Machine Learning Multi-Modality Fusion Approaches Outperform Single-Modality & Traditional Approaches
2021 IEEE AEROSPACE CONFERENCE (AEROCONF 2021)(2021)
摘要
This paper presents results from two machine learning components within the upstream multi-modal fusion and decision-making framework introduced previously (Garagic et al., 2018 IEEE Aerospace Conference, doi: 10.1109/AERO.2018.8396737, Garagic et al., 2019 IEEE Aerospace Conference, doi: 10.1109/AERO.2019.8742221). The first combines Bayesian change-point motion-based detection to discover new targets with online transfer learning over Convolutional Neural Networks (CNNs) to learn models of previously seen targets and adapt those models as target appearance changes. CNN detection overcomes motion-based detection problems that arise when targets stop or operate in close proximity to each other. Motion-based detection overcomes the CNN problem of initializing target appearance and adapting to target appearance changes. This novel combination of motion and CNN detectors obviates the need for prior knowledge about targets to stimulate target detection. This detection approach improves performance over that achieved without an appearance-based detector on challenging data. A closed-loop between the tracking component and the detector component modules drives detector model adaptation. The second component combines multi-sensor, multi-modality features from upstream detection and tracking components to learn dynamic compact probabilistic representations of targets as manifolds in a joint space over all inputs. This Multi-Modal Deep Belief Network (MMDBN) approach incorporates supervised deep autoencoders and unsupervised Dirichlet process mixture models. Supervision within 1VILVIDBN arises from consistent associations between sensor and modality inputs for a target of interest. 1VILVIDBN thus learns and optimally leverages inter-sensor, inter-modality features of targets. This approach obviates the need for explicit, hand-crafted intermodal features because it implicitly learns both inter-modal features and a likelihood model to evaluate those features. This fusion approach improves tracking performance over a traditional fusion approach on challenging data. This overall approach offers multiple benefits in addition to outperforming single-modality and traditional fusion approaches. It is feature agnostic by accommodating any data source that provides a feature set of pre-determined dimensionality for each target at each time step. This paper describes novel methods for enhancing motion-based tracking by adding an appearance-based sidecar that provides to improve DTC performance in difficult conditions. It also showed the benefits of a feature-level fusion approach in performing DTC on several challenging multi-modality, multi-platforms AFRL ESCAPE data scenarios.
更多查看译文
关键词
feature-level fusion approach,upstream multimodal fusion,2018 IEEE Aerospace Conference,2019 IEEE Aerospace Conference,combines Bayesian change-point motion-based detection,target appearance changes,CNN detection,motion-based detection problems,CNN problem,CNN detectors,target detection,appearance-based sidecar,motion-based tracking,challenging data,traditional fusion approach,likelihood model,inter-modal features,explicit hand-crafted intermodal features,inter-modality features,modality inputs,unsupervised Dirichlet process mixture models,MMDBN,tracking components,multimodal deep belief network approach,machine learning multimodality fusion approaches,upstream detection,multimodality features,multisensor,detector component modules drives detector model adaptation,tracking component,appearance-based detector
AI 理解论文
溯源树
样例
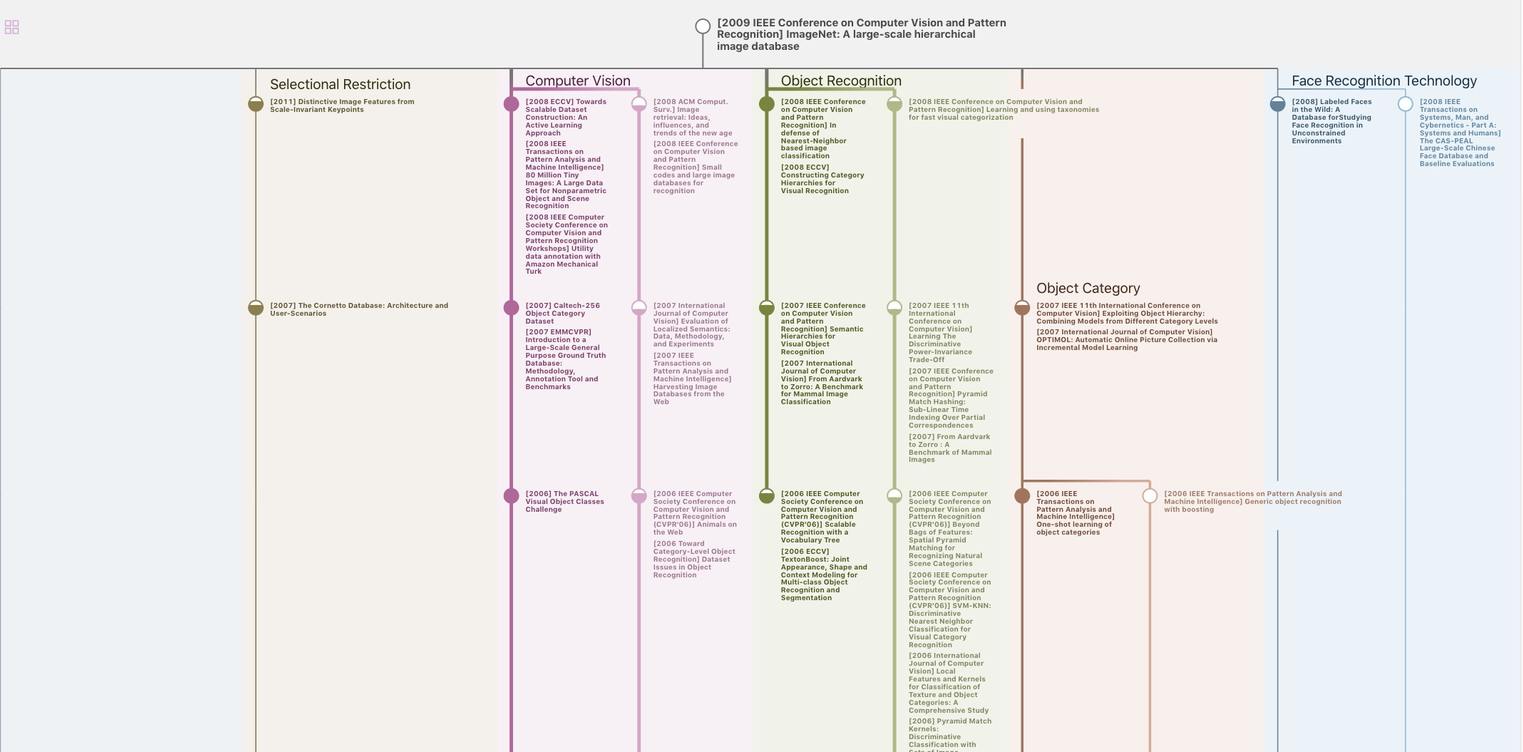
生成溯源树,研究论文发展脉络
Chat Paper
正在生成论文摘要