Machine Learning for Intrusion Detection: Design and Implementation of an IDS Based on Artificial Neural Network
JOURNAL OF INFORMATION ASSURANCE AND SECURITY(2021)
摘要
Securing the network from intrusions becomes a more challenging task to conduct for system administrators, and the need for a more powerful and efficient intrusion detection system emerges with the continuous development of cyber-attacks exploring various methods and techniques. We propose the usage of artificial intelligence to build a sophisticated Network Intrusion Detection System able to be trained/self-trained to detect malicious network traffic. The aim of this paper is to present a new IDS model based on machine learning approach to detect malicious traffic and protect the network from cyber-attacks. The usage of machine learning will allow better accuracy in detection and faster response time. To implement and measure the performance of our model, we used NSL-KDD dataset, after the preprocessing phase, (cleaning, normalization …), we used the most relevant attributes for the classification process based on CfsSubsetEval technique with BestFirst approach as an attribute selection algorithm for removing the redundant attributes to allow the usage of the most pertinent attributes of the dataset. Based on the comparative evaluation of three algorithms (K-means, AdaBoost and Multilayer Perceptron), the experimental results show that the MLP algorithm provides a high detection rate and reduces false alarm rate. Finally, a set of principles is concluded, which will set path for future research for implementing an efficient and performant IDS. To help researchers in the selection of IDS, several recommendations are provided with future directions for this research.
更多查看译文
关键词
Intrusion detection system,Machine learning,NSL-KDD,Multi-layer perceptron
AI 理解论文
溯源树
样例
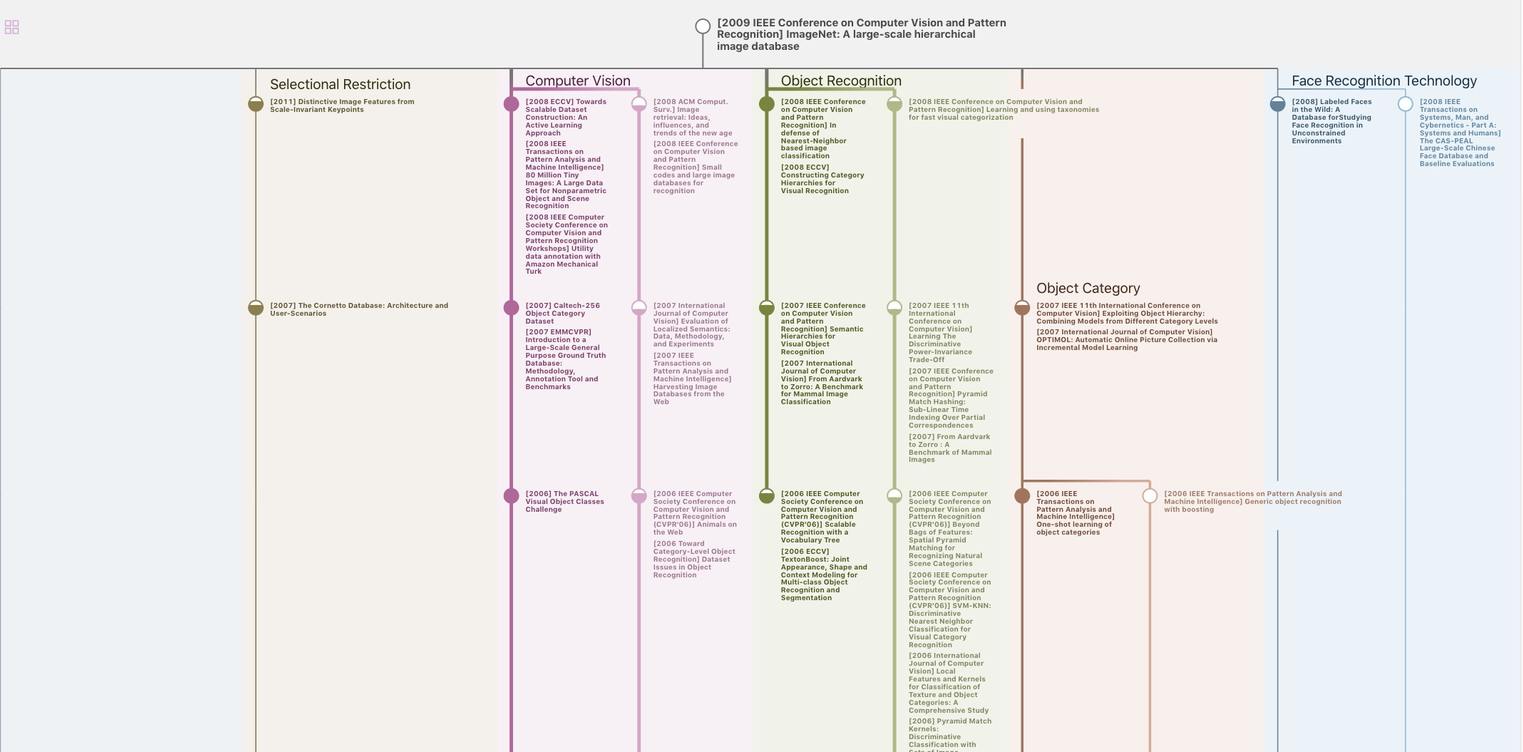
生成溯源树,研究论文发展脉络
Chat Paper
正在生成论文摘要