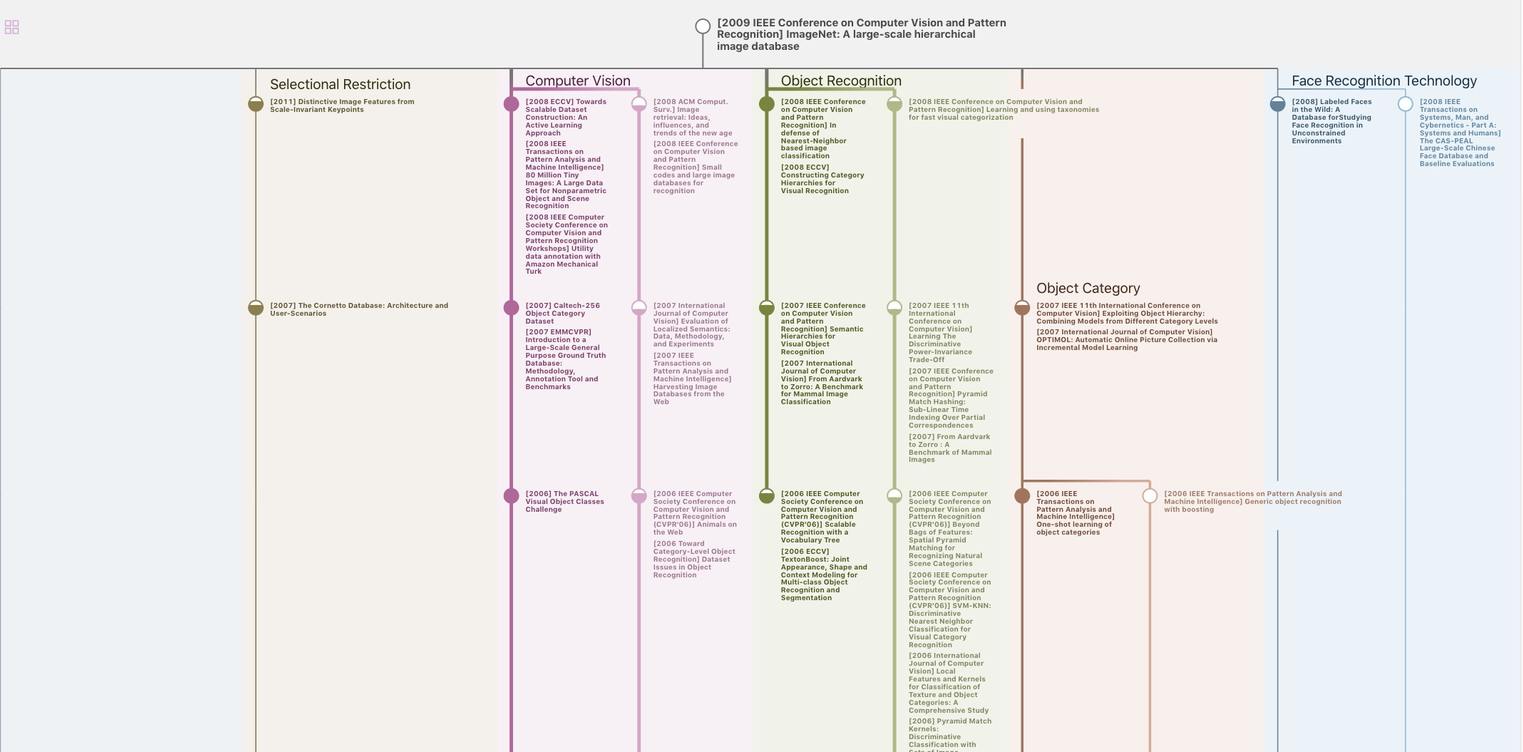
生成溯源树,研究论文发展脉络
Chat Paper
正在生成论文摘要