Online dependence clustering of multivariate streaming data using one-class SVMs
INTERNATIONAL JOURNAL OF INTELLIGENT SYSTEMS(2022)
摘要
Online clustering of multivariate streaming data has attracted considerable interest in recent years due to the abundance of data sources. Numerous studies in this field have been performed, but they usually suffer from the practical problems associated with discovering arbitrary-shaped clusters, specifying major parameters in advance, and detecting aberrant observations. Addressing these issues is important for online-clustering tasks, where data arrive in continuous streams and group behaviors change simultaneously. In this paper, we propose a kernel-based online dependence clustering, namely, KODC, that not only estimates the cluster membership using one-class support vector machines (OC-SVMs), but also detects outliers distant from the identified clusters by aggregating OC-SVM decisions in a realtime basis. At the base level, we use a new measure of connective dependence that forms the graph connected via modified Markovian transitions to enable large-scale clustering. The proposed framework introduces the coherence threshold to extract data points, which can represent a cluster to which they belong, thus controlling the computational complexity without degrading the clustering performance. To track the pattern evolution over time, KODC also updates the classifier configuration maximizing the total group connective dependence. We evaluate this framework on both several synthetic and real-world data sets involving multivariate streaming data, and compare it experimentally with other popular online-clustering methods in terms of four evaluation metrics. The results show that our framework effectively identifies the clusters and outliers, especially in various shaped data subject to change over time, without requiring any prior knowledge of the data.
更多查看译文
关键词
dependence clustering, one-class support vector machine, online data analysis, outlier detection, unsupervised learning
AI 理解论文
溯源树
样例
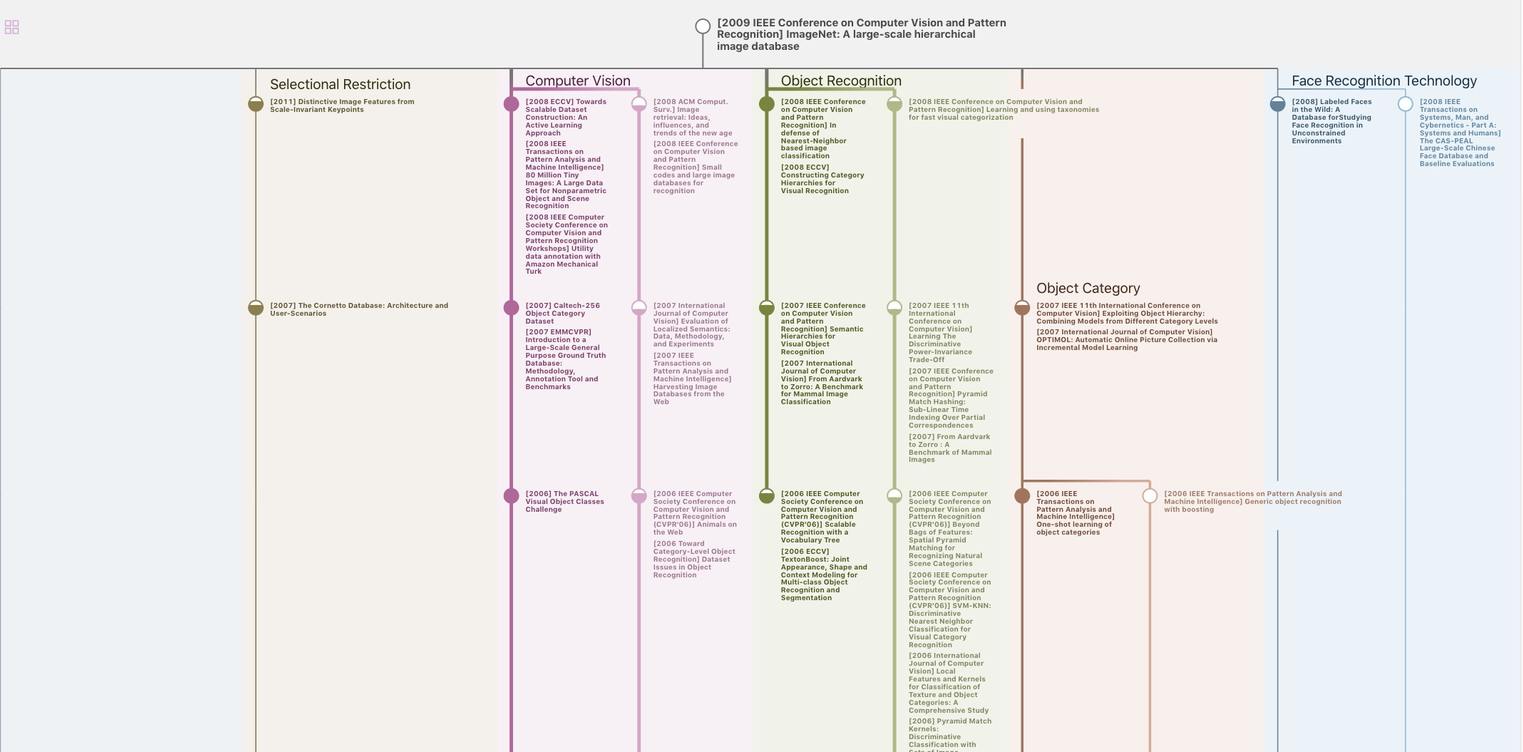
生成溯源树,研究论文发展脉络
Chat Paper
正在生成论文摘要